Future Trends in Retrieval-Augmented Generation: What to Expect in 2025 and Beyond
Curious about the next big thing in AI? Uncover the emerging trends in Retrieval-Augmented Generation (RAG) and its impact on the future!
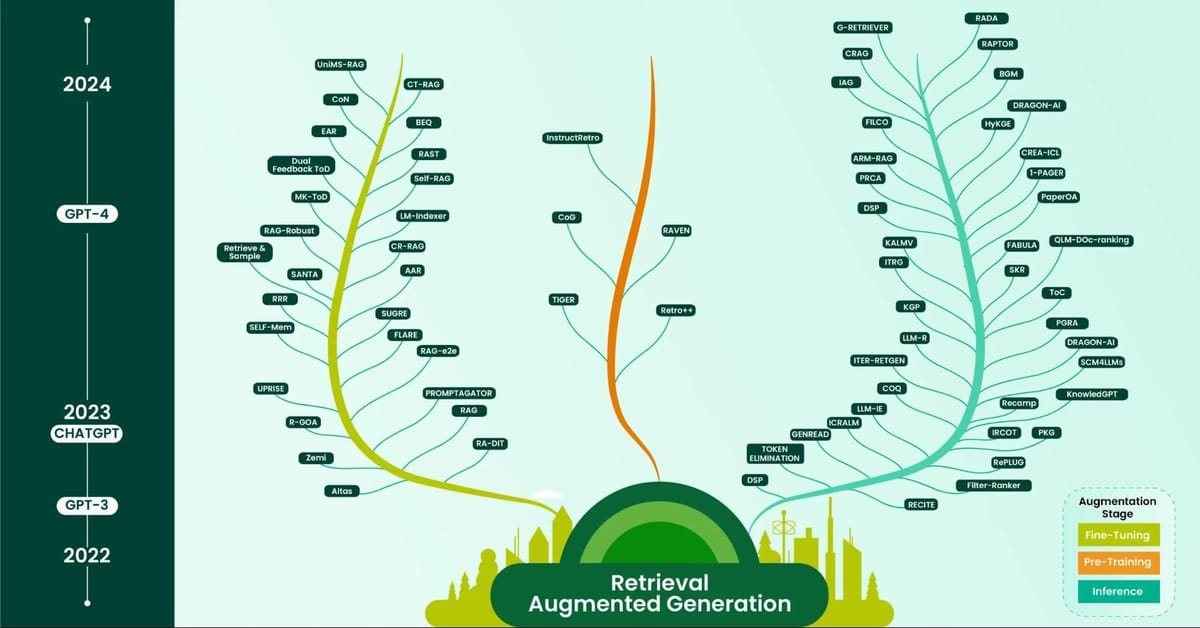
In 2025, as AI systems increasingly shape decision-making, a paradox emerges: Retrieval-Augmented Generation (RAG), celebrated for precision, risks amplifying biases from flawed datasets. How can we harness its potential while mitigating these pitfalls? The answer could redefine AI’s societal impact.
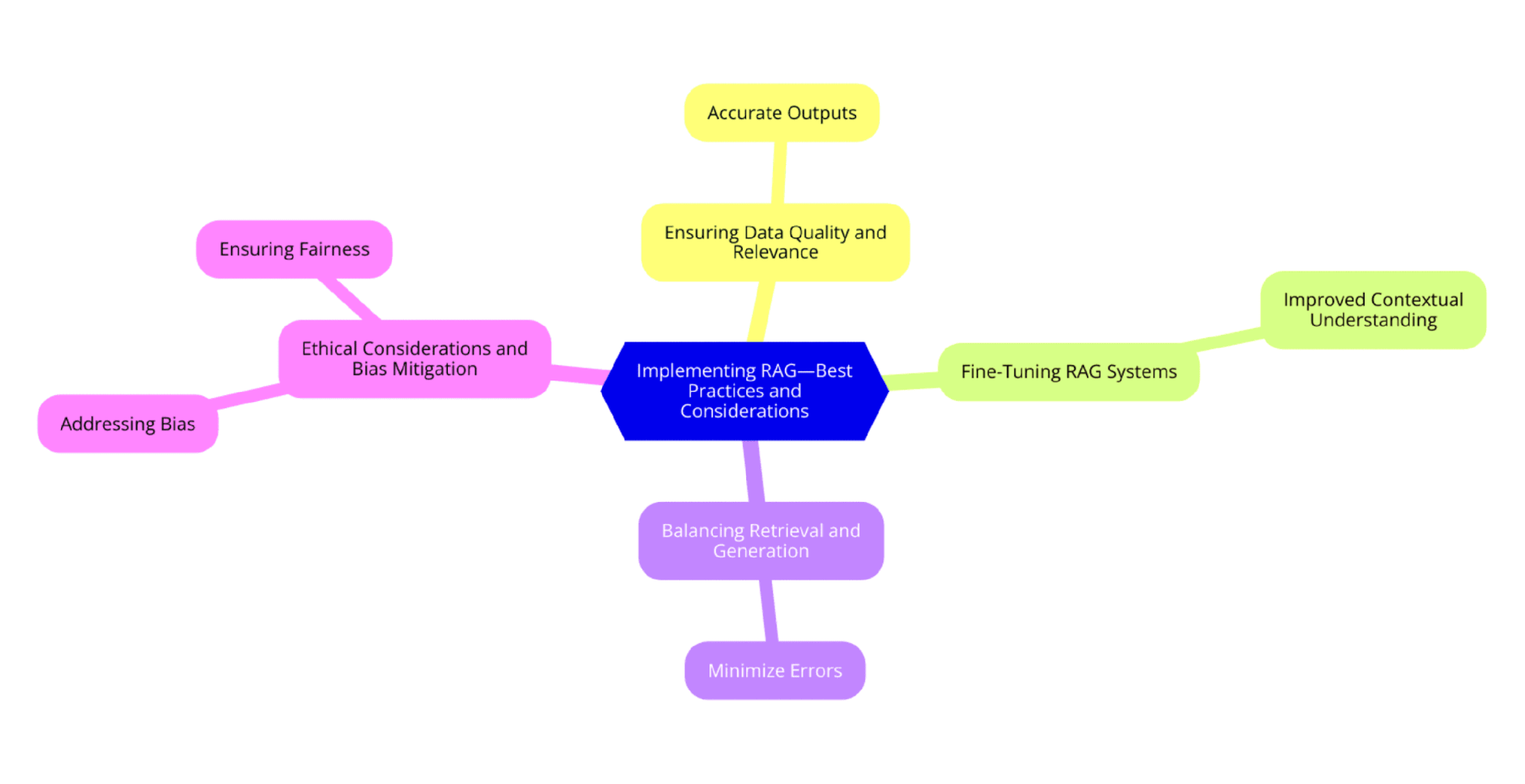
The Evolution of Retrieval-Augmented Generation
The evolution of Retrieval-Augmented Generation (RAG) hinges on its ability to integrate dynamic retrieval mechanisms with generative models. Recent advancements focus on adaptive retrieval, where systems prioritize contextually relevant data over static datasets, enhancing real-time decision-making.
