DeepSeek R1 Versus Leading AI Models: A Comprehensive Analysis
DeepSeek R1 is competing with top AI models—how does it compare? This analysis breaks down its performance, efficiency, and capabilities, helping you understand its strengths, weaknesses, and potential applications in the evolving AI landscape.
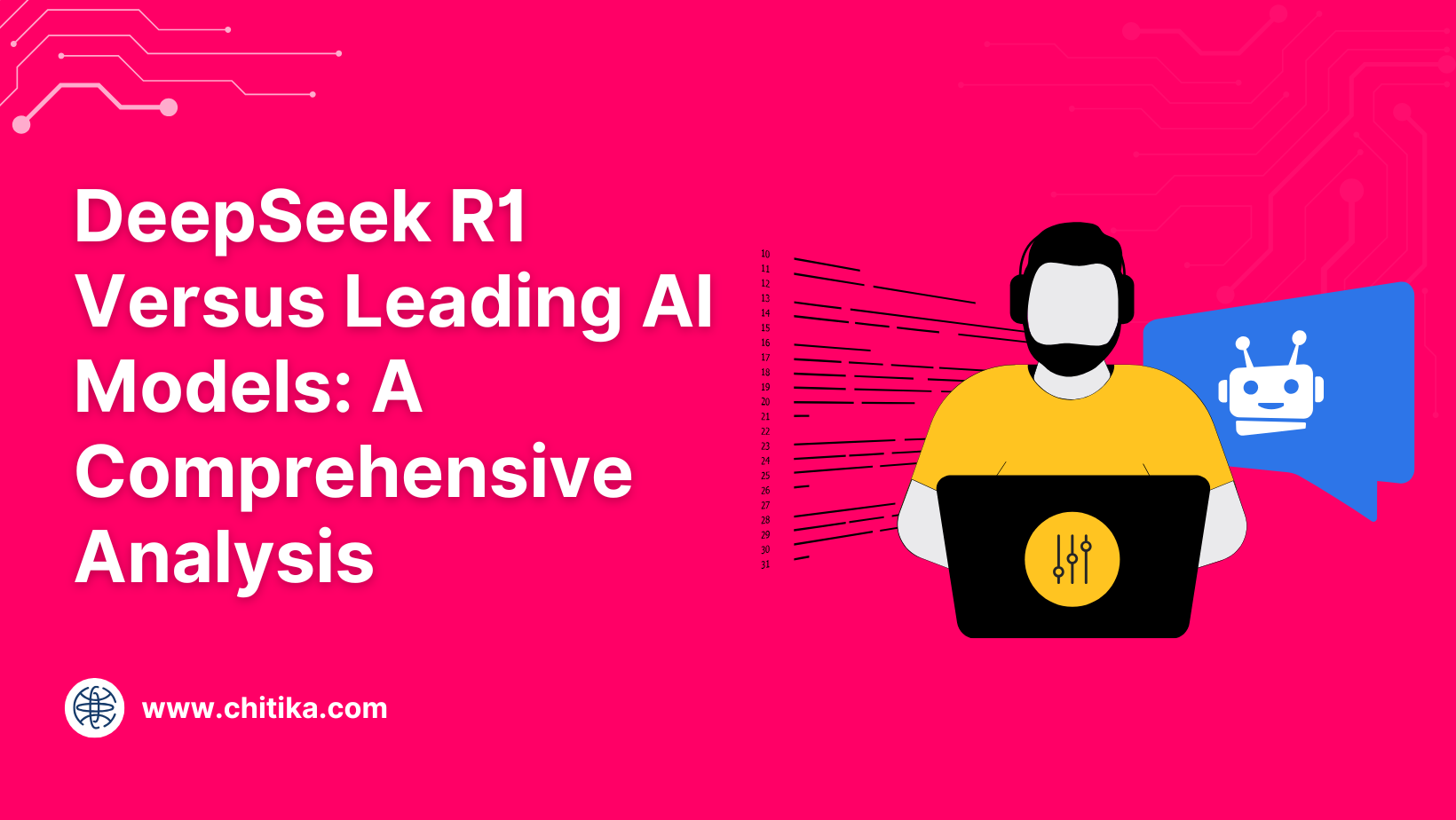
In January 2025, a Chinese startup quietly disrupted the AI industry’s entrenched norms.
