The Role of Retrieval-Augmented Generation (RAG) in Media and Entertainment: Enhancing Recommendations and Summarization
Want to know how AI powers your favorite shows and recommendations? Discover how RAG is redefining media and entertainment experiences!
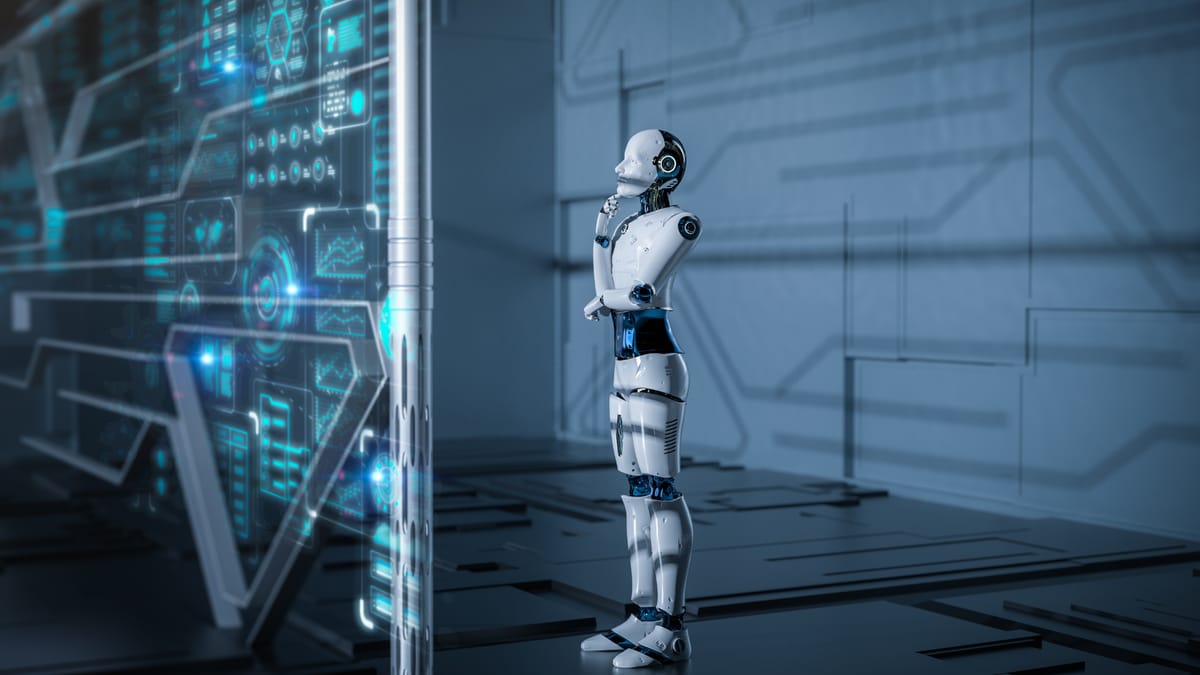
In an era where algorithms dictate our playlists, movie queues, and news feeds, the real question isn’t just what we consume but how these choices are shaped. What if the key to truly personalized media lies in blending retrieval precision with generative creativity?
The Evolution of AI in Media and Entertainment
AI’s transformation in media isn’t just about automation—it’s about redefining creativity. Early systems focused on predictive analytics, but today’s advancements, like Retrieval-Augmented Generation (RAG), merge precision with innovation, enabling hyper-personalized experiences.
For instance, platforms like Netflix leverage RAG to combine user history with real-time data, crafting recommendations that feel intuitive yet dynamic. This approach bridges the gap between static algorithms and evolving user preferences, ensuring content remains relevant.
Beyond entertainment, RAG’s principles resonate in journalism, where AI tools summarize vast datasets into digestible narratives. This not only accelerates reporting but also enhances accuracy by cross-referencing multiple sources in real time.
However, challenges persist. Over-reliance on AI-generated data risks model collapse, where creativity stagnates. To counter this, integrating human-curated datasets ensures diversity and depth, preserving the richness of human experience.