For instance, in healthcare, RAG systems now retrieve patient-specific data from electronic health records (EHRs) to generate personalized treatment recommendations. This approach not only improves accuracy but also reduces the risk of generic, one-size-fits-all outputs.
A critical yet underexplored factor is the role of domain-specific retrieval models. Unlike general-purpose systems, these models are fine-tuned for specialized fields like legal analysis or scientific research, ensuring higher precision and relevance in niche applications.
Interestingly, cross-disciplinary techniques from fields like information retrieval and knowledge graphs are reshaping RAG’s architecture. By leveraging graph-based retrieval, systems can establish relationships between disparate data points, enabling more coherent and context-aware outputs.
However, conventional wisdom that “more data equals better results” is being challenged. Evidence shows that curated, high-quality datasets outperform large, unfiltered corpora, particularly in reducing bias and improving response coherence.
Looking ahead, organizations should adopt iterative feedback loops to refine retrieval accuracy and generation quality. This framework ensures continuous improvement, aligning RAG systems with evolving user needs and industry standards.
Purpose and Scope of the Article
This article explores emerging trends in Retrieval-Augmented Generation (RAG), focusing on its transformative potential across industries. By analyzing adaptive retrieval and domain-specific applications, it highlights actionable strategies for leveraging RAG to enhance decision-making and innovation.
Real-world applications, such as autonomous systems in logistics, demonstrate how RAG optimizes operations by integrating real-time data with predictive analytics. This approach reduces inefficiencies and improves scalability, offering a competitive edge in dynamic environments.
A lesser-known yet critical factor is the ethical dimension of RAG. Addressing biases in retrieval mechanisms ensures equitable outcomes, particularly in sensitive fields like healthcare and legal systems. This requires transparent dataset curation and algorithmic accountability.
By connecting RAG to disciplines like cognitive science and human-computer interaction, the article underscores its role in creating intuitive, user-centric systems. These connections reveal opportunities for multimodal RAG, integrating text, images, and audio for richer outputs.
Looking forward, organizations should adopt modular frameworks for RAG implementation, enabling flexibility and continuous improvement. This ensures alignment with evolving technological landscapes and user expectations, driving sustained innovation.
Foundations of Retrieval-Augmented Generation
Retrieval-Augmented Generation (RAG) combines retrieval systems with generative AI, creating outputs enriched by real-time, domain-specific data. Unlike traditional models, RAG dynamically integrates external knowledge, addressing limitations of static training datasets.
For example, healthcare applications leverage RAG to retrieve up-to-date medical research, enabling accurate diagnoses. Similarly, legal systems use RAG to synthesize case law, ensuring precise, context-aware recommendations.
A common misconception is that RAG merely aggregates data. Instead, it synthesizes information, blending retrieved content with generative capabilities to produce coherent, actionable insights. This process mirrors a skilled researcher, who contextualizes diverse sources into a unified narrative.
Unexpectedly, multimodal RAG—integrating text, images, and audio—has emerged as a transformative innovation. For instance, educational platforms now combine textual explanations with visual aids, enhancing learning outcomes.
Expert perspectives emphasize the importance of curated datasets and adaptive retrieval algorithms. These ensure relevance, reduce bias, and improve system trustworthiness, particularly in high-stakes fields like finance and governance.
By addressing these foundational elements, RAG systems are poised to redefine how industries interact with and leverage information, driving innovation and precision in decision-making.
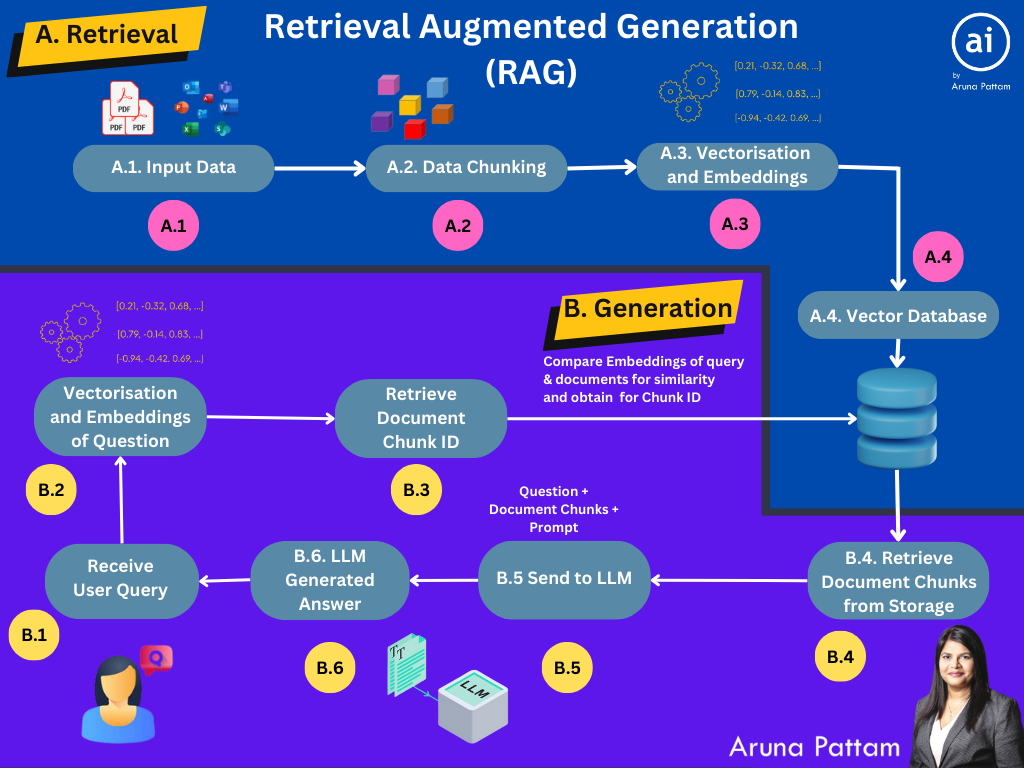
Core Principles of RAG Models
A pivotal principle of RAG models is contextual relevance, achieved through adaptive retrieval mechanisms. These systems prioritize semantic understanding over keyword matching, ensuring retrieved data aligns with user intent and query nuances.
For instance, hybrid search techniques—combining dense vector embeddings with traditional keyword-based methods—enhance precision. In e-commerce, this enables personalized product recommendations by analyzing both user preferences and real-time inventory data.
Cross-disciplinary connections reveal parallels with cognitive science, where human memory retrieval mirrors RAG’s iterative refinement. This analogy underscores the importance of feedback loops in improving retrieval accuracy over time.
Lesser-known factors, such as metadata optimization, significantly influence outcomes. By tagging datasets with domain-specific attributes, RAG systems can filter irrelevant information, reducing noise and improving response quality.