DeepSeek R1, an open-source language model, emerged not with the fanfare of billion-dollar marketing campaigns but with a startling proposition: rivalling the performance of industry titans like OpenAI’s GPT-4 at a fraction of the cost.
Yet, its rise also raised questions: Could a model built on cost-conscious principles truly compete with the giants? And what does this mean for the future of AI innovation?
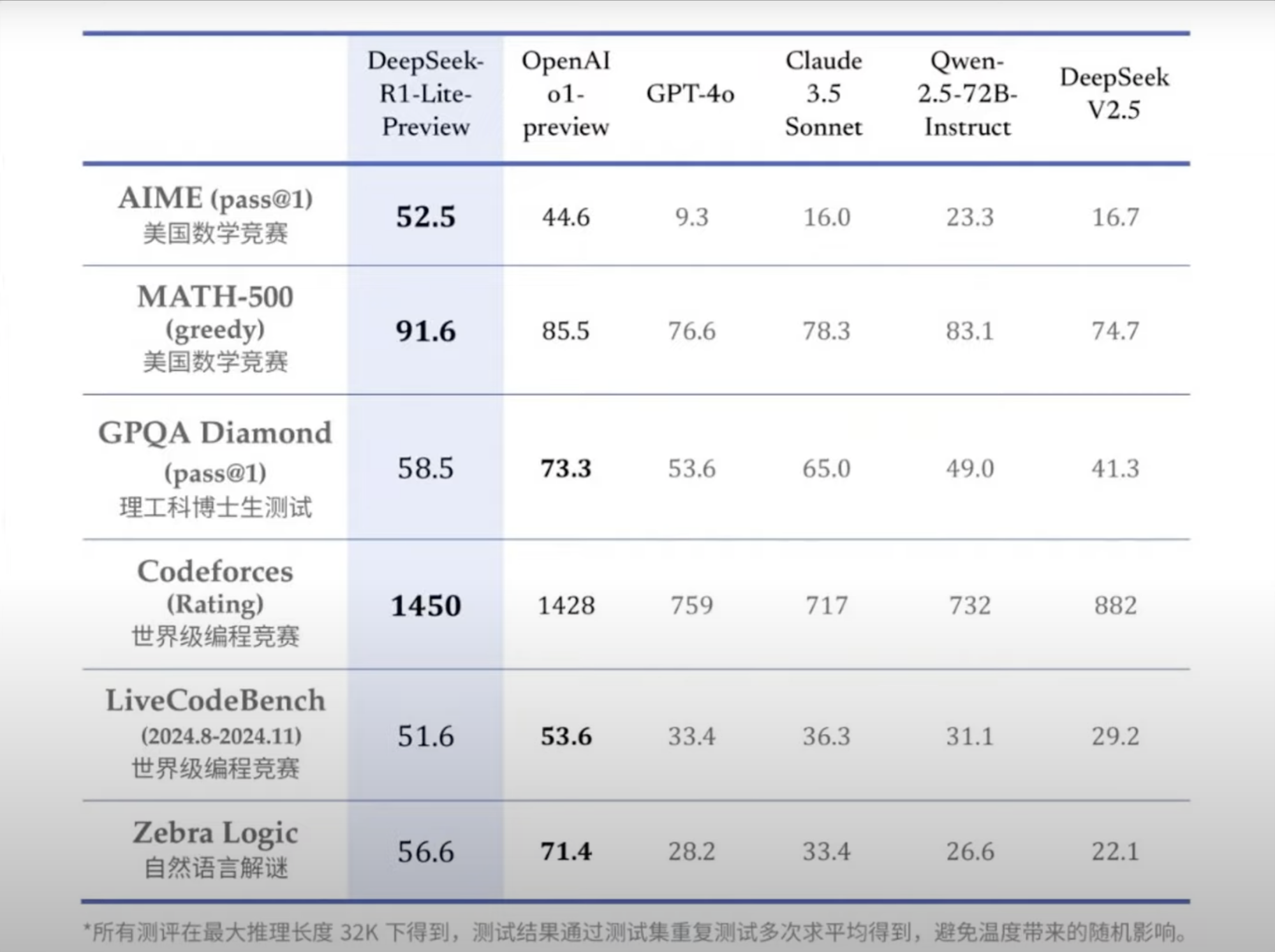
Technical Architecture of DeepSeek R1
DeepSeek R1’s architecture exemplifies a masterclass in balancing complexity with efficiency.