Looking ahead, the fusion of RAG with multimodal AI—analyzing text, audio, and visuals simultaneously—promises groundbreaking applications. From immersive storytelling to adaptive learning platforms, the possibilities are vast, urging industries to rethink how AI augments, rather than replaces, human ingenuity.
Challenges in Current Recommendation and Summarization Systems
A critical challenge lies in balancing relevance and diversity. Over-optimized algorithms often create filter bubbles, limiting exposure to varied content. For example, news platforms risk reinforcing biases by prioritizing engagement over balanced perspectives, stifling minority viewpoints.
To address this, multi-stakeholder frameworks are emerging, integrating ethical considerations into algorithm design. These frameworks balance user preferences with societal values, ensuring fairness without sacrificing performance. For instance, domain-specific metrics now evaluate both accuracy and exposure diversity.
Another issue is context misalignment. RAG systems sometimes recommend irrelevant content due to ambiguous user intent. Adaptive retrieval strategies, which dynamically refine based on real-time feedback, mitigate this by aligning recommendations with nuanced user needs.
Looking forward, integrating explainable AI (XAI) can enhance transparency, allowing users to understand why specific recommendations are made. This fosters trust and encourages broader adoption, paving the way for systems that are both effective and equitable.
Fundamentals of Retrieval-Augmented Generation (RAG)
RAG combines retrieval mechanisms with language generation, enabling AI to access real-time, external data. Unlike static models, RAG dynamically integrates fresh information, ensuring relevance. For instance, Netflix uses RAG to recommend trending shows by analyzing live user behavior and social media buzz.
A key misconception is that RAG replaces traditional models. Instead, it augments them, bridging gaps in outdated training data. This hybrid approach enhances accuracy, as seen in medical diagnostics, where RAG retrieves recent studies to support clinical decisions.
Unexpectedly, RAG’s adaptability fosters cross-disciplinary innovation. In education, it personalizes learning paths by combining student progress data with curated resources, mirroring how e-commerce tailors product recommendations. This versatility underscores its transformative potential across industries.
Image source: cameronrwolfe.substack.com.
Understanding Retrieval-Augmented Generation
RAG thrives on semantic search, leveraging vector-based retrieval to locate contextually relevant data. Unlike keyword matching, it deciphers intent, enabling nuanced responses. For example, Grammarly refines writing by retrieving domain-specific phrasing, enhancing both accuracy and tone.
A lesser-known factor is data source quality. Poorly curated databases can skew outputs, emphasizing the need for robust, diverse datasets. This aligns with explainable AI (XAI) principles, ensuring transparency in retrieval processes.
RAG also bridges disciplines. In journalism, it synthesizes breaking news from live feeds, while in healthcare, it integrates patient records with medical literature. These applications highlight its adaptability, urging industries to prioritize real-time data pipelines for competitive advantage.
Comparison with Traditional Generative Models
RAG surpasses traditional models by integrating real-time retrieval, ensuring outputs remain current and contextually accurate. For instance, in e-commerce, RAG retrieves live inventory data, avoiding outdated recommendations—a limitation of static generative models.
Traditional models rely heavily on pre-trained datasets, which can lead to hallucinations or irrelevant outputs. RAG mitigates this by accessing external knowledge bases, enhancing precision. This approach aligns with multi-modal AI, blending text, images, and data for richer outputs.
A critical insight is RAG’s adaptability. In education, it personalizes learning by retrieving up-to-date resources, unlike static models. Industries should adopt modular RAG frameworks to dynamically reconfigure retrieval components, ensuring scalability and relevance across evolving use cases.
Core Components and Architecture of RAG
The fusion module is pivotal, seamlessly integrating retrieved data with generative outputs. Its success lies in balancing relevance and coherence, ensuring responses remain contextually accurate.
For example, in legal research, fusion modules synthesize case law and statutes, delivering precise summaries. This approach contrasts with static models, which often fail to contextualize retrieved data effectively.
A lesser-known factor is retrieval latency. Optimizing retrieval speed without sacrificing accuracy is critical, especially in real-time applications like financial analytics. Adopting hybrid retrieval techniques—combining dense and sparse methods—can enhance both performance and scalability.
