DeepSeek’s Impact on Enterprise RAG Strategy: The Hybrid Model Approach
DeepSeek is revolutionizing enterprise RAG strategies with a hybrid model that enhances retrieval-augmented generation (RAG) for improved accuracy, efficiency, and scalability. Discover how this approach optimizes data retrieval and AI-driven insights for businesses.
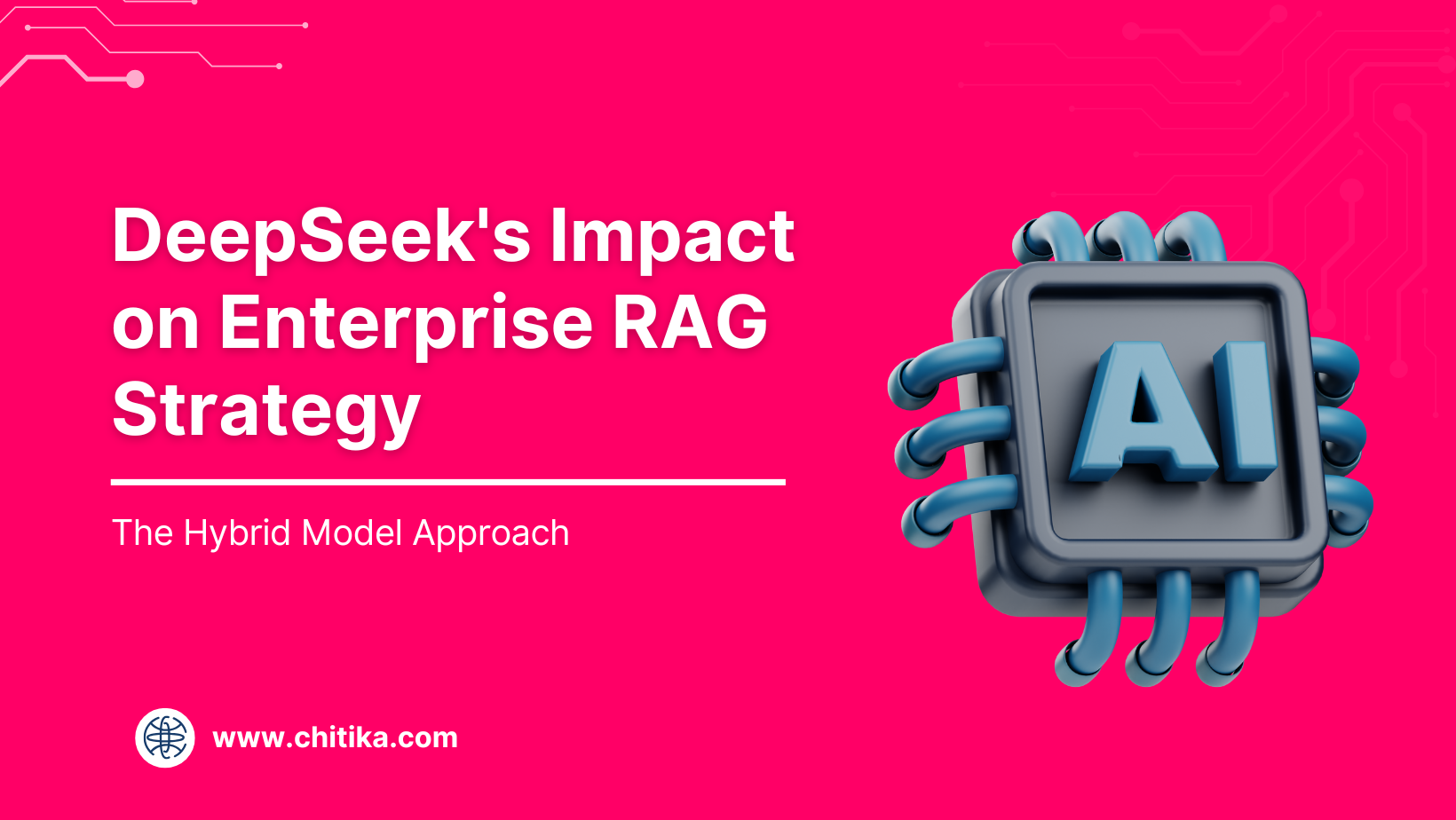
In enterprise AI, there's a common misconception that we must choose between the flexibility of cloud-based solutions and the security of on-premise systems.
Well, here’s a spoiler alert—we don’t need to.
In fact, hybrid RAG models, powered by DeepSeek, prove that enterprises can have the best of both worlds.
Here’s the kicker: while most organizations are still grappling with the trade-offs of traditional RAG setups, those adopting hybrid models power are slashing costs and scaling faster than their competitors.
Why now? Because the stakes have never been higher.