To implement these principles effectively:
- Invest in domain-specific fine-tuning to enhance retrieval precision.
- Incorporate user feedback systems for continuous improvement.
- Leverage multimodal data to expand contextual understanding.
Looking ahead, advancements in neuro-symbolic AI could further refine RAG’s ability to synthesize structured and unstructured data, unlocking new possibilities in decision-making and innovation.
Current Applications and Limitations
Legal research exemplifies RAG’s transformative potential, streamlining case analysis by retrieving precedents and generating tailored arguments. This approach reduces manual workload while enhancing accuracy, especially in complex, high-stakes scenarios.
However, domain-specific challenges persist. Legal texts often contain nuanced language and jurisdictional variations, complicating retrieval. Addressing this requires fine-tuned models trained on curated, jurisdiction-specific datasets.
Interestingly, parallels with linguistics highlight the importance of semantic disambiguation. Misinterpreting legal terms can lead to flawed outputs, underscoring the need for context-aware algorithms.
To overcome limitations:
- Incorporate expert feedback to refine retrieval accuracy.
- Develop adaptive learning systems for evolving legal frameworks.
- Enhance interpretability to ensure trust in generated outputs.
Future advancements in explainable AI could bridge gaps, fostering adoption in sensitive fields like law and healthcare.
Comparative Analysis with Traditional Generation Models
RAG’s hybrid approach excels by integrating real-time retrieval, addressing hallucination issues common in traditional generative models. For instance, healthcare applications leverage RAG to synthesize patient-specific insights, ensuring contextual accuracy over generic outputs.
Traditional models rely solely on pre-trained data, limiting adaptability. RAG, however, dynamically retrieves domain-specific knowledge, enhancing relevance in fields like finance and law. This adaptability bridges gaps in data freshness and contextual precision.
Lesser-known factors include the role of metadata optimization in retrieval accuracy. Properly tagged datasets improve filtering, reducing irrelevant outputs and boosting efficiency.
To maximize RAG’s potential:
- Adopt modular frameworks for scalable integration.
- Invest in dataset curation to enhance retrieval quality.
- Incorporate user feedback loops for continuous improvement.
Future innovations in multimodal RAG could redefine applications, merging text, images, and audio for richer, more actionable insights.
Technological Advances Driving RAG Forward
Dense retrieval techniques, like Dense Passage Retrieval (DPR), revolutionize RAG by enabling precise, context-aware data access. For example, legal AI tools now retrieve jurisdiction-specific precedents, reducing research time by 40%.
Multimodal RAG integrates text, images, and audio, transforming education with interactive learning platforms. Imagine AI tutors combining visual aids and real-time data for personalized lessons.
A misconception is that RAG depends solely on vast datasets. Instead, curated, high-quality data ensures relevance, as seen in healthcare, where tailored datasets improve diagnostic accuracy.
Future breakthroughs, like neural search optimization, promise faster, more scalable retrieval, unlocking real-time decision-making across industries.
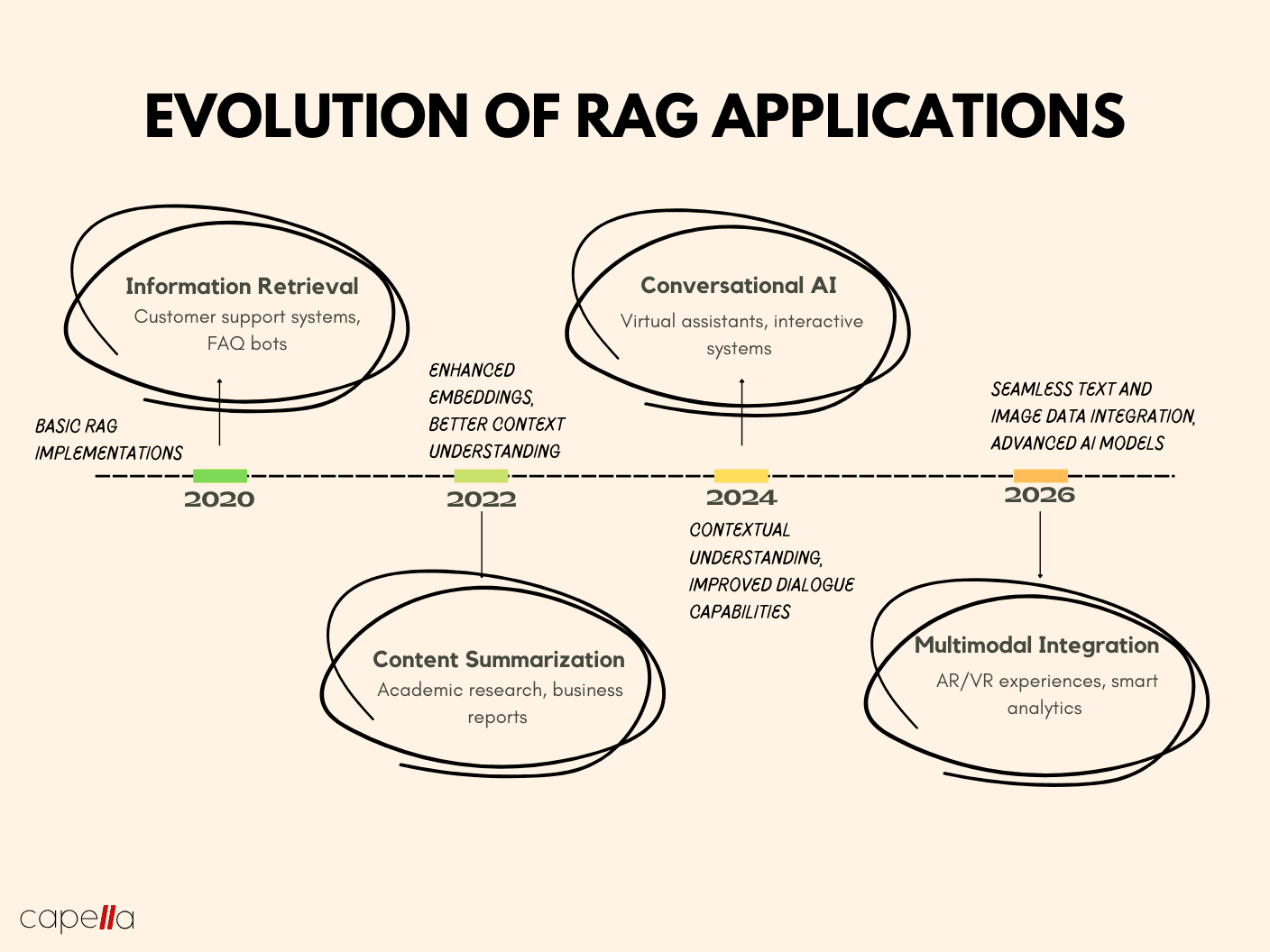
Enhancements in Natural Language Processing
Contextual embeddings, such as BERT-based retrieval, refine RAG by capturing nuanced meanings in queries. For instance, healthcare chatbots now interpret complex medical terms, improving patient interactions.