At its core lies the Mixture of Experts (MoE) framework, a design that activates only task-relevant expert networks from its 671 billion parameters, with just 37 billion engaged per computation.
A standout feature is its Multi-head Latent Attention (MLA) mechanism, which enhances the model’s ability to capture intricate patterns across diverse datasets.
For instance, in sentiment analysis, MLA enables nuanced understanding by dynamically focusing on context-specific data points, outperforming monolithic architectures in both speed and accuracy.
Contrary to the misconception that efficiency sacrifices performance, R1’s modularity allows seamless adaptation to shifting data landscapes.
This adaptability is evident in real-time logistics, where R1 optimizes routes under fluctuating conditions, saving enterprises millions annually.
By prioritizing specialization over brute force, DeepSeek R1 challenges the industry’s obsession with scale, offering a blueprint for sustainable AI innovation.
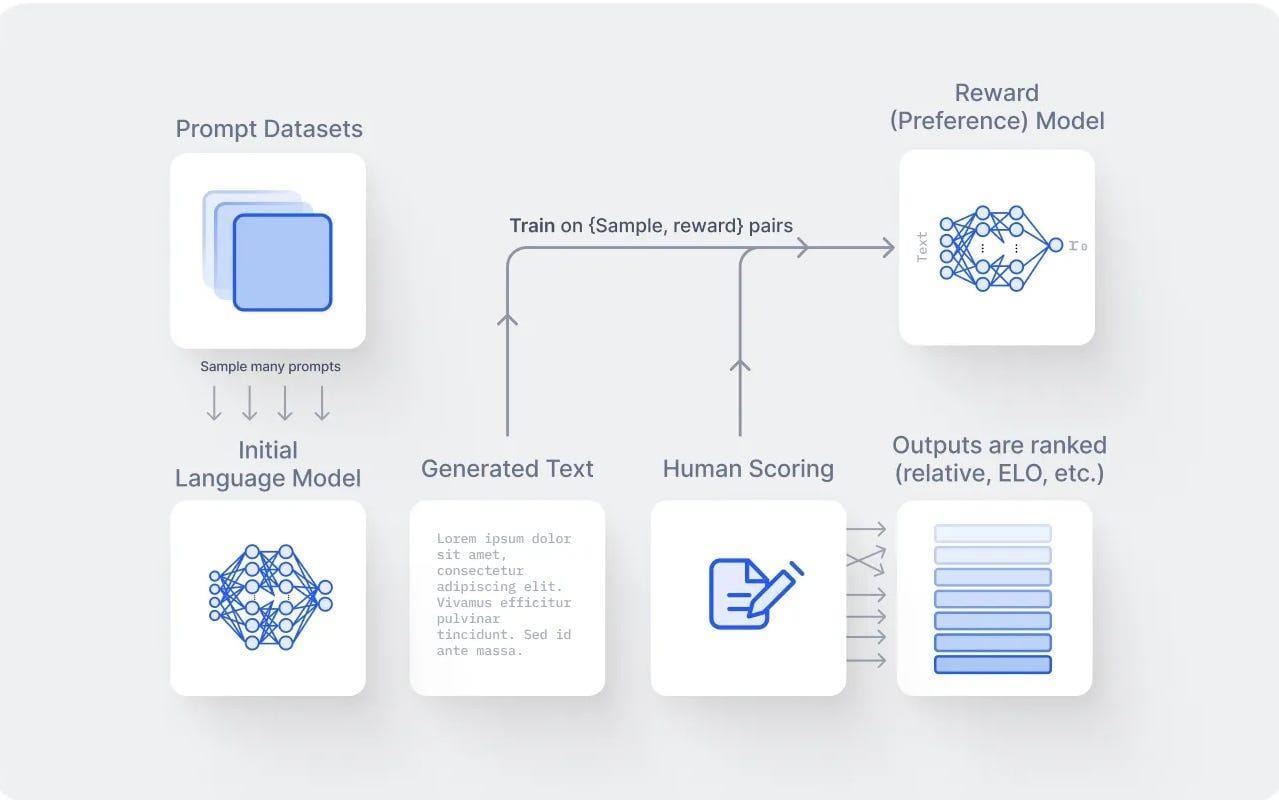
Training Methodologies
A standout aspect of DeepSeek R1’s training methodologies is its use of automatically verifiable training data, particularly in domains like mathematics and coding.