With data privacy regulations tightening and AI workloads ballooning, the pressure to innovate without compromising security is immense.
But here’s the real question: How do you strike the perfect balance between cloud agility and on-prem control? This article explores the hybrid model approach and reveals how DeepSeek is rewriting the rules of enterprise RAG strategy.
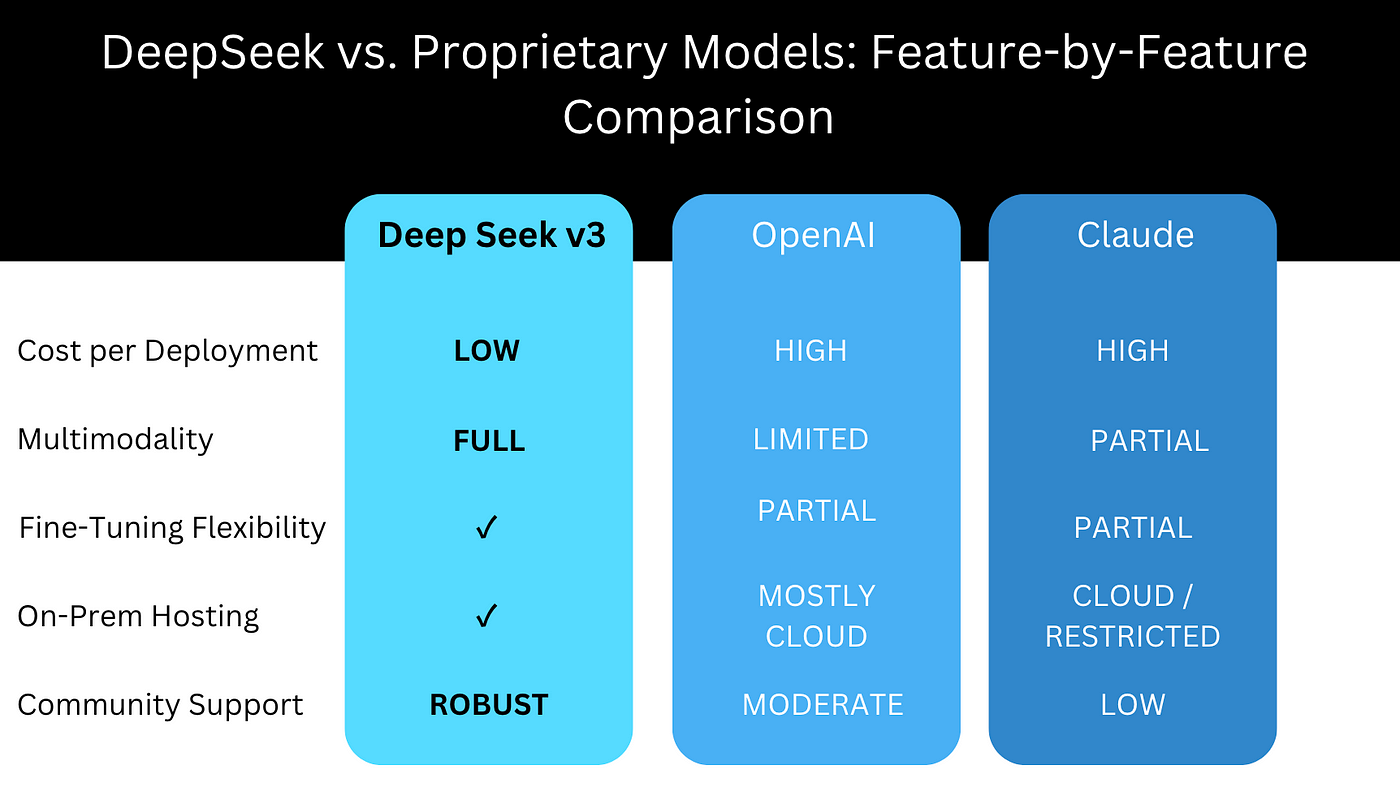
Introduction to DeepSeek and Its Relevance
DeepSeek R1 is redefining enterprise RAG strategies by addressing a critical pain point: the trade-off between cost efficiency and high-performance AI.
Unlike proprietary models, DeepSeek R1’s open-source framework offers enterprises a commercially viable solution with advanced reasoning capabilities at a fraction of the cost.
This makes it particularly relevant for organizations navigating tight budgets without compromising on innovation.
Here’s the kicker: DeepSeek’s hybrid compatibility allows seamless integration of on-premise and cloud-based systems.
For instance, a healthcare provider can use on-premise setups to safeguard sensitive patient data while leveraging cloud AI for predictive analytics in treatment planning. This dual approach ensures compliance without sacrificing scalability.
Fundamentals of Retrieval-Augmented Generation (RAG) in Enterprises
Consider RAG as the brain behind enterprise AI—combining retrieval systems with generative models to deliver precise, context-aware answers.