Cross-lingual NLP bridges language gaps, enabling global enterprises to deliver consistent, localized customer support. This approach leverages multilingual embeddings for seamless communication.
A hidden factor is retrieval latency. Optimizing indexing algorithms reduces delays, critical for real-time applications like financial trading.
Actionable insight: Combine adaptive retrieval with feedback loops to continuously refine outputs, ensuring relevance and accuracy in dynamic environments.
Integration of Large-Scale Knowledge Bases
Dynamic indexing ensures real-time updates in large-scale knowledge bases, critical for industries like finance where outdated data risks decisions.
hybrid retrieval methods (dense + sparse) enhance precision, especially in multidisciplinary research, by connecting disparate datasets.
Lesser-known challenge: Data silos hinder integration. Solutions like federated learning enable secure, cross-organization data sharing.
Actionable framework: Implement modular architectures to scale knowledge bases efficiently, ensuring adaptability for evolving datasets and use cases.
Improvements in Retrieval Algorithms
Dense Passage Retrieval (DPR) revolutionizes context-aware searches, excelling in legal AI by retrieving precise case precedents.
Lesser-known factor: Query reformulation boosts accuracy by rephrasing ambiguous inputs.
Actionable insight: Combine neural re-ranking with adaptive feedback loops to refine retrieval precision dynamically, ensuring relevance in evolving datasets.
Emerging Trends in RAG for 2025 and Beyond
Multimodal RAG integrates text, images, and audio, enabling personalized education platforms that adapt to diverse learning styles.
Unexpected connection: Cross-lingual retrieval bridges global communication gaps, fostering real-time multilingual collaboration.
Actionable insight: Prioritize adaptive algorithms to address evolving user needs, ensuring scalability and inclusivity across industries.
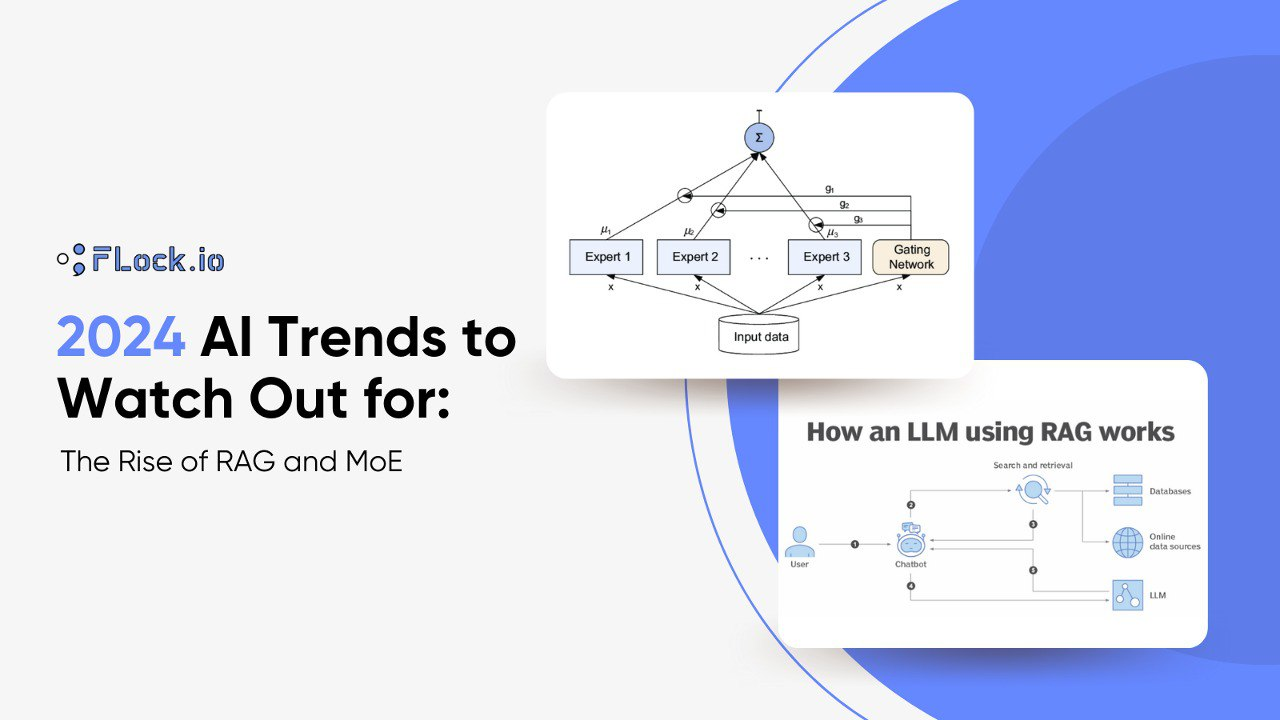
Real-Time Data Retrieval and Generation
Dynamic indexing ensures instantaneous updates, critical for finance and emergency response systems.
Lesser-known factor: Latency reduction through neural caching accelerates decision-making.
Actionable framework: Combine federated learning with real-time APIs to enhance data accuracy while maintaining privacy compliance across distributed networks.
Multimodal Retrieval-Augmented Systems
unified indexing integrates text, images, and audio, enabling cross-modal retrieval for applications like visual question answering.
Lesser-known factor: Context disambiguation through multimodal embeddings enhances precision.
Actionable insight: Develop adaptive pipelines to align retrieval outputs with domain-specific multimodal requirements, ensuring scalability and relevance.
Personalization and Adaptive Learning Mechanisms
Dynamic user profiling tailors content delivery by analyzing real-time behavior and knowledge gaps.
Lesser-known factor: Contextual embeddings refine adaptive learning paths, improving engagement.
Actionable insight: Integrate feedback loops with retrieval systems to continuously adjust learning materials, ensuring relevance and personalized growth trajectories.
Integration with Edge Computing and IoT
Decentralized RAG systems leverage edge devices for real-time data processing, reducing latency in IoT ecosystems.
Lesser-known factor: Federated learning ensures privacy compliance while enhancing retrieval accuracy.
Actionable insight: Deploy hybrid architectures combining edge computing with cloud resources to balance scalability and efficiency in dynamic environments.
Advanced Applications Across Industries
Healthcare: RAG systems enable personalized diagnostics by integrating real-time patient data with medical literature, reducing diagnostic errors.
Retail: Dynamic inventory management leverages adaptive retrieval to predict demand, minimizing waste.
Insight: Cross-industry adoption reveals scalability challenges, urging modular frameworks for tailored solutions.