This approach ensures that the model is trained on datasets where correctness can be algorithmically validated, significantly reducing the risk of errors introduced by human labelling.
Another critical innovation is the Group Relative Policy Optimization (GRPO) algorithm, which refines reinforcement learning by tailoring reward functions to specific tasks.
Unlike traditional methods that generalize across tasks, GRPO ensures that computational resources are directed toward high-impact learning objectives.
For instance, in supply chain optimization, this methodology enables R1 to process complex, multi-variable scenarios in real-time, delivering actionable insights that drive efficiency and cost savings.
Performance and Reasoning Capabilities
DeepSeek R1 redefines performance by excelling in reasoning-centric tasks, a domain where many AI models falter.
Unlike traditional models that rely on pattern recognition, R1 employs chain-of-thought reasoning, enabling it to break down complex problems into manageable steps.
For instance, in the MATH-500 benchmark, R1 achieved an impressive 97.3%, outperforming even proprietary giants like OpenAI’s o1 model.
Looking ahead, R1’s reasoning capabilities challenge the industry to prioritize intelligent design over brute computational force, paving the way for more accessible, high-performance AI solutions.
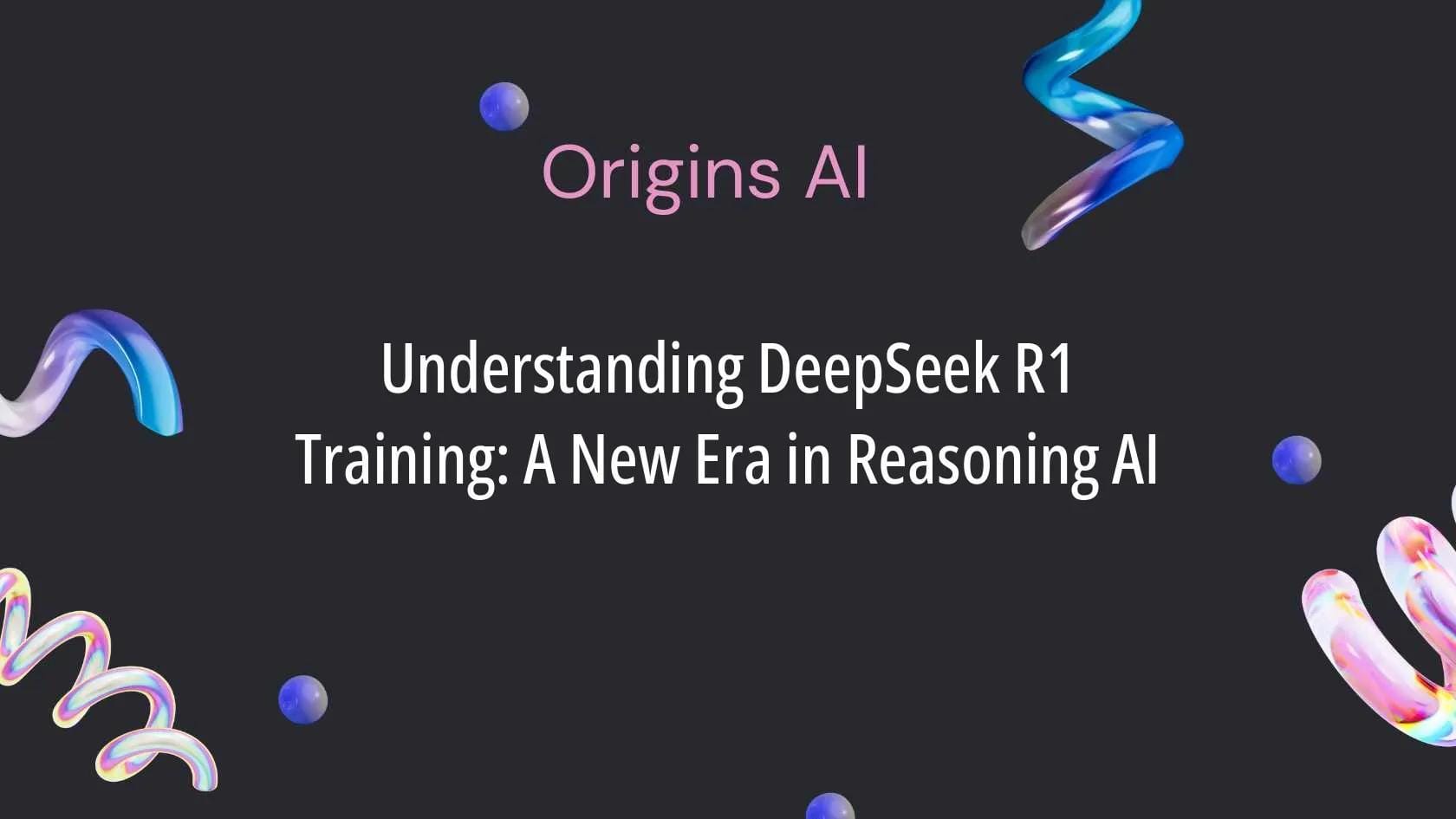
Benchmark Results and Task Proficiency
DeepSeek R1’s standout performance in benchmarks like Codeforces and MATH-500 highlights its ability to excel in tasks requiring both precision and adaptability.
A key factor behind this success is its dynamic routing algorithm, which intelligently activates task-relevant expert networks within its Mixture of Experts (MoE) framework.