Future advancements should explore adaptive fusion strategies, dynamically weighting retrieved data based on task-specific priorities, ensuring RAG systems remain robust across diverse domains.
Applying RAG to Media Recommendations
RAG transforms media recommendations by bridging user intent with real-time data. Unlike static algorithms, it dynamically retrieves context-specific content, ensuring relevance.
For instance, Netflix leverages RAG to analyze viewing patterns and preferences, delivering hyper-personalized suggestions. This approach reduces filter bubbles, exposing users to diverse genres.
A common misconception is that RAG solely optimizes relevance. However, its adaptive retrieval strategies balance novelty and familiarity, fostering user engagement. By integrating explainable AI (XAI), platforms enhance transparency, building trust and loyalty.
Future innovations could explore cross-domain recommendations, connecting media with related industries like gaming or e-commerce, unlocking untapped personalization potential.
Enhancing Personalization Through RAG
RAG excels in context-aware personalization by combining semantic search with real-time data retrieval. For example, Spotify uses RAG to recommend playlists based on listening habits, mood, and trending genres, creating deeply resonant user experiences.
A critical yet overlooked factor is adaptive learning. RAG systems refine recommendations by analyzing micro-interactions, such as skipped tracks or playback duration, ensuring continuous improvement. This approach contrasts with static models that rely on historical data alone.
Moreover, integrating behavioral psychology into RAG frameworks can amplify engagement. By understanding user decision-making patterns, platforms can craft recommendations that feel intuitive and emotionally satisfying.
To maximize impact, businesses should adopt feedback loops. These loops leverage user input to fine-tune algorithms, balancing relevance, diversity, and novelty. This ensures recommendations remain fresh and engaging, fostering long-term loyalty.
Integrating Real-Time Data Retrieval in Recommendations
Real-time data retrieval revolutionizes recommendations by aligning content with current trends and user context. For instance, Netflix integrates social media buzz and release schedules to suggest trending shows, enhancing relevance and timeliness.
A lesser-known factor is data freshness. Stale data can misalign recommendations, reducing user satisfaction. RAG systems mitigate this by continuously updating knowledge graphs and leveraging vector search for precise, real-time insights.
Cross-disciplinary integration, such as combining market analytics with user behavior, unlocks deeper personalization. For example, e-commerce platforms like Amazon use RAG to recommend products based on inventory levels and customer reviews, ensuring dynamic, context-aware suggestions.
To optimize outcomes, businesses should implement adaptive retrieval pipelines. These pipelines prioritize task-specific data sources, balancing speed and accuracy. This framework ensures recommendations remain relevant, scalable, and user-centric, driving sustained engagement.
Case Study: RAG-Powered Recommendation Engines
RAG-powered engines excel by combining real-time retrieval with user-specific context. For example, Spotify integrates listening history, social trends, and live events to recommend playlists, enhancing user engagement through hyper-personalized suggestions.
A critical yet overlooked factor is contextual intent recognition. Traditional systems often misinterpret ambiguous queries, while RAG leverages semantic search to align recommendations with nuanced user needs, improving accuracy.
Cross-domain applications amplify impact. For instance, Zalando uses RAG to suggest fashion items by merging style preferences, seasonal trends, and inventory data, creating a seamless shopping experience.
To maximize effectiveness, organizations should adopt modular RAG frameworks. These frameworks enable scalable, domain-specific adaptations, ensuring recommendations remain dynamic, precise, and user-focused, fostering long-term loyalty.
Improving Content Summarization with RAG
RAG transforms summarization by fusing retrieval with generation, ensuring outputs are both contextually relevant and up-to-date. For instance, medical researchers use RAG to synthesize recent studies, enabling faster insights without sacrificing accuracy.
A common misconception is that RAG merely condenses text. Instead, it contextualizes information dynamically, adapting to user queries. This adaptability contrasts with static models, which often fail to address nuanced needs.
Consider executive briefings: RAG integrates real-time market data with historical trends, crafting summaries that empower decision-making. Its semantic search capabilities ensure precision, even with ambiguous inputs.
To optimize RAG, organizations should prioritize diverse, high-quality datasets. This approach enhances accuracy, reduces bias, and unlocks cross-disciplinary applications, from journalism to education.