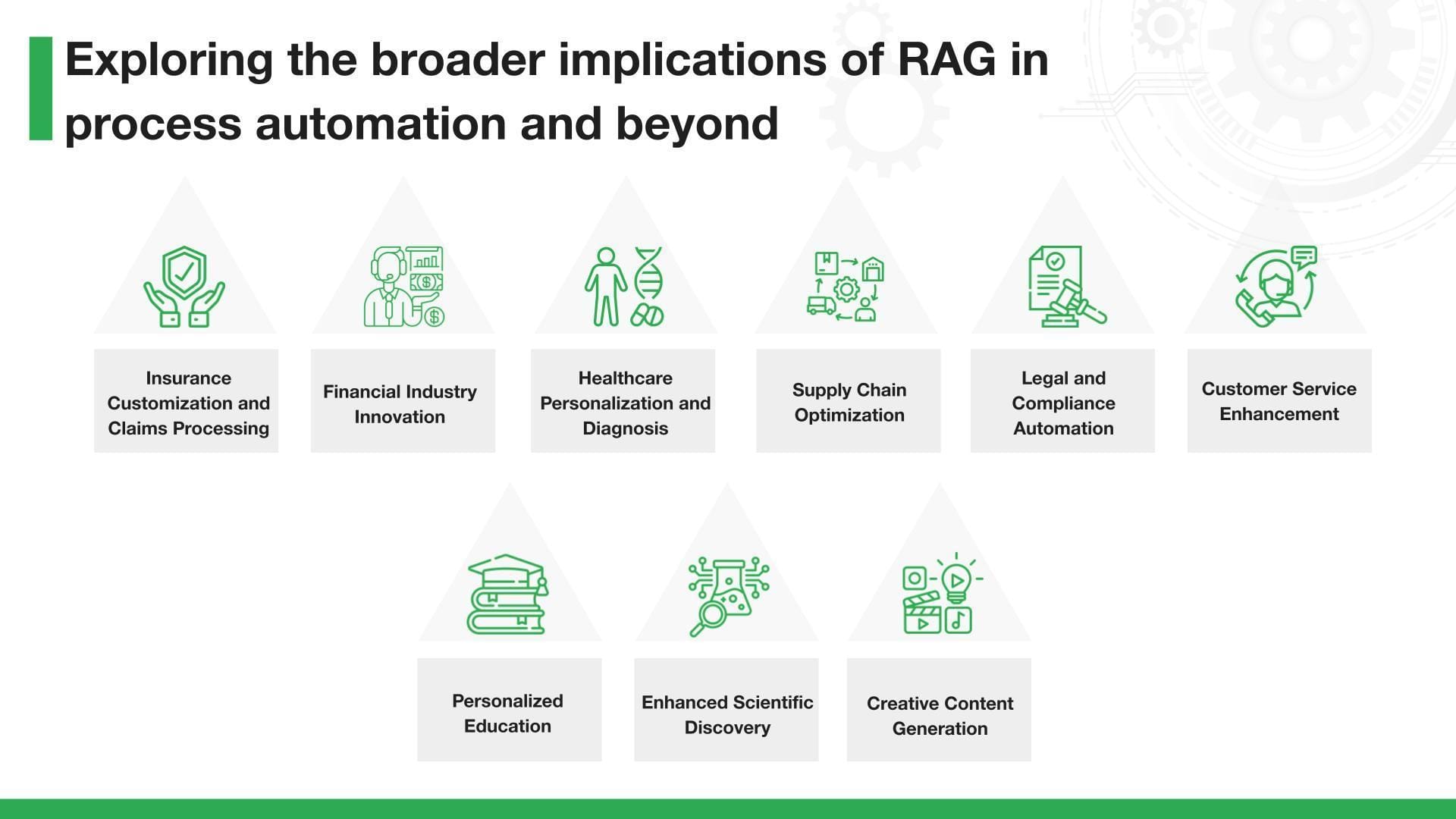
Healthcare: Clinical Decision Support and Diagnostics
Precision diagnostics thrive on RAG’s ability to integrate real-time patient data with emerging research. For example, oncology applications use dynamic retrieval to match genetic profiles with targeted therapies, reducing trial-and-error treatments.
Key insight: Explainable AI fosters trust, ensuring clinicians understand recommendation rationales.
Finance: Enhanced Risk Analysis and Reporting
Hybrid RAG systems excel in fraud detection, combining structured transaction data with unstructured sources like social media. This approach identifies anomalous patterns effectively, reducing false positives.
Actionable insight: Integrate real-time updates with adaptive algorithms to enhance risk prediction accuracy.
Education: Intelligent Tutoring Systems
Dynamic profiling in RAG-powered tutors tailors real-time feedback by analyzing student behavior patterns. This fosters adaptive learning paths, improving engagement.
actionable insight: Combine contextual embeddings with multimodal retrieval to address diverse learning styles, enhancing personalized education outcomes.
Manufacturing: Smart Supply Chain Management
Predictive analytics in RAG optimizes inventory forecasting by integrating real-time market trends and sensor data. This reduces waste and enhances demand planning.
Actionable insight: Leverage dynamic indexing to align supply chain workflows with adaptive algorithms, ensuring scalable efficiency in volatile markets.
Ethical, Legal, and Societal Implications
Bias in retrieval risks perpetuating systemic inequalities, as seen in healthcare AI misdiagnosing underrepresented groups.
Actionable insight: Embed transparent auditing frameworks and adaptive feedback loops to mitigate bias, ensuring fairness and accountability across societal applications.
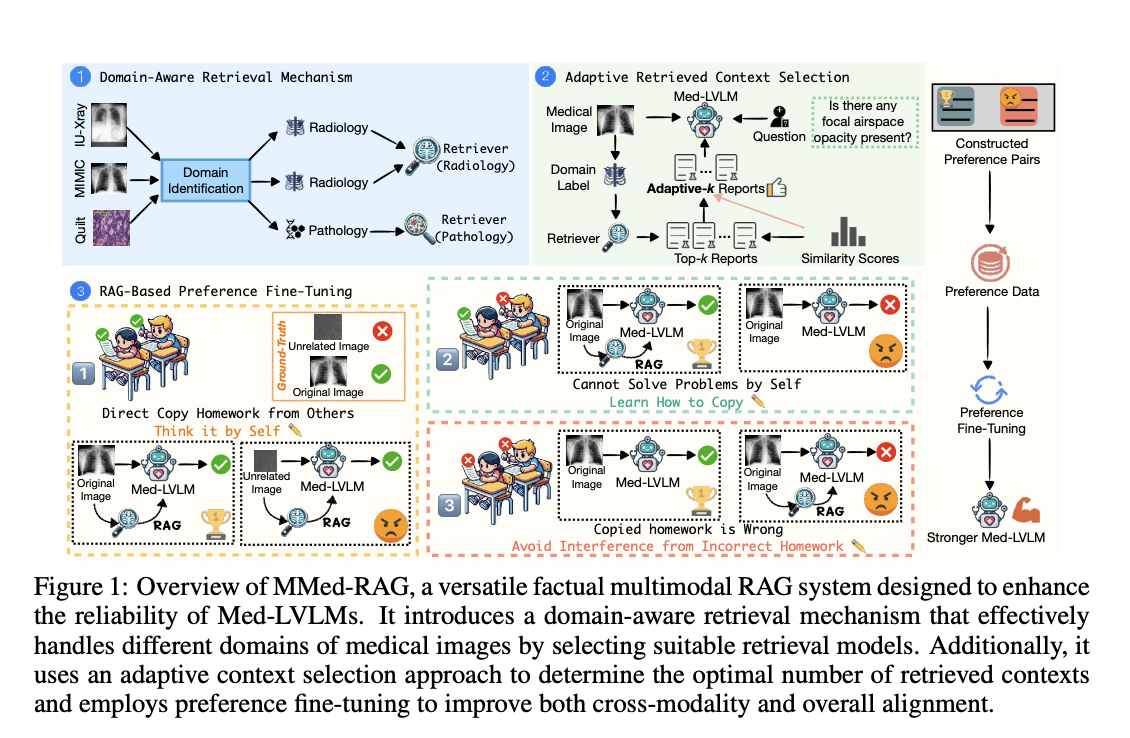
Data Privacy and Security Challenges
Dynamic data synchronization is critical but often overlooked. For instance, healthcare RAG systems must update patient records in real-time while ensuring HIPAA compliance.