But here’s the twist: its success hinges on the quality of the knowledge base, not just the model.
For example, a healthcare provider using RAG might need to answer, “What are the side effects of Drug X?” If the knowledge base is fragmented or outdated, even the best AI will fail.
This is where DeepSeek shines. It enables seamless integration of on-premise and cloud systems, ensuring data is both accessible and secure.
The takeaway? RAG thrives on balance. Like a symphony, it requires harmony between data, infrastructure, and AI to truly transform enterprise operations.
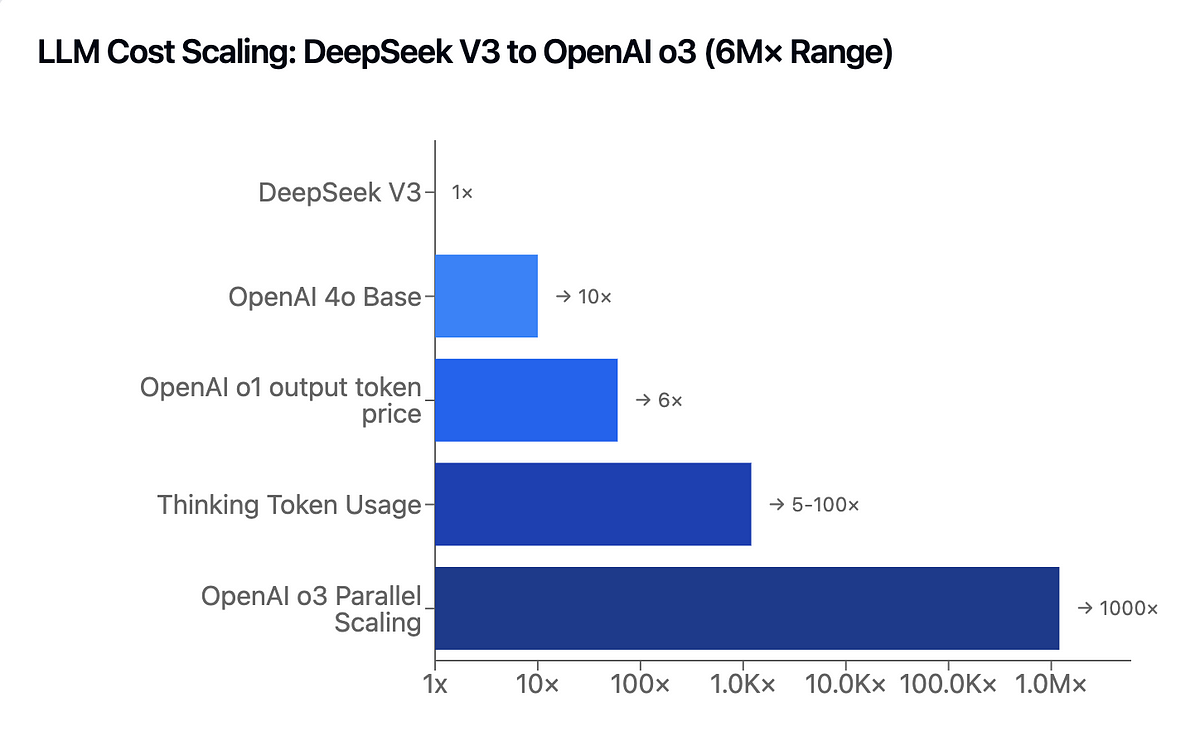
DeepSeek’s Technology and Core Capabilities
At the heart of DeepSeek R1 is its chain-of-thought reasoning, a feature that sets it apart from conventional RAG systems.
This approach doesn’t just retrieve and generate—it explains. DeepSeek allows enterprises to debug, refine, and trust the system’s outputs by making its reasoning process visible.
Think of it as having a GPS that shows the route and explains why it chose that path.
Another standout capability? It's adaptive retrieval tuning. DeepSeek learns from real-time feedback, optimizing results dynamically. This is a game-changer for industries like e-commerce, where user preferences shift constantly.
Differentiators of DeepSeek in the Market
DeepSeek R1’s open-source accessibility is a game-changer.
Unlike proprietary models, it allows enterprises to customize workflows without being locked into vendor ecosystems. This flexibility is critical for industries like finance, where compliance demands unique, tailored solutions.
Cost-effectiveness is another overlooked factor. DeepSeek’s architecture reduces computational overhead, making it viable for small-to-medium enterprises. This levels the playing field, enabling broader adoption of advanced AI.
The Hybrid Model Approach in RAG
Think of hybrid RAG as the Swiss Army knife of enterprise AI—versatile, adaptable, and built for complex challenges. It combines the security of on-premise systems with the scalability of cloud solutions, giving enterprises the best of both worlds.
