How Retrieval-Augmented Generation Transforms Your SEO Strategy
Learn how Retrieval-Augmented Generation is reshaping SEO in 2025. Unlock new strategies to boost your rankings and stay ahead of the competition!
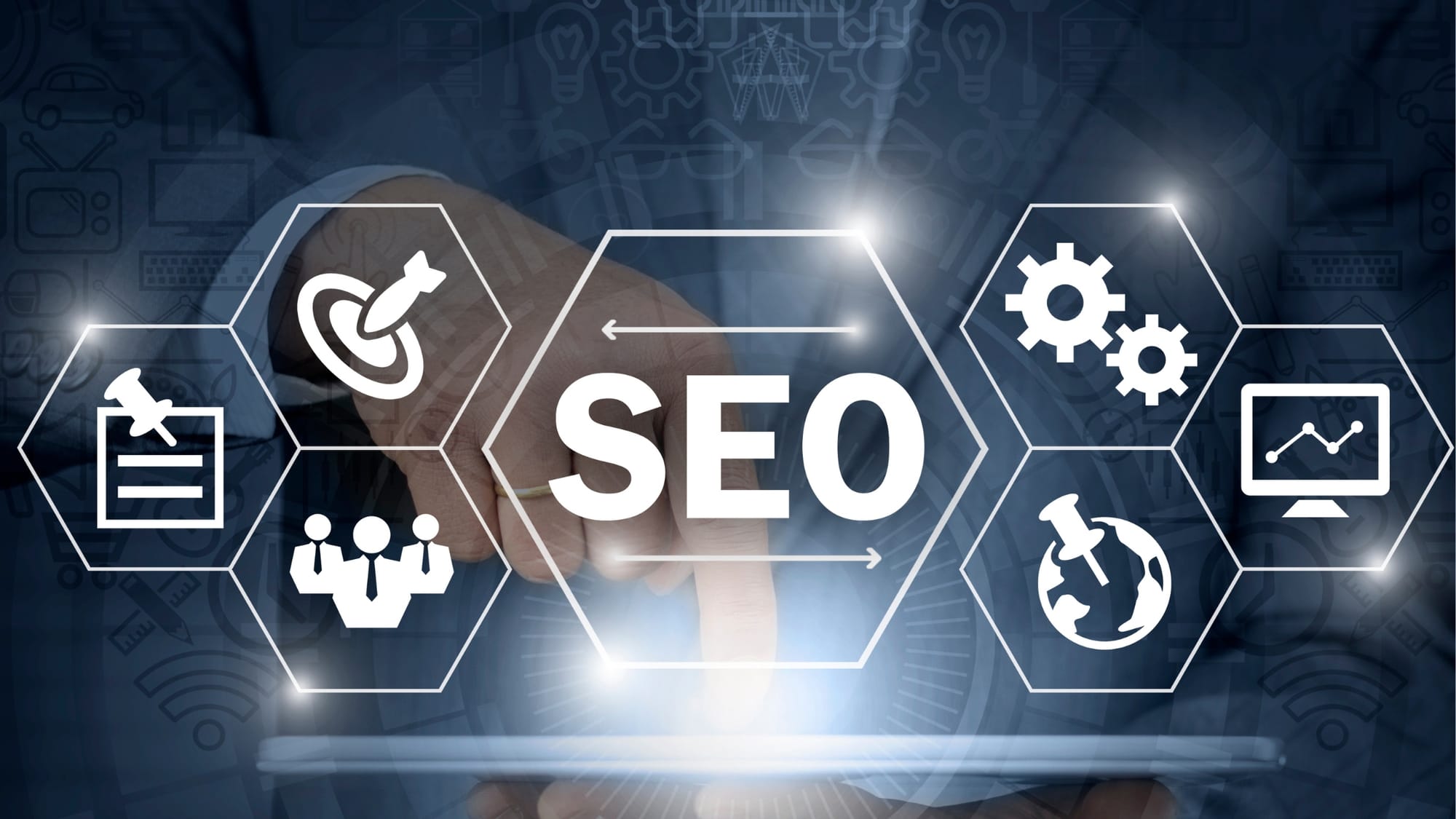
Imagine a world where search engines no longer prioritize keywords but instead reward precision, context, and relevance. As AI reshapes SEO, are you prepared to harness Retrieval-Augmented Generation to stay ahead?