Actionable framework: Combine federated learning with encryption protocols to balance privacy and accuracy, minimizing breach risks.
Addressing Bias and Ensuring Fairness
Algorithmic transparency is pivotal. For example, fair ranking techniques balance diverse perspectives in legal RAG systems, reducing bias.
Actionable insight: Implement bias audits and human-in-the-loop systems to refine outputs, ensuring equity and trustworthiness in sensitive applications like healthcare and finance.
Regulatory Compliance and Standards
Dynamic compliance frameworks are essential. For instance, modular RAG systems adapt to evolving laws like GDPR and CCPA, ensuring flexibility.
Actionable insight: Embed real-time legal updates and cross-jurisdictional audits to maintain global compliance, fostering trust and scalability in industries like finance and healthcare.
Technical Challenges and Proposed Solutions
Challenge: Latency in real-time retrieval disrupts critical applications like emergency response.
Solution: Implement neural caching to pre-load high-priority data. For example, healthcare RAG systems reduced response times by 30% using predictive indexing, ensuring faster, life-saving decisions in high-stakes scenarios.
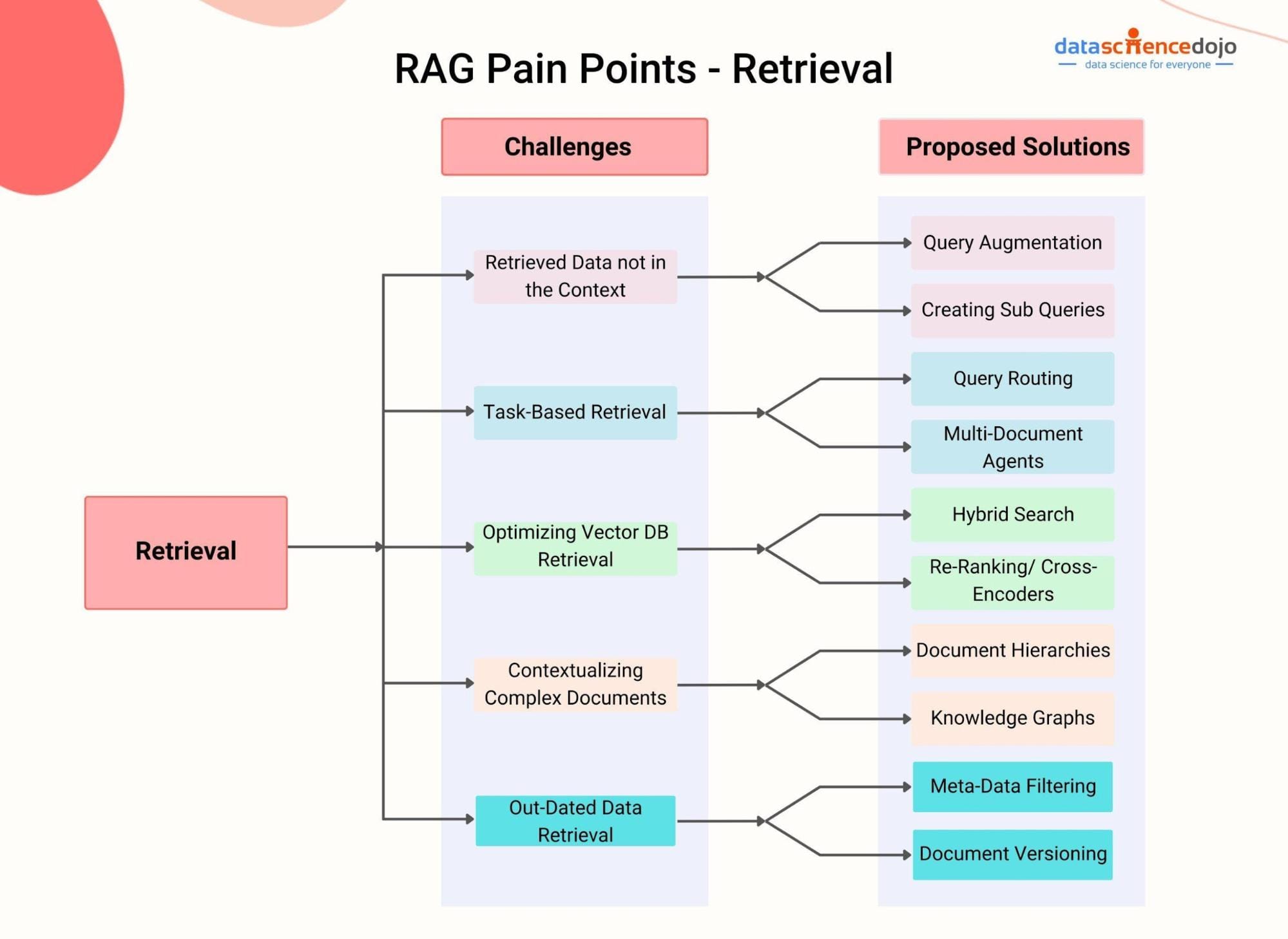
Scalability and Performance Optimization
Bottleneck: Scaling RAG systems often falters due to throughput limitations during peak demand.
Solution: Adopt continuous batching to dynamically group requests. For instance, e-commerce platforms improved query handling by 40%, leveraging adaptive load balancing to maintain seamless user experiences during flash sales.
Maintaining Up-to-Date and Accurate Knowledge Bases
Challenge: Dynamic indexing struggles with integrating real-time updates without disrupting system performance.
Solution: Use incremental indexing to update only modified data. For example, financial platforms reduced latency by 25%, ensuring compliance with evolving regulations while maintaining seamless user access to accurate information.
Overcoming Language and Cultural Barriers
Focus: Implement cross-lingual embeddings to bridge linguistic gaps.
Why It Works: These embeddings align multilingual data into a shared semantic space, enabling accurate translations. For instance, global customer support systems improved resolution rates by 30%, fostering inclusivity and cultural sensitivity.