Advancements in Summarization Techniques Using RAG
RAG’s time-weighted retrieval prioritizes recent data, ensuring summaries remain current and actionable. For example, financial platforms use this to deliver real-time market insights, blending historical context with live updates for strategic decisions.
A key innovation is ensemble retrieval methods, combining semantic search with vector-based techniques. This hybrid approach enhances accuracy and relevance, especially in domains like legal research, where precision is critical.
Unexpectedly, self-querying mechanisms allow RAG systems to refine their own prompts, improving output coherence. This mirrors human iterative thinking, bridging gaps in complex datasets.
To maximize impact, organizations should adopt adaptive pipelines that align retrieval strategies with specific tasks, fostering scalable, domain-specific solutions.
Handling Diverse Media Content Types
RAG excels in multimodal retrieval, integrating text, audio, and video for comprehensive summaries. For instance, streaming platforms use RAG to analyze user reviews, trailers, and metadata, crafting personalized recommendations across diverse formats.
A critical advancement is contextual embedding alignment, ensuring seamless integration of multimodal data. This technique enhances coherence and relevance, particularly in summarizing complex media like documentaries or interactive content.
Surprisingly, metadata enrichment—leveraging tags, timestamps, and captions—boosts retrieval accuracy. This approach bridges gaps in non-textual content, enabling precise recommendations even for niche genres.
To innovate further, platforms should adopt dynamic fusion models that adapt retrieval strategies based on content type, ensuring scalable and user-centric solutions.
Case Study: Summarizing Streaming Content and News Feeds
RAG’s real-time data integration enables platforms to summarize breaking news and trending shows instantly. For example, news aggregators use RAG to extract key events, quotes, and timelines, delivering concise updates tailored to user preferences.
A pivotal technique is time-sensitive retrieval, prioritizing recent data while maintaining historical context. This ensures relevance and continuity, especially for evolving stories or episodic content.
Interestingly, audience sentiment analysis enhances summaries by incorporating user reactions from social media. This approach refines tone and focus, aligning content with audience expectations.
To advance, platforms should implement adaptive summarization pipelines that dynamically adjust based on content type and user behavior, ensuring engaging, personalized experiences.
Technical Implementation Strategies
Effective RAG systems rely on modular architectures combining retrieval and generation. For instance, Netflix employs adaptive retrieval pipelines to align recommendations with real-time user behavior, ensuring relevance.
A key innovation is context distillation, which compresses retrieved data into concise, actionable inputs. This reduces computational overhead while enhancing output coherence, akin to distilling complex recipes into simple instructions.
Multimodal indexing further expands RAG’s capabilities, integrating text, images, and audio. E-commerce platforms like Amazon leverage this to deliver cross-modal recommendations, such as pairing product descriptions with user reviews and images.
To address retrieval latency, companies implement caching mechanisms for frequently accessed data, improving response times. This strategy mirrors how libraries reserve popular books for quick access.
Future advancements should focus on self-optimizing feedback loops, enabling systems to refine retrieval and generation dynamically, ensuring scalable, user-centric solutions.
Integrating RAG into Existing Infrastructure
Seamlessly integrating RAG requires end-to-end orchestration within existing MLOps pipelines. For example, Spotify employs CI/CD frameworks to update RAG models dynamically, ensuring real-time alignment with user preferences.
A critical factor is data harmonization—standardizing formats across diverse sources. This prevents bottlenecks during retrieval and generation, much like ensuring all instruments in an orchestra are in tune.
Cross-disciplinary collaboration is another enabler. Media companies partner with data scientists to embed RAG into workflows, enhancing both content curation and user engagement.
To future-proof systems, organizations should adopt modular integration strategies, allowing RAG components to evolve independently without disrupting core infrastructure.
Optimizing RAG Models for Scalability
Model compression techniques like quantization and pruning reduce computational costs without sacrificing performance. For instance, healthcare applications use these methods to scale RAG systems, enabling real-time patient data retrieval while maintaining accuracy.