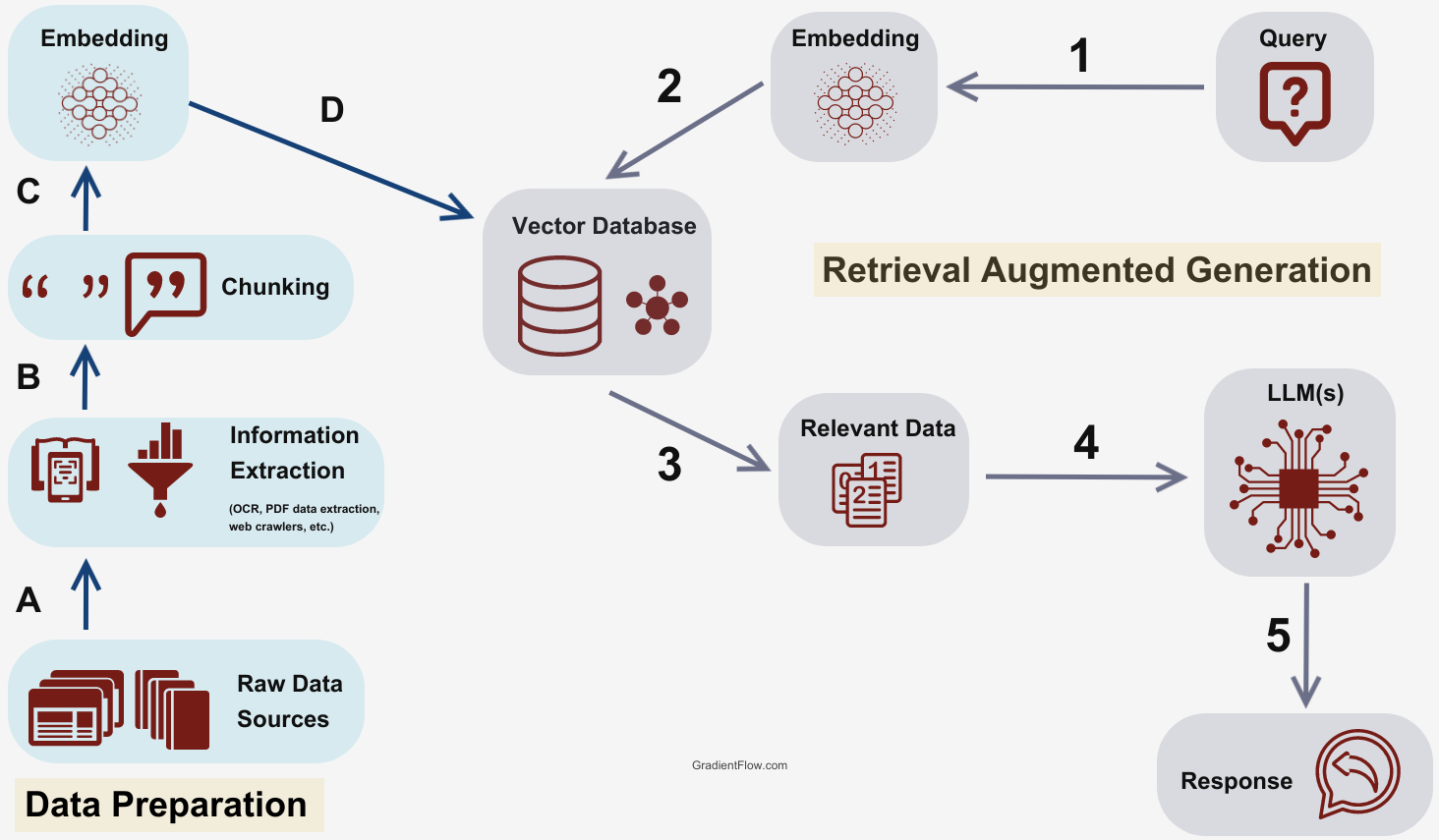
The Evolution of SEO in the Age of AI
AI-driven algorithms like Google BERT and MUM have redefined SEO by prioritizing contextual understanding over keyword density. This shift demands content that aligns with user intent, offering nuanced, actionable insights.