In real-world applications, this precision translates into significant advantages.
For example, in fraud detection systems, R1’s ability to identify anomalies with minimal false positives has saved financial institutions millions annually.
Its multi-token prediction capabilities further enhance its utility in domains like natural language generation, where speed and contextual accuracy are critical.
Interestingly, R1’s modular design challenges the industry’s reliance on monolithic architectures. By focusing on contextual adaptability, it mirrors principles from biological neural networks, where energy is conserved without sacrificing functionality.
This paradigm shift suggests a future where AI models prioritize specialization over scale, enabling more sustainable and domain-specific innovations across industries.
Comparisons with Leading AI Models
DeepSeek R1 challenges the dominance of leading AI models like OpenAI’s GPT-4 by prioritizing efficiency and specialization over brute computational scale.
While GPT-4 exemplifies the “bigger is better” philosophy, R1’s Mixture of Experts (MoE) framework activates only task-relevant parameters, achieving comparable performance with significantly fewer resources.
A striking contrast lies in their training methodologies. GPT-4 relies heavily on vast, human-labeled datasets, whereas R1 employs automatically verifiable training data, particularly in domains like mathematics and coding.
This not only reduces costs but also enhances reasoning accuracy, as seen in its superior performance on SWE-bench Verified.
Interestingly, R1’s modularity mirrors biological neural networks, where energy is conserved without sacrificing functionality.
This approach challenges the misconception that smaller models lack depth, proving that targeted expertise can rival general-purpose giants.