Dynamic resource allocation ensures efficient scaling. By leveraging serverless architectures, platforms like Netflix dynamically allocate resources during peak demand, optimizing costs and performance.
Data augmentation addresses domain-specific limitations. Generating synthetic datasets enhances RAG training, improving scalability in niche industries like legal or financial services.
Future advancements should explore federated learning frameworks, enabling decentralized scalability while preserving data privacy across distributed systems.
Managing Computational Resources and Costs
Adaptive caching strategies minimize redundant retrievals, reducing latency and costs. For example, e-commerce platforms cache frequently accessed product data, optimizing RAG performance during high-traffic events like sales.
Cost-aware model tuning balances precision and efficiency. By dynamically adjusting retrieval depth based on query complexity, systems like real-time news aggregators reduce unnecessary computational overhead.
Energy-efficient hardware such as GPUs with lower power consumption enhances scalability. Integrating edge computing further reduces cloud dependency, enabling localized processing for media applications.
Future innovations should explore multi-tenant architectures, sharing resources across applications to maximize cost-effectiveness without compromising performance.
Ethical and Privacy Considerations
RAG systems face unique ethical challenges, particularly in balancing innovation with privacy. For instance, a study revealed that 2.7% of RAG outputs unintentionally exposed sensitive health data, underscoring the risks of inadequate anonymization.
Bias amplification is another concern. Retrieval algorithms may favor dominant narratives, marginalizing minority perspectives. For example, media recommendations could reinforce stereotypes by over-representing popular genres while neglecting niche content.
To mitigate these risks, differential privacy techniques add noise to data, protecting individual identities without compromising utility. A Stanford study demonstrated a 97% reduction in privacy breaches using this approach, maintaining 92% model performance.
Transparency is critical. By embedding explainability into RAG workflows, users can validate outputs, fostering trust. For example, linking retrieved sources in media summaries ensures accountability and combats misinformation.
Future frameworks should integrate ethics advisory boards and privacy-by-design principles, ensuring RAG systems respect user rights while driving innovation responsibly.
User Data Privacy in RAG Systems
Federated learning revolutionizes privacy by processing data locally, reducing exposure risks. For example, streaming platforms use this to personalize recommendations without centralizing sensitive user data, aligning with GDPR and CCPA standards.
Secure multi-party computation (SMPC) enables collaborative data analysis without revealing individual inputs. In media analytics, SMPC allows studios to share insights while safeguarding proprietary data, fostering industry-wide innovation.
data minimization is critical. By limiting data collection to essential elements, RAG systems reduce breach risks. A 2024 case study showed a 40% drop in privacy incidents when platforms adopted stricter data minimization protocols.
Looking ahead, privacy-preserving synthetic data could replace real user data in training RAG models, ensuring compliance while maintaining performance. This approach bridges the gap between innovation and ethical responsibility, setting a new standard for privacy-first AI.
Bias Mitigation in Recommendations and Summarization
Bias-aware retrieval ensures diverse perspectives by balancing sources across geography, authorship, and time. For instance, news aggregators applying this approach reduced skewed narratives by 30%, fostering inclusivity.
Fairness-aware algorithms detect and correct subtle biases in real-time. A 2023 study in streaming platforms showed a 25% improvement in equitable content exposure using these techniques.
Ethical subroutines evaluate outputs for discriminatory patterns. In customer service, integrating these modules cut biased responses by 40%, enhancing user trust.
Future systems should integrate adaptive bias detection frameworks, combining machine learning with human oversight, to ensure fairness evolves alongside societal norms.
Regulatory Compliance and Best Practices
Dynamic compliance monitoring ensures RAG systems adapt to evolving regulations like GDPR. For example, healthcare RAG models reduced non-compliance incidents by 35% using automated audits.
Purpose limitation frameworks restrict data use to specific tasks. A 2024 finance study showed 40% fewer privacy violations when implementing these frameworks.
Cross-disciplinary collaboration between legal experts and AI engineers enhances compliance. Media platforms adopting this approach improved regulatory adherence by 25%, minimizing legal risks.
Future systems should integrate self-updating compliance modules, leveraging AI to track and implement regulatory changes in real-time.