A common misconception is that hybrid models are “just a compromise.” In reality, they’re a strategic advantage.
By blending retrieval methods—like semantic search for unstructured data and keyword search for structured data—DeepSeek ensures precision without sacrificing speed.
Think of it like a relay race. Each system (on-prem or cloud) plays its part, passing the baton seamlessly for a winning finish.
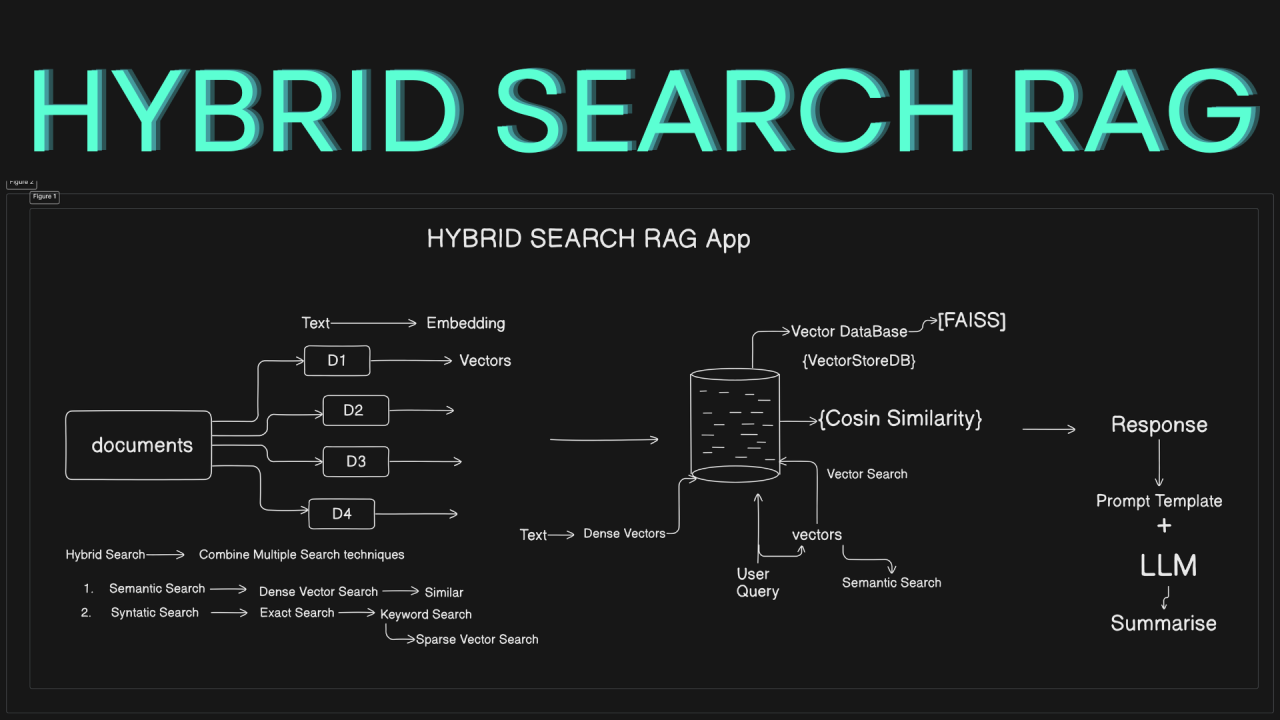
Integrating DeepSeek with the Hybrid Model Approach
DeepSeek R1 can act as a glue that binds the hybrid RAG model together.
It doesn’t just sit in the system—it actively bridges the gap between cloud scalability and on-premise security.
Here’s how: its adaptive retrieval tuning ensures that sensitive data stays local, while less critical tasks leverage the cloud for speed and scale.