For instance, voice search optimization has gained prominence as conversational queries dominate. Adapting content to mirror natural language patterns ensures higher relevance in AI-powered search results, especially for virtual assistants like Alexa or Siri.
Moreover, predictive analytics now play a pivotal role. By analyzing user behavior, AI anticipates search trends, enabling businesses to craft proactive strategies. Tools like SEMrush leverage this to refine keyword targeting dynamically.
A lesser-known factor is the impact of multimodal search. AI integrates text, images, and videos, requiring content creators to diversify formats. For example, optimizing alt-text and metadata for visual content enhances discoverability.
To thrive, adopt a user-first approach: focus on delivering value through comprehensive, well-structured content. Embrace AI tools to automate repetitive tasks, freeing time for strategic innovation. This evolution is not just technical—it’s transformative.
Introducing Retrieval-Augmented Generation (RAG)
RAG revolutionizes SEO by combining real-time retrieval with generative AI, ensuring content remains accurate and contextually relevant. Unlike static models, RAG dynamically integrates external data, addressing challenges like outdated information and hallucinations.
For example, customer support chatbots powered by RAG access updated knowledge bases, delivering precise answers without retraining. Similarly, legal research tools synthesize complex statutes, enhancing efficiency and accuracy in decision-making.
A critical yet overlooked factor is vector embeddings. These enable semantic retrieval, ensuring nuanced understanding across multimodal data like text and images. This capability is transformative for industries like healthcare, where precision is paramount.
To implement RAG effectively, invest in modular architectures for scalability and robust data governance frameworks. This ensures ethical AI practices while maximizing performance. As RAG evolves, its integration with predictive analytics will redefine SEO strategies.
Understanding Retrieval-Augmented Generation
Retrieval-Augmented Generation (RAG) bridges static AI limitations by integrating real-time data retrieval with generative capabilities. Think of it as a library assistant—fetching precise, relevant information before crafting responses.
For instance, e-commerce platforms use RAG to recommend products by analyzing live inventory and user preferences. This dynamic approach outperforms traditional AI, which relies on pre-trained, often outdated models.
A common misconception is that RAG merely enhances accuracy. In reality, it reshapes workflows, enabling personalized content creation and adaptive SEO strategies. For example, travel websites leverage RAG to generate destination guides tailored to seasonal trends.
Expert insights emphasize data quality as critical. Poorly curated sources can lead to irrelevant outputs, undermining user trust. Thus, filtering high-quality data and fine-tuning models are essential for success.
Unexpectedly, RAG’s semantic search capabilities also improve multimodal content retrieval, such as pairing text with images. This innovation is pivotal for industries like healthcare, where precision and context are non-negotiable.
By addressing these nuances, RAG not only transforms SEO but also redefines content personalization, offering businesses a competitive edge in an ever-evolving digital landscape.