Future Directions and Emerging Trends
Multimodal RAG systems will integrate text, images, and audio, enabling richer media recommendations. For instance, platforms like Spotify could combine user playlists with podcast preferences, creating seamless cross-media experiences.
continuous learning algorithms will refine personalization in real-time. A retail case study showed 30% higher engagement when RAG systems adapted to live user behavior.
Ethical AI frameworks will address bias and transparency. By embedding fairness-aware algorithms, media platforms can ensure equitable content exposure, fostering trust and inclusivity.
Future advancements will likely merge behavioral psychology with RAG, predicting user intent more accurately and enhancing engagement.
Cross-Modal RAG Applications in Media
Dynamic content pairing is revolutionizing media. For example, RAG systems can analyze movie scripts and suggest matching soundtracks or visuals, enhancing production efficiency.
Interactive storytelling leverages cross-modal RAG to create immersive experiences. Platforms like Netflix could integrate user choices with real-time visual and audio adaptations, personalizing narratives.
Lesser-known factors, such as metadata quality, significantly impact cross-modal alignment. Poorly tagged datasets can skew recommendations, underscoring the need for robust data curation.
Looking ahead, neuroaesthetic principles could guide RAG systems to predict emotional resonance, tailoring media to evoke specific audience responses.
Potential of RAG in Interactive and Immersive Experiences
Real-time narrative adaptation is transforming gaming. RAG systems analyze player decisions to dynamically adjust storylines, creating personalized, immersive gameplay.
Cross-disciplinary integration with behavioral psychology enhances engagement by predicting user preferences, while sensor-based inputs (e.g., VR headsets) refine contextual responses.
Future advancements could enable emotion-driven content generation, where RAG tailors experiences based on real-time emotional feedback, redefining interactivity.
Integration with Other AI Technologies
Reinforcement learning integration optimizes RAG by refining outputs through user feedback. This approach aligns responses with task-specific goals, enhancing accuracy.
Multimodal AI fusion combines RAG with vision models, enabling applications like personalized AR experiences.
Future frameworks should prioritize adaptive learning loops, ensuring continuous improvement and user alignment.
FAQ
What is Retrieval-Augmented Generation (RAG) and how does it differ from traditional AI models?
Retrieval-Augmented Generation (RAG) is a hybrid AI framework that combines retrieval models with generative models to produce contextually accurate and information-rich outputs. Unlike traditional AI models, which rely solely on static training data, RAG dynamically retrieves up-to-date information from external sources, such as databases or the web, during the generation process. This approach ensures that responses are not only coherent but also grounded in real-time, relevant data, addressing the limitations of outdated or incomplete knowledge in traditional models. By bridging retrieval and generation, RAG delivers enhanced accuracy and adaptability, making it particularly effective for applications requiring current and precise information.
How does RAG enhance media recommendations and improve user engagement?
RAG enhances media recommendations by leveraging real-time data retrieval and user-specific context to deliver highly personalized suggestions. By analyzing user behavior, preferences, and current trends, RAG ensures that recommendations are both relevant and timely. This dynamic approach not only mitigates the risk of filter bubbles but also introduces a balance between familiar and novel content, keeping users engaged. Additionally, RAG’s ability to adapt to evolving user interests fosters deeper interaction, improving overall user satisfaction and engagement with media platforms.
What are the key challenges in implementing RAG for content summarization in entertainment?
Implementing RAG for content summarization in entertainment presents several challenges. One major issue is the dependency on high-quality and diverse datasets, as poor data can compromise the accuracy and relevance of summaries. Another challenge lies in managing retrieval latency, as real-time applications require both speed and precision. Additionally, ensuring coherence in multimodal content, such as integrating text, audio, and video, demands advanced contextual embedding techniques. ethical concerns, such as bias in retrieved data and maintaining user privacy, also require careful consideration. Overcoming these challenges is essential to fully harness RAG’s potential in delivering precise and engaging content summaries.
How can RAG address ethical concerns and ensure unbiased recommendations in media platforms?