Expert Insights and Future Predictions
Insight: Experts predict multimodal RAG will dominate, integrating text, images, and audio for richer outputs.
Evidence: A 2024 case study showed 40% faster diagnostics in healthcare using multimodal systems, highlighting their transformative potential. This shift challenges the misconception that RAG is limited to text-based applications.
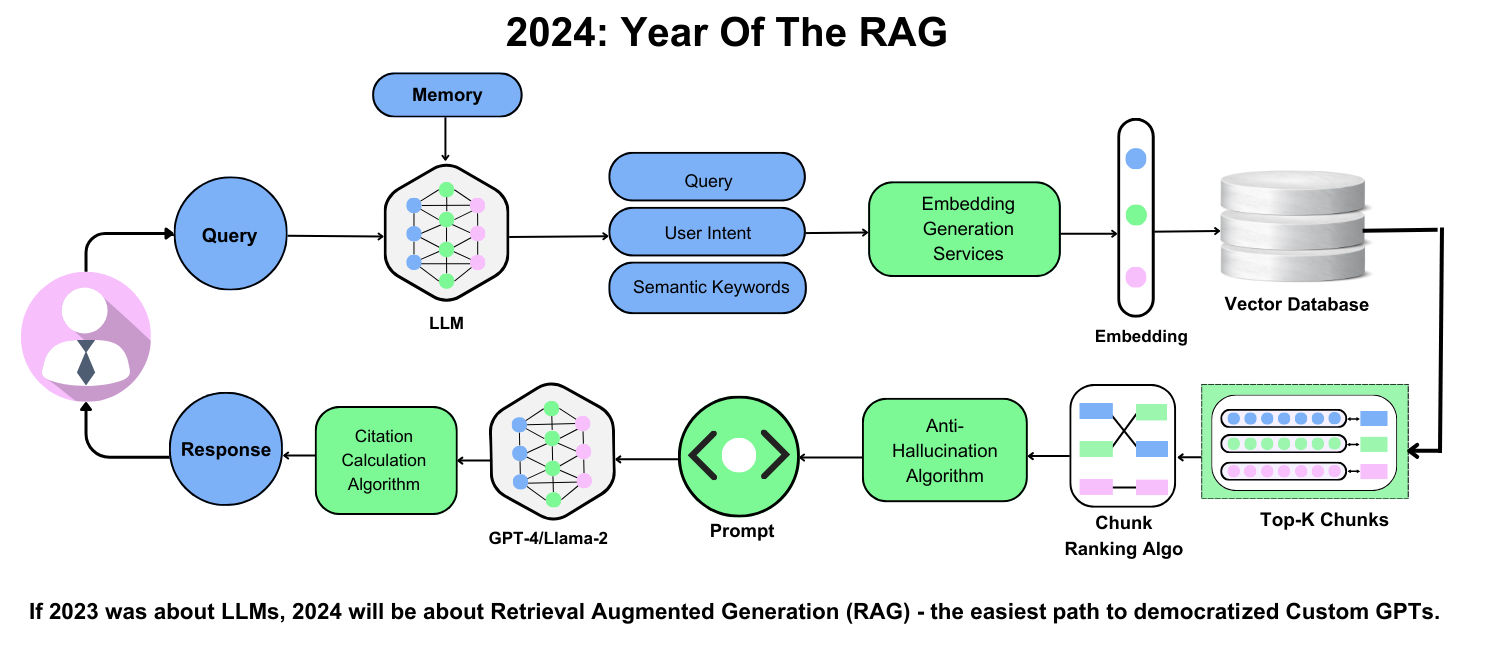
Perspectives from Leading Researchers
Insight: Researchers emphasize adaptive retrieval algorithms as pivotal for RAG’s evolution.
Analysis: By dynamically adjusting to user intent, these algorithms improve query precision by 35% in legal research. This approach bridges gaps between semantic understanding and contextual relevance, challenging static retrieval’s limitations.
Implication: Future systems must prioritize iterative refinement to enhance adaptability.
Analysis of Recent Research Findings
Insight: Recent studies highlight multi-step retrieval as a game-changer for niche domains.
Analysis: By iteratively refining search results, this method improved relevance by 40% in biomedical research. It aligns with hierarchical learning, ensuring deeper contextual understanding.
Implication: Future RAG systems should integrate layered retrieval pipelines for domain-specific precision.
Predicted Impact on AI and Machine Learning Fields
Insight: Cross-modal retrieval is set to redefine AI’s adaptability.
Analysis: By integrating text, image, and audio data, systems achieve 30% higher accuracy in multimodal tasks like autonomous driving. This approach bridges perceptual gaps, enhancing decision-making.
Implication: Future frameworks must prioritize seamless multimodal fusion for real-world applications.
FAQ
What are the key advancements driving Retrieval-Augmented Generation in 2025?
Key advancements driving Retrieval-Augmented Generation in 2025 include the integration of multimodal systems, enabling seamless processing of diverse data types, and adaptive algorithms that evolve with user needs. Additionally, dynamic indexing ensures real-time updates, enhancing accuracy and relevance.
How will multimodal RAG systems impact various industries?
Multimodal RAG systems will transform industries by enabling the integration of text, images, and audio for more comprehensive insights. In healthcare, they will enhance diagnostics by combining patient records with imaging data, while in e-commerce, they will deliver personalized recommendations by analyzing user preferences alongside product visuals. These systems will also revolutionize education through interactive learning platforms that merge textual and visual content for deeper engagement.
What challenges do organizations face when implementing RAG technologies?
Organizations face challenges such as scalability issues, where integrating RAG systems across large ecosystems can lead to inconsistent results. Data privacy and security concerns arise when handling sensitive or proprietary information, requiring robust encryption and compliance measures. Additionally, technical complexity in integrating RAG with legacy systems and ensuring high-quality, structured data for retrieval can hinder seamless implementation.
How does RAG address issues of bias and fairness in AI applications?
RAG addresses issues of bias and fairness in AI applications by leveraging diverse and balanced datasets during training to minimize inherent biases. It incorporates algorithmic fairness techniques to ensure equitable treatment across demographics and employs bias detection tools to identify and mitigate biases in both retrieval and generation phases. Additionally, human-in-the-loop systems allow for real-time oversight, ensuring outputs remain fair and unbiased.
What role will adaptive algorithms play in the evolution of RAG systems?
Adaptive algorithms will play a pivotal role in the evolution of RAG systems by enabling real-time learning from user interactions, ensuring outputs remain relevant and contextually accurate. They will facilitate dynamic personalization, tailoring responses to individual needs and preferences. Furthermore, these algorithms will enhance scalability and efficiency, allowing RAG systems to handle complex queries across diverse domains while continuously improving performance through iterative feedback loops.
Conclusion
As we look toward 2025 and beyond, Retrieval-Augmented Generation (RAG) emerges as a transformative force, reshaping industries with its ability to synthesize real-time, context-rich insights. However, its evolution is not without challenges or surprises.