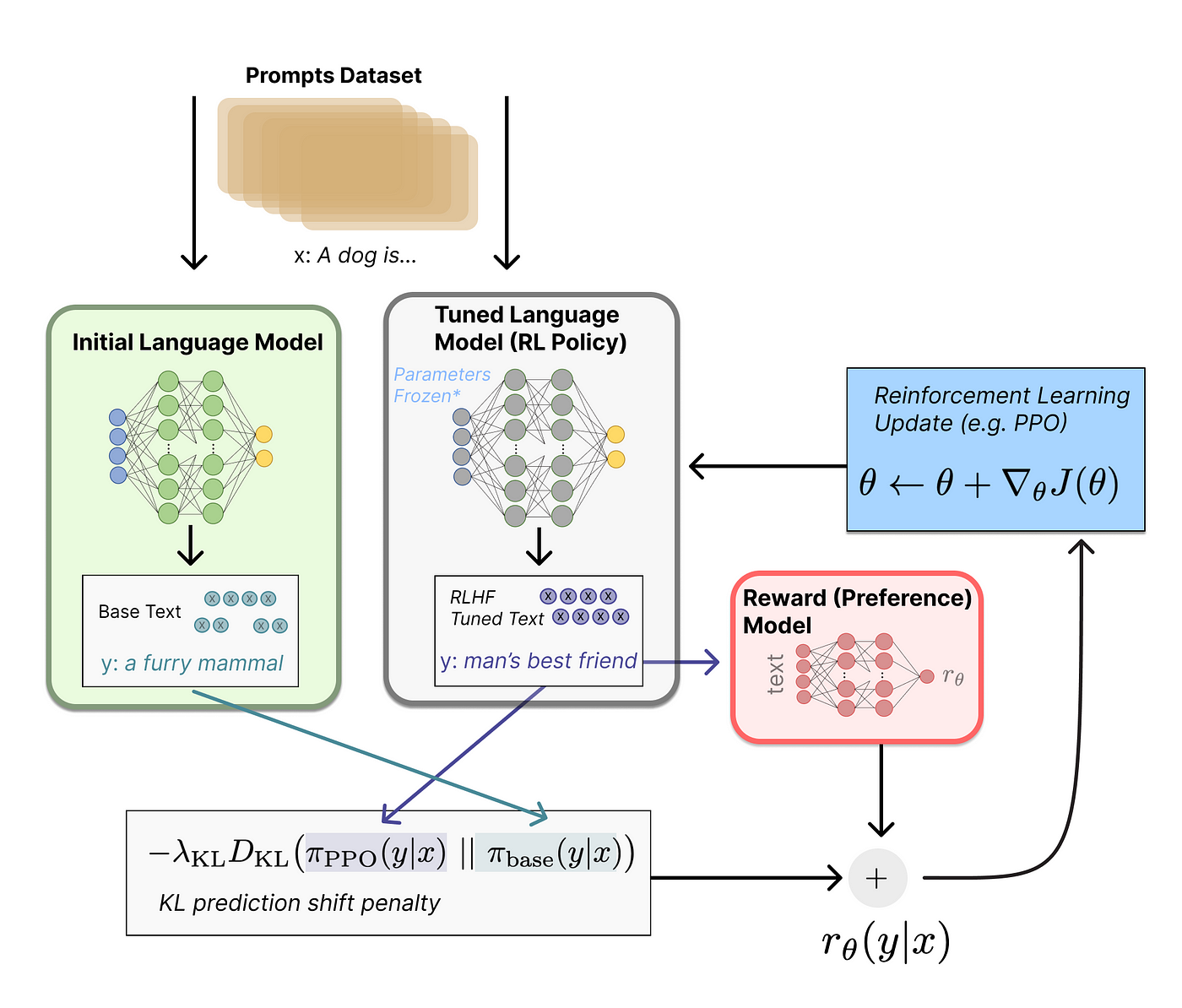
Performance Metrics Against GPT-4 and Others
DeepSeek R1’s standout performance on reasoning-centric benchmarks like MATH-500 and SWE-bench Verified highlights its precision-driven architecture.
This efficiency not only reduces computational overhead but also enhances task-specific accuracy.
A lesser-known factor driving R1’s success is its use of automatically verifiable training data.
In domains like mathematics, where correctness can be algorithmically validated, this approach ensures higher data quality, directly improving reasoning capabilities. For example, in financial modeling, R1’s precision has enabled firms to optimize trading algorithms, reducing latency and increasing profitability in volatile markets.