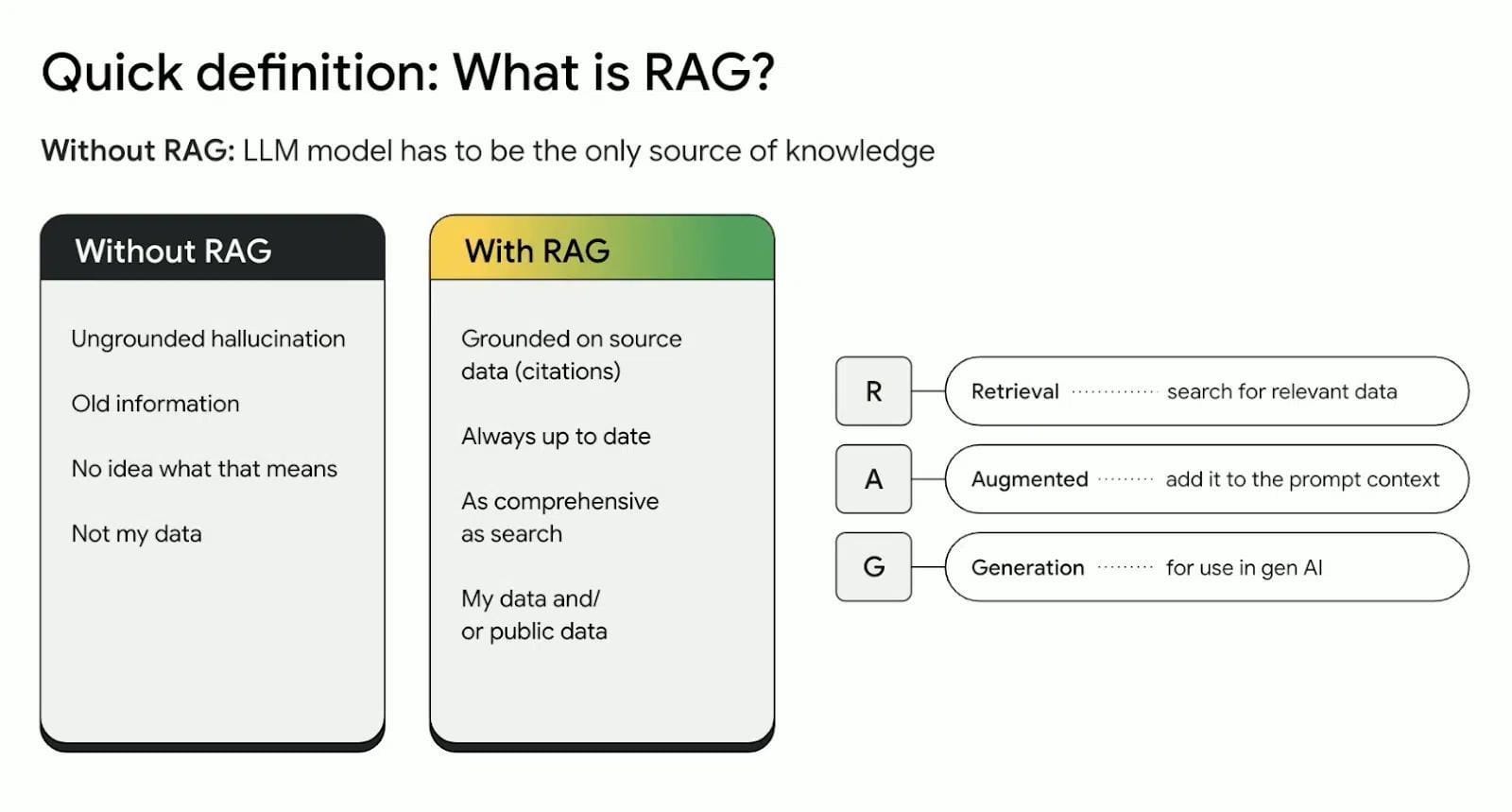
Defining RAG: Merging Retrieval and Generation
RAG’s core innovation lies in its ability to combine retrieval precision with generative creativity, creating outputs grounded in real-world data. This duality ensures relevance while maintaining adaptability across diverse applications.
For example, legal research tools powered by RAG retrieve case law and generate concise summaries, saving hours of manual work. Similarly, marketing platforms use RAG to craft personalized campaigns by analyzing user behavior in real time.
A lesser-known factor is vector embeddings, which enable semantic understanding of queries. This approach bridges gaps between structured and unstructured data, making RAG indispensable for industries like healthcare and finance.