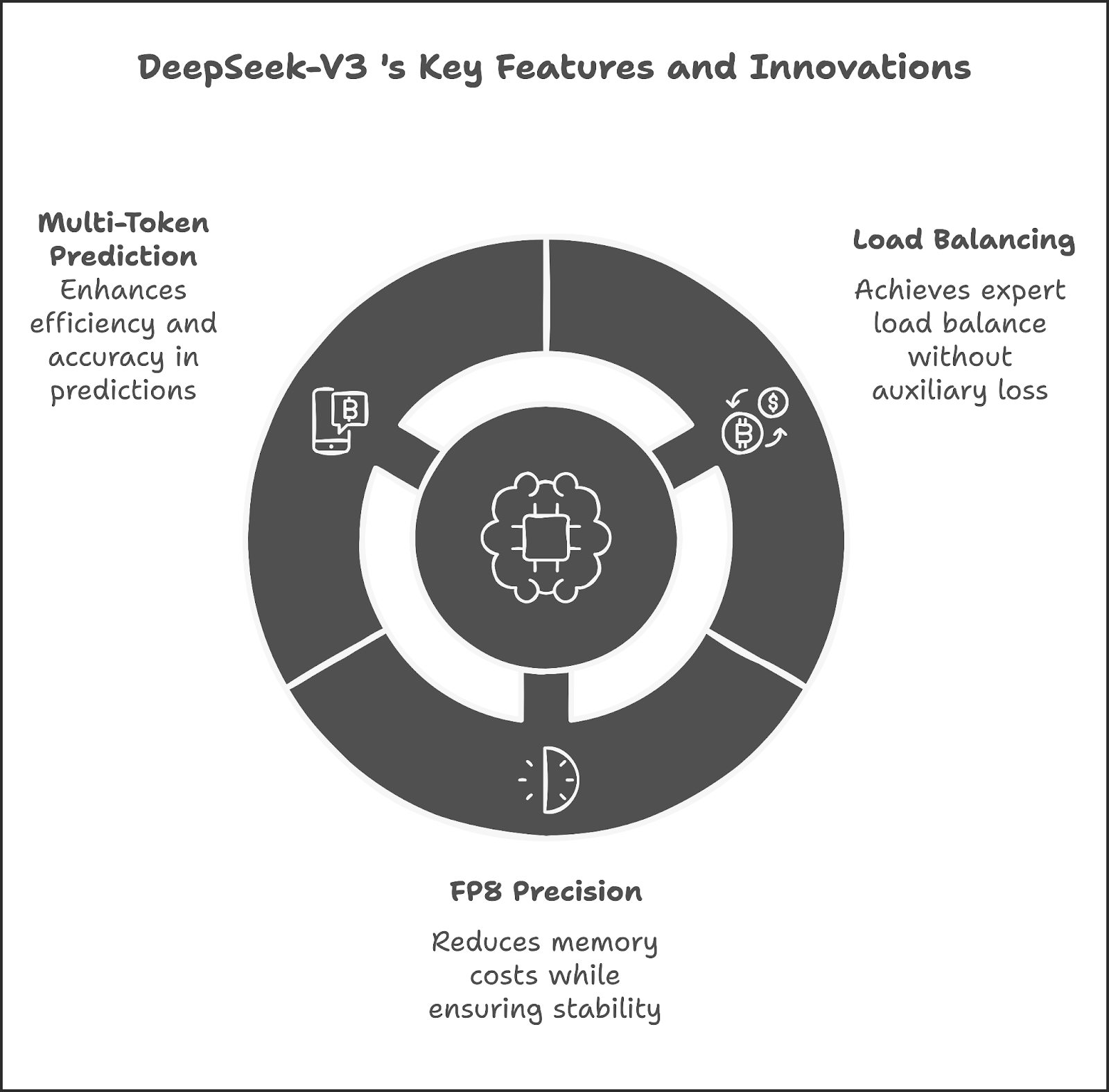
How DeepSeek Implements Hybrid Models
DeepSeek R1’s secret weapon in hybrid models is its dynamic workload allocation.
It uses metadata tagging to classify data by sensitivity and urgency, ensuring that critical information stays on-premise while less sensitive tasks are offloaded to the cloud.
This isn’t just efficient—it’s strategic. Sensitive healthcare records, for instance, can remain secure on local servers, while cloud resources analyze anonymized patient trends for predictive care.
Enhancing Retrieval with Deep Neural Networks
Deep neural networks (DNNs) are the backbone of DeepSeek’s retrieval precision, but here’s the twist: it’s not just about raw power—it’s about contextual intelligence.
By leveraging transformer-based architectures, DeepSeek doesn’t just retrieve data; it understands the why behind the query. This allows it to surface results that align with user intent, not just keywords.