Interestingly, R1’s modularity draws parallels to distributed computing, where resource allocation is optimized for specific tasks. This challenges the conventional wisdom that larger models inherently perform better, proving that targeted specialization can rival general-purpose systems.
Looking forward, R1’s approach suggests a framework for future AI development: prioritizing contextual adaptability and domain-specific expertise over sheer scale, paving the way for more sustainable and accessible innovations.
Cost Efficiency and Market Implications
DeepSeek R1’s cost efficiency is driven by its focus on software optimization over expensive hardware.
Using techniques like 8-bit floating-point precision and advanced compression, R1 reduces computational needs significantly.
As a result, training R1 required only 2,000 Nvidia H100 GPUs, costing approximately $5.5 million. In contrast, OpenAI’s GPT-4 training expenses exceeded $100 million.
A critical but often overlooked advantage is R1’s open-source model, which broadens access to high-quality AI.
Small businesses and startups can now implement powerful AI systems locally or through affordable cloud services like Hugging Face or AWS. This openness removes barriers, allowing companies in developing countries to participate in AI innovation.
Market shifts following R1’s launch highlight its disruptive impact. Nvidia’s market value dropped by over $600 billion in early 2025, partly due to reduced demand projections for high-end GPUs.
This event signaled a transition from hardware-intensive AI models to more software-efficient solutions, challenging assumptions that advanced hardware dictates AI success.
Implications and Future Directions
DeepSeek R1’s emergence signals a paradigm shift in AI development, where efficiency and accessibility redefine competitive advantage.
By achieving top-tier performance with minimal resources, R1 challenges the entrenched belief that AI progress is tied to brute computational power.
This shift mirrors the transition from mainframe computing to personal computers, democratizing access and fostering innovation.
One striking implication is the potential for regional AI empowerment. For example, startups in developing economies can now deploy R1 locally, bypassing the prohibitive costs of proprietary models.
This accessibility could catalyze breakthroughs in areas like precision agriculture, where R1’s adaptability optimizes resource use in real time, addressing food security challenges.