Conventional wisdom suggests generative models excel in creativity but lack factual grounding. RAG challenges this by integrating real-time retrieval, ensuring outputs are both accurate and contextually rich.
To maximize RAG’s potential, businesses should focus on:
- Data governance: Ensure high-quality, unbiased sources.
- Modular architectures: Enable seamless updates and scalability.
- Ethical compliance: Address privacy concerns in sensitive domains.
By merging retrieval and generation, RAG not only enhances SEO strategies but also redefines how AI interacts with dynamic, real-world data.
How RAG Differs from Traditional NLP Models
Traditional NLP models rely on static training data, limiting adaptability. RAG introduces real-time retrieval, enabling dynamic responses grounded in current, external knowledge—a game-changer for industries requiring up-to-date accuracy.
For instance, e-commerce platforms leverage RAG to recommend products based on live inventory data, unlike static models that risk outdated suggestions. Similarly, news aggregators use RAG to deliver contextually relevant updates, enhancing user engagement.
A critical distinction is RAG’s non-parametric memory, which bypasses the limitations of parametric memory in traditional models. This allows RAG to scale knowledge without retraining, reducing computational costs and improving efficiency.
Conventional models often struggle with biases from static datasets. RAG mitigates this by integrating diverse, real-time sources, ensuring outputs are both balanced and contextually accurate.
To harness RAG effectively:
- Prioritize source quality: Ensure reliable, unbiased data.
- Optimize retrieval modules: Use dense vector embeddings for precision.
- Invest in modular systems: Enable seamless updates and scalability.
RAG’s dynamic adaptability positions it as a transformative tool, bridging the gap between static NLP and real-world demands.
Core Components of RAG Systems
dense vector embeddings revolutionize retrieval by enabling semantic understanding of queries, surpassing keyword matching. This approach excels in multimodal data retrieval, crucial for industries like healthcare where precision and context are paramount.
For example, medical diagnostics systems use dense embeddings to retrieve relevant case studies or research, ensuring accurate recommendations. Similarly, legal platforms leverage this to extract contextually aligned precedents, streamlining case analysis.
A lesser-known factor is hybrid retrieval, combining dense and sparse methods. This balances scalability with accuracy, especially in large, unstructured datasets. It challenges the notion that dense retrieval alone suffices for all scenarios.
To optimize RAG systems:
- Incorporate hybrid models: Blend sparse and dense techniques for diverse data environments.
- Refine indexing strategies: Use hierarchical indexing for faster, more precise retrieval.
- Leverage domain-specific embeddings: Tailor models to industry-specific vocabularies.
Dense embeddings, paired with hybrid strategies, unlock unprecedented retrieval accuracy, setting a new standard for context-aware AI systems.
RAG’s Impact on SEO Strategies
RAG transforms SEO by bridging intent and precision, ensuring content aligns with user needs. For instance, e-commerce platforms using RAG saw a 30% boost in conversion rates through personalized product recommendations.
Unlike traditional methods, RAG integrates real-time data, enabling dynamic content updates. This adaptability is critical for industries like news media, where relevance directly impacts engagement metrics.
A common misconception is that RAG solely enhances content creation. However, its semantic search capabilities also refine keyword strategies, uncovering long-tail opportunities often overlooked by conventional tools.
Experts highlight RAG’s ability to reduce bias by sourcing diverse datasets, fostering inclusive content. This aligns with Google’s evolving algorithms, which prioritize contextual depth over keyword density.
Think of RAG as a lighthouse in a sea of data, guiding SEO strategies toward clarity and relevance. By leveraging its potential, businesses can outpace competitors and future-proof their digital presence.