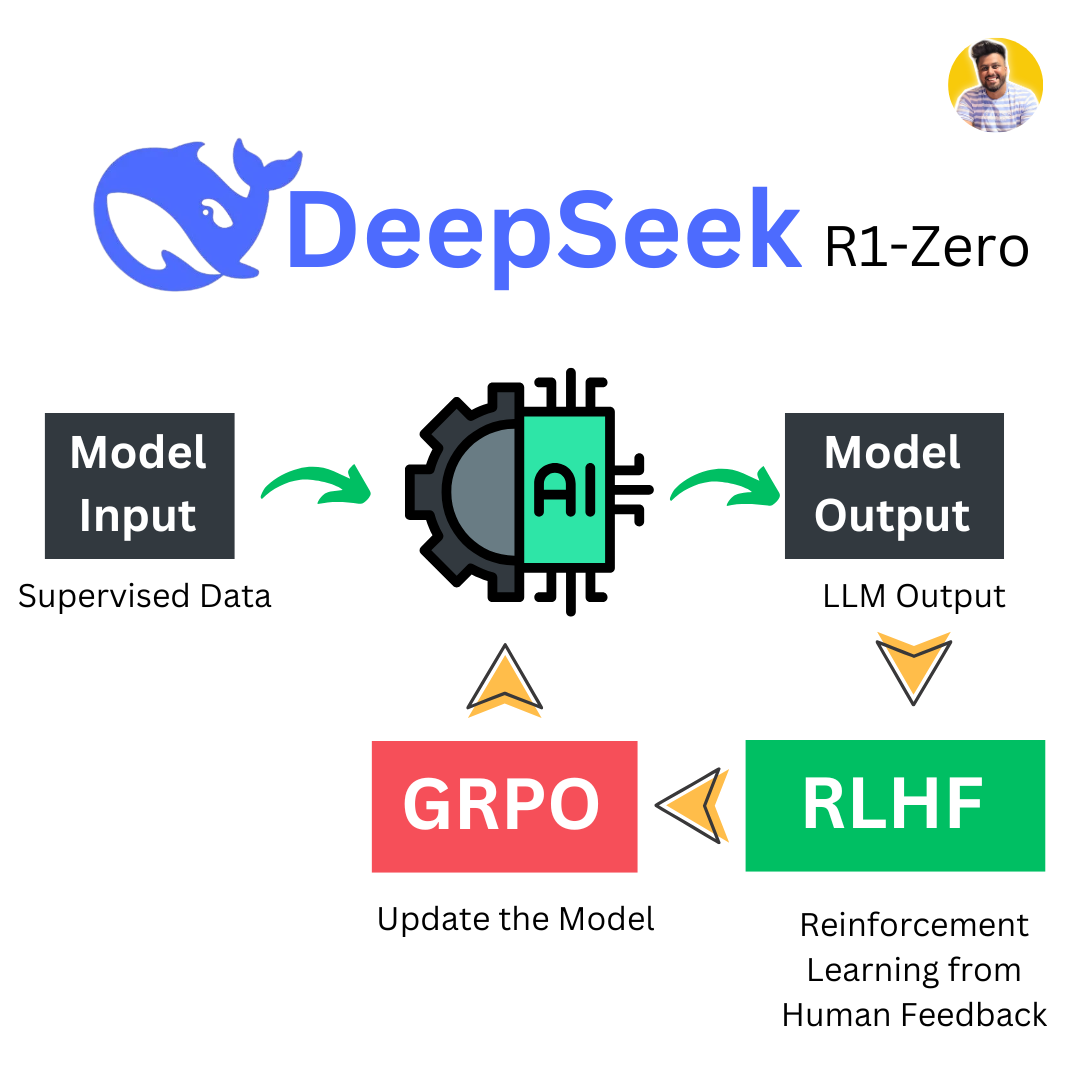
Scalability and Performance Benefits
Scalability isn’t just about handling more data—it’s about doing it smarter.
DeepSeek R1’s hybrid model excels here by dynamically allocating workloads between on-premise systems and the cloud.
Sensitive data stays secure on-premise, while computationally intensive tasks leverage cloud resources. This balance ensures enterprises can scale without compromising compliance or performance.
Here’s where it gets interesting: contextual embeddings. These allow DeepSeek to process data relationships at scale, making retrieval faster and more accurate as datasets grow.
ROI Analysis and Business Implications
Regarding ROI, DeepSeek R1 flips the script on traditional RAG investments. Instead of pouring resources into rigid, cloud-only systems, enterprises can now leverage a hybrid model that pays for itself.
Here’s how: dynamic workload allocation ensures that high-cost cloud resources are only used when absolutely necessary, while sensitive data stays on-premise, cutting compliance-related expenses.
The secret sauce is adaptive retrieval tuning. By continuously refining results based on real-time feedback, DeepSeek ensures that every dollar spent on AI delivers measurable outcomes.
Implementation Best Practices
When implementing DeepSeek R1 in a hybrid RAG model, think of it like orchestrating a symphony.
Each component—on-premise systems, cloud resources, and data orchestration—must play in harmony to deliver peak performance.
Start by categorizing your data. Sensitive information should be kept on-premise. Non-sensitive data should be leveraged in the cloud for scalability.
Next, prioritize metadata tagging. Metadata is the sheet music for your AI symphony, guiding retrieval systems to the right data. A healthcare provider saw a 25% reduction in search time by tagging patient records with contextual keywords.
Finally, embrace feedback loops. DeepSeek’s adaptive tuning thrives on user input, much like a conductor refining a performance. Over time, this iterative process improves accuracy and relevance, ensuring your hybrid RAG model evolves with your needs.