However, scalability remains a concern. As demand for R1 grows, ensuring infrastructure compatibility without diluting its cost advantages will be critical.
Interestingly, its modular design offers a solution, enabling tailored deployments that balance performance with resource constraints.
Looking forward, R1’s success may inspire a broader industry pivot toward lean, domain-specific AI, fostering sustainable innovation while challenging the dominance of monolithic architectures.
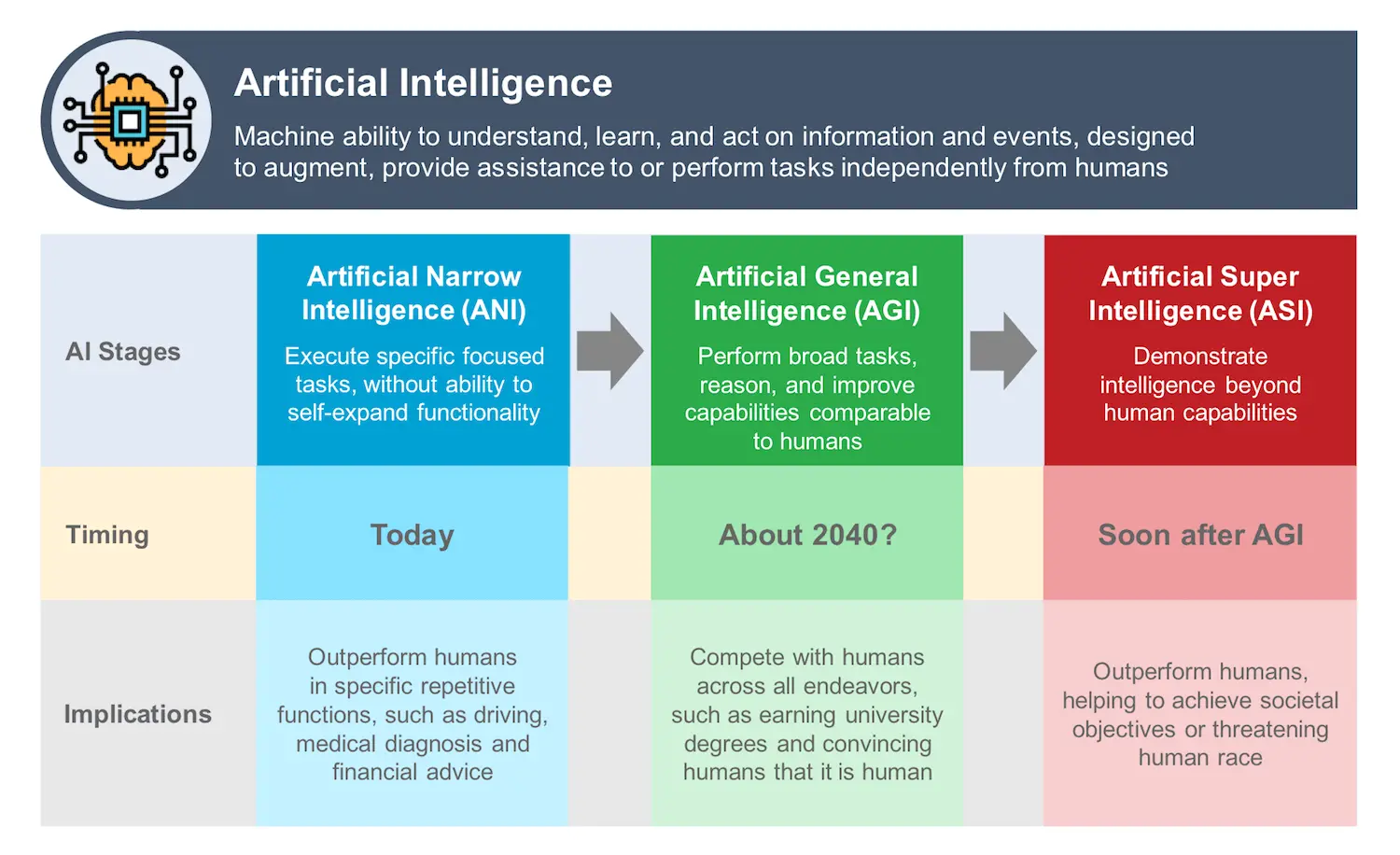
FAQ About DeepSeek R1 Versus AI Models
What makes DeepSeek R1 different from GPT-4?
DeepSeek R1 uses a Mixture of Experts (MoE) architecture, activating only essential parameters per task, unlike GPT-4’s resource-heavy approach. It achieves similar performance efficiently, emphasizing domain-specific accuracy and affordability.
How does the Mixture of Experts (MoE) framework benefit DeepSeek R1?
The MoE framework enhances R1’s performance by selectively activating relevant parts of the model. This reduces computational costs, boosts speed, and maintains accuracy, ideal for specialized tasks like fraud detection or financial modeling.
What are the key real-world applications of DeepSeek R1?
DeepSeek R1 excels in healthcare diagnostics, fraud detection, personalized education, and coding assistance. Its efficiency and precision make it a strong fit for sectors demanding real-time, context-aware solutions.
Why is DeepSeek R1’s open-source nature important?
Being open-source democratizes access to high-performance AI, enabling customization and rapid innovation. Businesses of all sizes can integrate and adapt R1 affordably, promoting collaboration and broadening AI adoption.
What does DeepSeek R1’s cost efficiency mean for AI’s future?
R1’s cost efficiency disrupts the AI landscape, lowering barriers for small enterprises and startups. Its lean architecture shifts focus from expensive hardware to smart algorithms, paving the way for sustainable, scalable AI innovation.
Conclusion
DeepSeek R1 represents a significant turning point in AI development, proving that efficiency and specialization can rival the power of conventional, resource-intensive models.
Its innovative approach, particularly the Mixture of Experts architecture, challenges the industry’s prevailing mindset that bigger is always better.
By achieving comparable or superior performance at a fraction of the cost, R1 has democratized access to high-level AI, empowering smaller enterprises and emerging economies to innovate without prohibitive barriers.