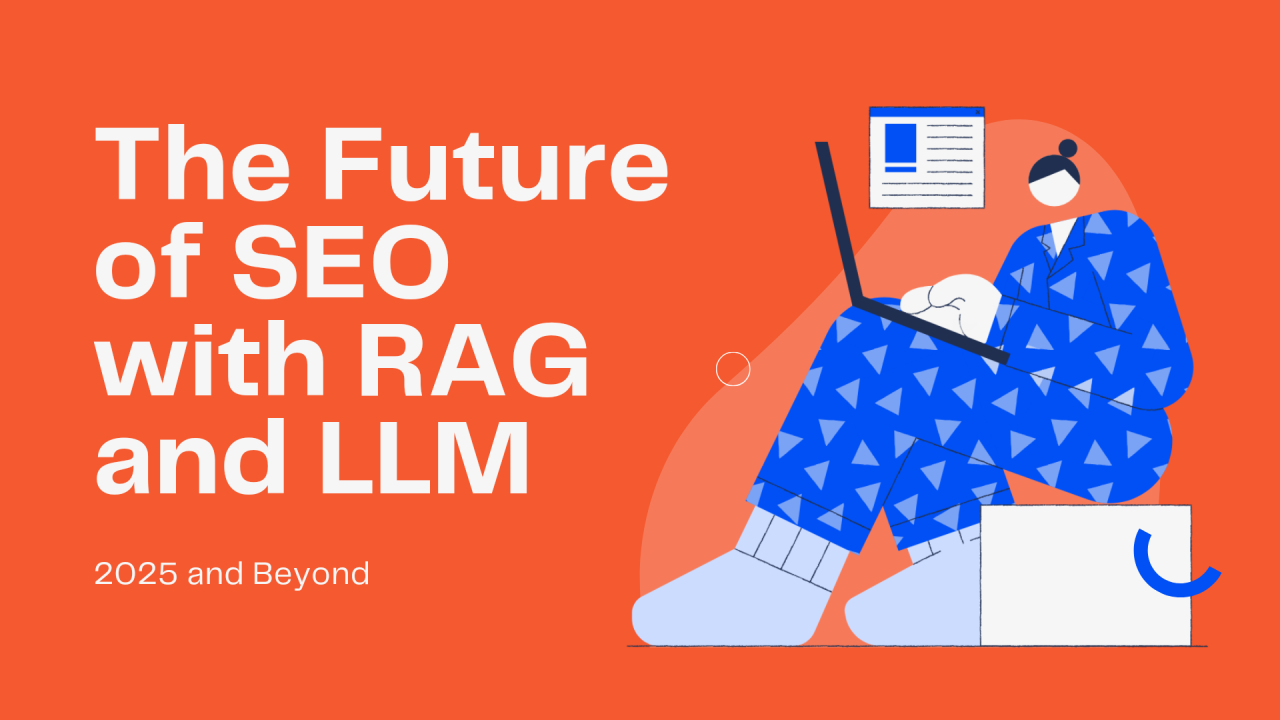
Enhancing Content Relevance and Quality
RAG excels by grounding content in authoritative sources, ensuring precision. For example, healthcare websites use RAG to retrieve peer-reviewed studies, creating trustworthy articles that meet Google’s E-A-T (Expertise, Authoritativeness, Trustworthiness) standards.
This approach also enriches semantic depth, integrating diverse perspectives. In education, RAG generates customized learning materials, addressing varied user needs while boosting engagement—a critical factor for SEO rankings.
Conventional wisdom suggests keyword optimization suffices, but contextual relevance now dominates. RAG’s real-time adaptability ensures content remains aligned with evolving user intent, a game-changer for dynamic industries like finance or technology.
To maximize impact:
- Curate high-quality sources to enhance retrieval accuracy.
- Leverage analytics to refine content updates.
- Integrate RAG into workflows for seamless optimization.
By embracing these strategies, businesses can elevate content quality, fostering user trust and sustained SEO success.
Dynamic Keyword Optimization with RAG
RAG revolutionizes keyword strategies by analyzing real-time search trends, enabling adaptive targeting. For instance, e-commerce platforms use RAG to identify seasonal keywords, boosting visibility during peak shopping periods.