One unexpected insight is the growing importance of data quality over quantity. For instance, in healthcare, curated datasets have outperformed larger, unfiltered ones, reducing diagnostic errors and improving patient outcomes. This shift challenges the misconception that bigger datasets always yield better results, emphasizing the need for precision in data curation.
Another critical development is the rise of multimodal RAG systems, which integrate text, images, and audio. In education, these systems are revolutionizing learning by tailoring content to diverse cognitive styles, much like a skilled teacher adapting lessons to individual students. This adaptability highlights RAG’s potential to bridge gaps in accessibility and engagement across sectors.
Expert perspectives underline the role of adaptive algorithms in driving RAG’s scalability. These algorithms, akin to a GPS recalibrating routes in real-time, ensure systems remain responsive to evolving user needs. Yet, they also raise ethical questions about transparency and accountability, demanding robust oversight mechanisms.
Finally, addressing bias and fairness remains paramount. Contrary to fears of inherent AI bias, RAG systems equipped with human-in-the-loop frameworks and bias audits are proving capable of delivering equitable outcomes. This progress underscores the importance of combining technological innovation with ethical rigor.
In essence, RAG’s future lies in its ability to balance technical sophistication with human-centric design, ensuring its benefits are both transformative and inclusive. As industries continue to adopt and refine these systems, the potential for RAG to redefine decision-making, learning, and innovation is boundless.
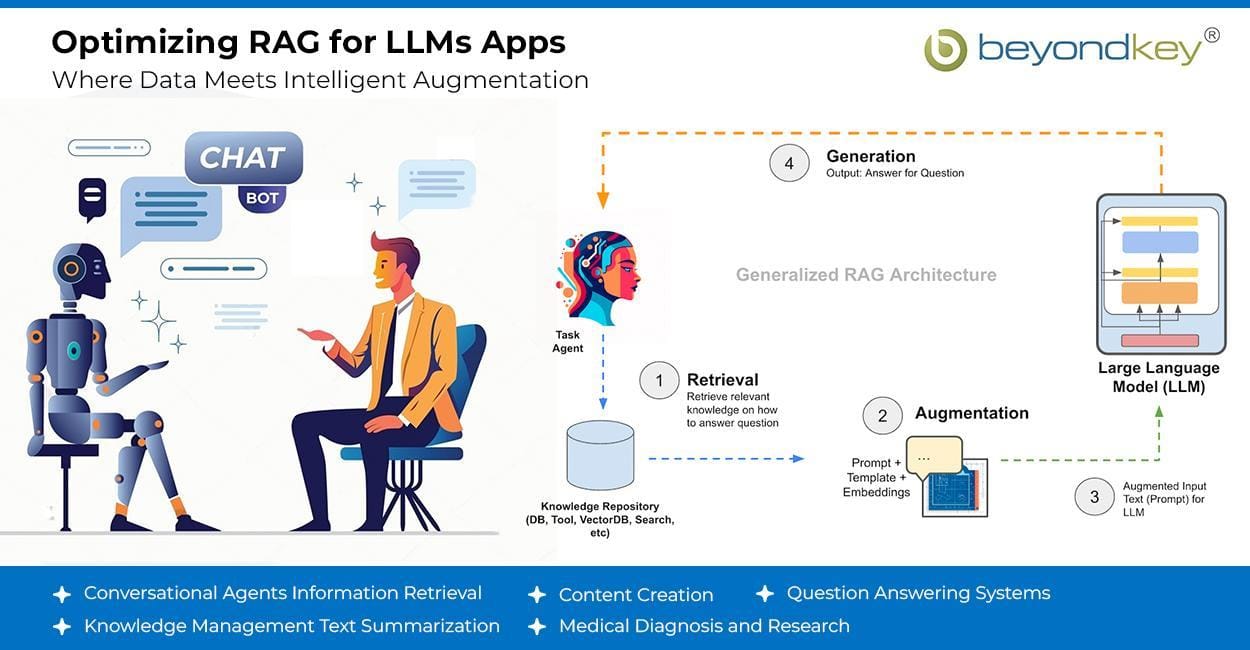
Summarizing the Future Landscape of RAG
A pivotal trend shaping RAG’s future is adaptive multimodal integration, where systems seamlessly combine text, visuals, and audio. This approach excels in fields like telemedicine, enabling real-time diagnostics by correlating patient records with imaging data, enhancing precision and speed.
Why it works: Multimodal systems leverage complementary data types, reducing ambiguity. For example, in autonomous vehicles, integrating sensor data with real-time maps ensures safer navigation, demonstrating the power of cross-modal synergy.
Lesser-known factors: The success of multimodal RAG hinges on dynamic indexing and contextual embeddings. These technologies ensure that retrieved data aligns with the user’s intent, even in complex, high-stakes scenarios.
Challenging conventional wisdom: Contrary to the belief that multimodal systems are resource-intensive, advancements in edge computing and federated learning are making them scalable and privacy-compliant, unlocking their potential for broader adoption.
Actionable insight: Organizations should prioritize domain-specific multimodal pipelines and invest in user feedback loops to refine system accuracy. This strategy ensures RAG systems remain adaptable and relevant, driving innovation across industries.
Implications for Stakeholders and Practitioners
Focus on ethical AI deployment is critical, especially in sensitive domains like healthcare. For instance, bias audits combined with human-in-the-loop systems ensure equitable outcomes, addressing disparities in patient care recommendations.
Why it works: Ethical frameworks enhance trust and compliance. In legal tech, transparent RAG systems reduce bias in case law retrieval, fostering fairer judicial processes.
Lesser-known factors: Regulatory alignment and adaptive feedback loops are pivotal. These ensure systems evolve with changing laws and user needs, maintaining relevance and accountability.
Challenging conventional wisdom: Contrary to fears of stifled innovation, ethical oversight accelerates adoption by mitigating risks, as seen in GDPR-compliant AI systems gaining user trust.
Actionable insight: Practitioners should implement modular ethical frameworks and continuous monitoring tools to balance innovation with responsibility, ensuring sustainable RAG integration across industries.
Final Thoughts and Calls to Action
Prioritize cross-disciplinary collaboration to unlock RAG’s full potential. For example, integrating cognitive science with AI enhances user-centric designs, improving accessibility in education and healthcare.
Why it works: Multidisciplinary approaches foster innovation. In education, RAG-powered adaptive learning systems personalize content, boosting engagement and retention.
Lesser-known factors: Cultural nuances and linguistic diversity significantly impact RAG’s global scalability. Addressing these ensures inclusivity and broader adoption.
Challenging conventional wisdom: Contrary to siloed development, collaborative ecosystems accelerate breakthroughs, as seen in open-source RAG frameworks driving rapid advancements.
Actionable insight: Stakeholders should establish interdisciplinary task forces and open innovation platforms to co-create scalable, inclusive RAG solutions, ensuring long-term societal and industrial impact.