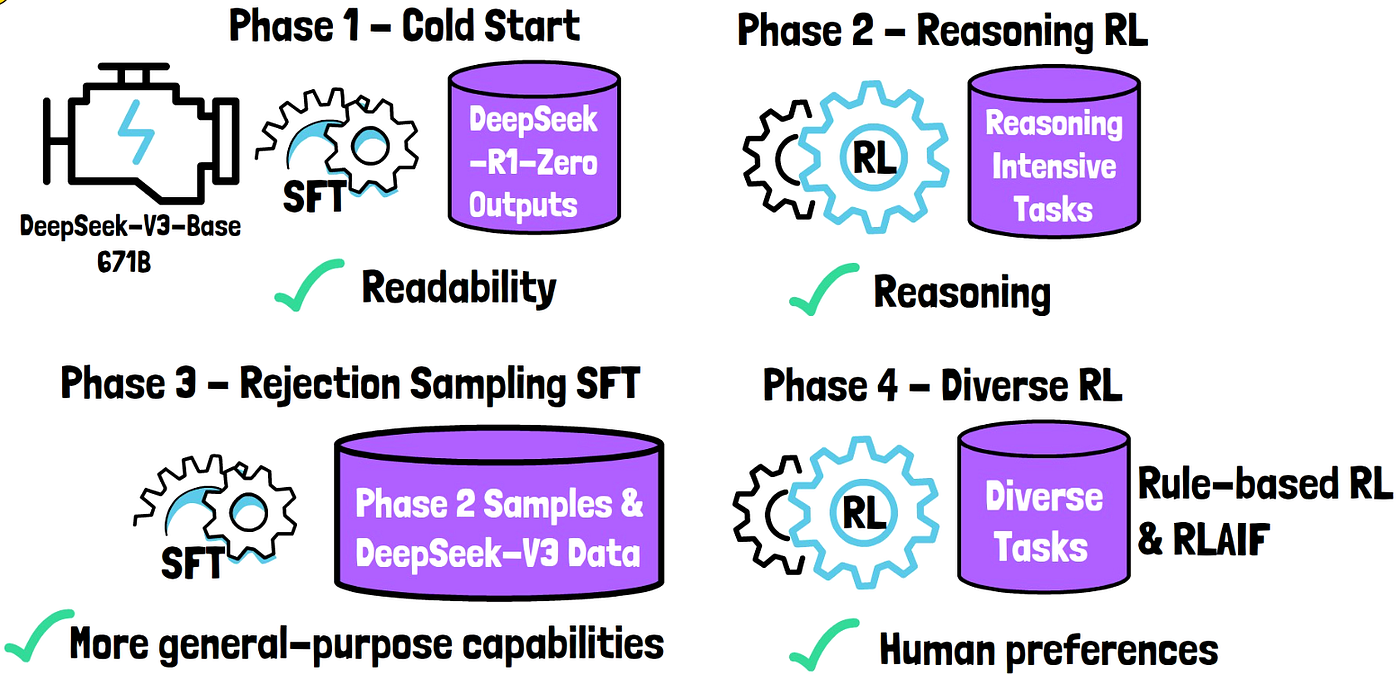
Technical Considerations and Requirements
When integrating DeepSeek into hybrid RAG models, metadata tagging deserves special attention.
Why? Because it’s the backbone of seamless data orchestration.
Metadata ensures that sensitive data stays on-prem while less critical workloads leverage cloud scalability.
However, metadata strategies often fail without proper governance. Enterprises must establish clear tagging protocols and automate them using AI-driven tools.
This reduces human error and ensures compliance with regulations like GDPR or HIPAA. Think of it as creating a digital filing system that’s both intelligent and adaptive.
Overcoming Common Implementation Challenges
Let’s talk about data quality—the silent killer of RAG implementations.
Poor data quality doesn’t just reduce retrieval accuracy; it creates a ripple effect, leading to irrelevant outputs, user frustration, and wasted resources.
The fix? It’s a data-first approach that prioritizes validation and cleansing before anything else.
It works by automating data validation with AI tools which can flag inconsistencies, duplicates, or missing fields in real-time.