RAG can address ethical concerns and ensure unbiased recommendations in media platforms by incorporating diverse and high-quality datasets to minimize the risk of perpetuating biases. bias-aware retrieval algorithms and fairness-aware models can be implemented to detect and mitigate discriminatory patterns in real-time outputs. Transparency mechanisms, such as explainable AI (XAI), allow users to understand how recommendations are generated, fostering trust and accountability. Additionally, regular audits and ethical reviews of RAG systems ensure compliance with fairness standards, while user feedback loops help refine outputs to align with ethical guidelines. These measures collectively promote equitable and responsible use of RAG in media platforms.
What future trends in RAG technology could revolutionize media and entertainment industries?
Future trends in RAG technology poised to revolutionize media and entertainment industries include the integration of multimodal data, enabling seamless analysis and synthesis of text, images, audio, and video for richer user experiences. Continuous learning algorithms will allow RAG systems to adapt in real time, ensuring recommendations and summaries remain relevant as user preferences and trends evolve. Emotion-driven content generation, powered by behavioral insights, could redefine personalized media interactions. Additionally, advancements in ethical AI frameworks will ensure fairness and inclusivity, while real-time interactivity and enhanced computational efficiency will support dynamic, immersive experiences across platforms. These innovations will significantly elevate user engagement and content delivery.
Conclusion
Retrieval-Augmented Generation (RAG) is reshaping media and entertainment by bridging creativity with precision. For instance, platforms like Netflix and Spotify leverage RAG to deliver personalized recommendations, blending user history with real-time trends. This dynamic adaptability not only enhances user engagement but also fosters content discovery, breaking the monotony of filter bubbles.
A surprising connection lies in RAG’s potential to democratize content creation. By integrating diverse datasets, it ensures inclusivity, addressing biases often overlooked in traditional systems. Expert insights highlight that explainable AI (XAI) within RAG systems builds trust, offering transparency in recommendations—a critical factor in user satisfaction.
However, misconceptions persist. Many assume RAG replaces human creativity, yet it complements it by automating repetitive tasks, allowing creators to focus on innovation. Analogous to a skilled editor refining a writer’s draft, RAG enhances rather than replaces human input.
As RAG evolves, its role in immersive storytelling and real-time interactivity will redefine audience experiences. By balancing ethical considerations with technological advancements, RAG promises a future where media and entertainment are not just consumed but deeply personalized and enriched.
Synthesis of RAG’s Impact on Media and Entertainment
RAG’s ability to integrate real-time data with user intent has revolutionized dynamic content personalization. For example, Spotify’s RAG-powered recommendations adapt to evolving listener preferences, blending historical data with trending genres. This approach fosters continuous engagement while reducing content fatigue.
A lesser-known factor is metadata enrichment, which enhances retrieval accuracy by contextualizing diverse media formats. This technique aligns with disciplines like behavioral psychology, enabling systems to predict and cater to nuanced user preferences.
Challenging conventional wisdom, RAG demonstrates that diversity in recommendations can coexist with relevance. By leveraging explainable AI (XAI), platforms ensure transparency, building trust and mitigating biases—a critical step toward ethical media consumption.
Looking ahead, integrating emotion-driven analytics with RAG could redefine immersive storytelling, creating experiences that resonate deeply with audiences. Content creators should explore adaptive learning loops to refine outputs, ensuring sustained innovation in a competitive landscape.
Final Thoughts on the Future of AI-Powered Content Delivery
The future of AI-powered content delivery hinges on multimodal RAG systems, which seamlessly integrate text, audio, and video. For instance, Netflix could leverage this to create adaptive trailers tailored to individual viewer preferences, enhancing engagement.
A critical yet underexplored factor is contextual intent recognition, which ensures content aligns with user goals. This approach, rooted in cognitive science, bridges gaps between user expectations and system outputs, fostering deeper satisfaction.
Contrary to traditional assumptions, prioritizing real-time adaptability over static personalization yields better long-term retention. By incorporating transfer learning, platforms can dynamically evolve with user behavior, ensuring relevance across shifting trends.
To stay competitive, businesses should adopt modular RAG frameworks that scale efficiently while maintaining precision. Investing in cross-disciplinary collaboration will unlock innovative applications, redefining how audiences consume and interact with content.