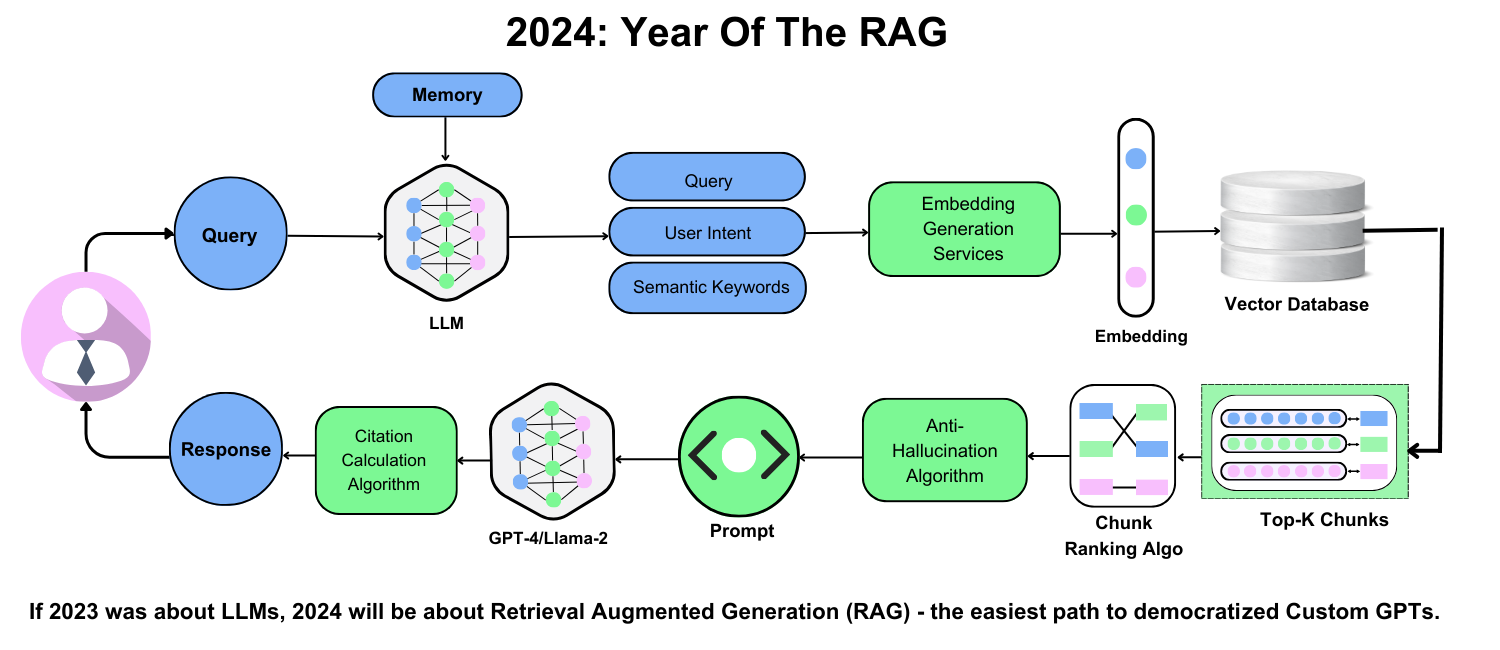
FAQ
What is DeepSeek, and how does it enhance enterprise RAG strategies?
DeepSeek is an advanced open-source AI model designed to revolutionize enterprise RAG strategies by seamlessly integrating retrieval and generation processes. Unlike traditional RAG systems that separate these stages, DeepSeek employs an end-to-end learning pipeline, optimizing performance and reducing latency.
How does the hybrid model approach improve compliance and scalability in enterprise AI?
The hybrid model approach improves compliance and scalability in enterprise AI by strategically balancing the strengths of cloud and on-premise systems.
Sensitive data can be processed securely on-premise, ensuring adherence to regulations like GDPR and HIPAA, while less critical workloads leverage the scalability and cost-efficiency of cloud infrastructure.
What are the key benefits of integrating DeepSeek into existing enterprise systems?
Integrating DeepSeek into existing enterprise systems offers several key benefits, starting with enhanced data orchestration.
DeepSeek’s ability to seamlessly connect with CRMs, ERPs, and other enterprise platforms ensures that data silos are eliminated, enabling a unified and efficient workflow. This integration also improves decision-making by providing real-time, context-aware insights tailored to specific business needs.
How does DeepSeek’s chain-of-thought reasoning contribute to better decision-making?
DeepSeek’s chain-of-thought reasoning contributes to better decision-making by providing a transparent and structured explanation of how conclusions are reached.
This methodology breaks down complex queries into logical steps, allowing users to trace the reasoning process and identify potential gaps or errors. Such transparency not only builds trust in AI-driven outputs but also enables teams to refine and improve decision-making workflows.
What best practices should enterprises follow when implementing DeepSeek in a hybrid RAG model?
Enterprises should prioritize robust metadata tagging for data orchestration and retrieval accuracy. They should also adopt a modular integration approach, allowing them to address specific pain points without overhauling their entire infrastructure. Finally, continuous feedback loops should be implemented to refine DeepSeek’s performance over time.
Final Thoughts on the Future of RAG
If there’s one aspect of RAG that deserves more attention, it’s explainability. As RAG systems grow more complex, trust becomes the currency of adoption. Enterprises need to know why a system made a decision—not just the result.
But here’s the challenge: most RAG systems prioritize speed over transparency. This is where chain-of-thought reasoning, like DeepSeek’s, shines. Breaking down the decision path balances clarity with performance.
Actionable insight? Build explainability into your RAG workflows from day one. Use visual tools or dashboards to demystify outputs. The result? Better decisions, stronger trust, and a future-proof AI strategy.