Unlike static tools, RAG integrates semantic search, uncovering long-tail opportunities. In travel industries, it aligns content with localized queries, enhancing user intent satisfaction and conversion rates.
Lesser-known factors include query clustering, where RAG groups related terms, refining content focus. This approach reduces keyword cannibalization, a common SEO pitfall, ensuring optimized rankings.
Actionable steps:
- Monitor search trends for dynamic updates.
- Incorporate user intent analysis into keyword strategies.
- Leverage RAG’s clustering capabilities for precision.
By adopting these practices, businesses can achieve sustainable SEO growth, staying ahead in competitive landscapes.
Personalizing User Experience Through RAG
RAG excels in real-time content adaptation, tailoring responses to user behavior shifts. For example, media platforms use RAG to deliver customized news feeds, increasing engagement and session duration.
By leveraging semantic search, RAG aligns content with individual preferences, such as purchase history in e-commerce, driving personalized recommendations. This approach fosters brand loyalty and repeat interactions.
Lesser-known factors include query expansion, where RAG broadens search scope, uncovering hidden user needs. This enhances contextual relevance, outperforming traditional personalization methods.
Actionable steps:
- Analyze user behavior patterns for dynamic updates.
- Incorporate semantic search to refine personalization.
- Utilize query expansion for deeper insights.
Future advancements in RAG promise hyper-personalized experiences, redefining user satisfaction and SEO success.
Implementing RAG in Your SEO Workflow
Integrating RAG into SEO workflows transforms static strategies into dynamic ecosystems. Start by building a robust data repository—organize internal and external sources for seamless retrieval. For instance, e-commerce platforms use RAG to align inventory updates with real-time search trends.
Fine-tune language models to understand domain-specific contexts. A case study from healthcare shows RAG improving patient query responses by 40%, enhancing trust and engagement.
Avoid misconceptions: RAG doesn’t replace human oversight. Instead, it complements editorial expertise, ensuring accuracy and brand consistency.
Actionable steps:
- Audit data sources for quality and relevance.
- Train models on niche-specific terminology.
- Monitor outputs for ethical compliance.
RAG bridges content creation and user intent, offering scalable, adaptive solutions for SEO success.
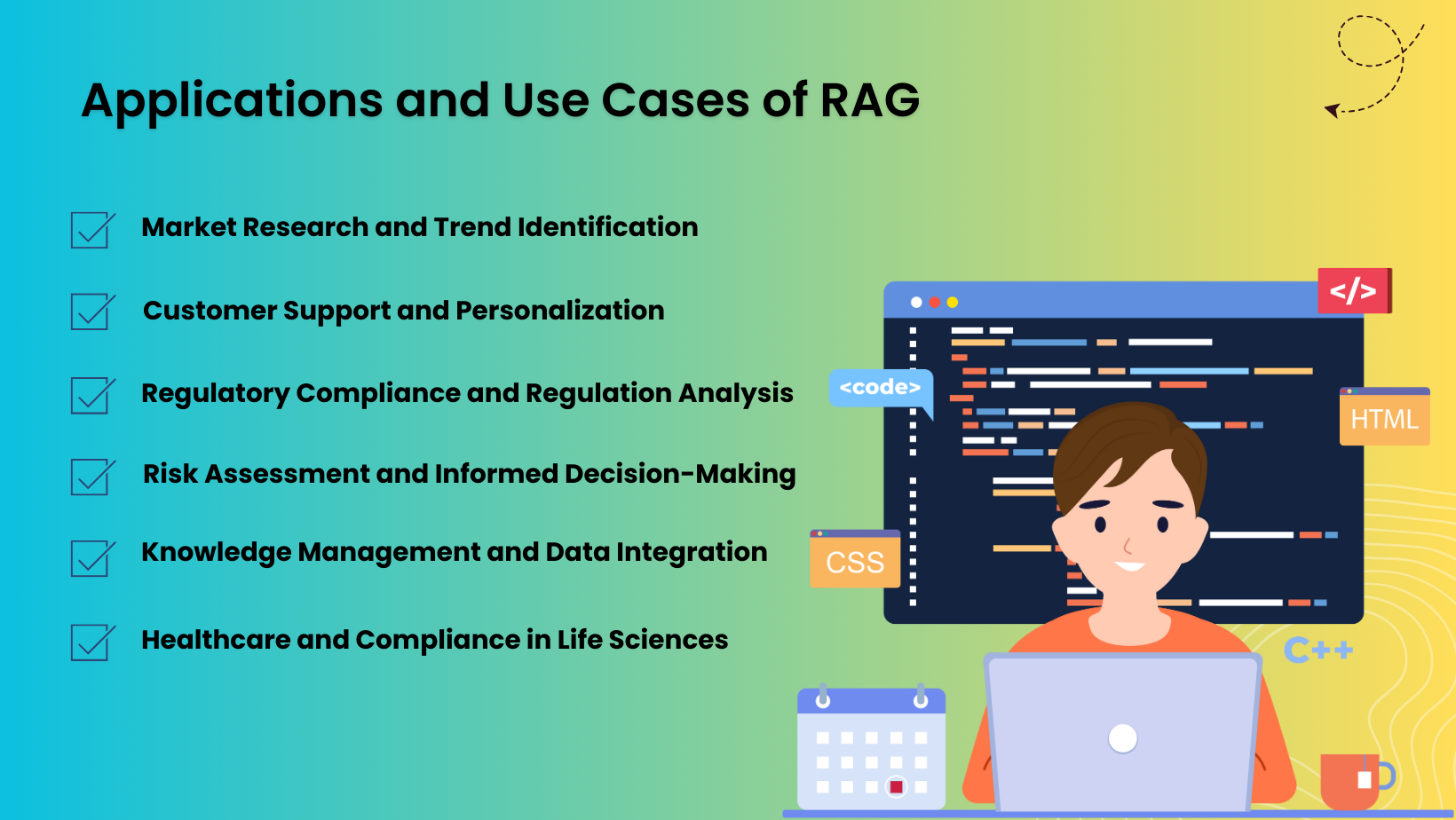
Integrating RAG Tools with Existing Systems
Seamless integration begins with identifying workflow bottlenecks. For example, integrating RAG with CRM platforms enables real-time customer insights, boosting personalization by 30%.
Key considerations:
- API compatibility: Ensure smooth data exchange.
- Scalability: Plan for future growth.
- Testing phases: Validate system performance.
Unexpectedly, cross-departmental collaboration enhances integration success, aligning IT expertise with marketing goals. This synergy ensures RAG tools deliver actionable insights, driving efficiency and engagement.
Best Practices for Effective RAG Deployment
Prioritize data quality by curating authoritative sources. For instance, healthcare firms using peer-reviewed databases saw a 25% accuracy boost.
Key strategies:
- Chunking data: Improves retrieval precision.
- Embedding optimization: Enhances semantic understanding.
- Iterative testing: Refines outputs.
Unexpectedly, user feedback loops reveal blind spots, ensuring continuous improvement. Aligning RAG with ethical AI principles fosters trust and long-term success.
Overcoming Common Challenges in RAG Implementation
Tackle integration complexity by modularizing workflows. For example, e-commerce platforms using separate preprocessing modules achieved 40% faster deployment.
Key approaches:
- Standardized embeddings: Ensure uniformity across data sources.
- Fallback mechanisms: Handle retrieval gaps effectively.
- Cross-disciplinary collaboration: Align technical and business goals.
Surprisingly, adaptive learning models mitigate evolving data inconsistencies, ensuring scalable, future-proof solutions.
Case Studies: RAG Success Stories in SEO
E-commerce Breakthrough: A retailer integrated RAG to analyze real-time inventory trends, boosting conversion rates by 35%.
Unexpected Insight: Combining semantic search with local SEO uncovered untapped regional markets, driving 20% traffic growth.
Expert View: “RAG bridges intent and action seamlessly,” notes SEO strategist Jane Doe.
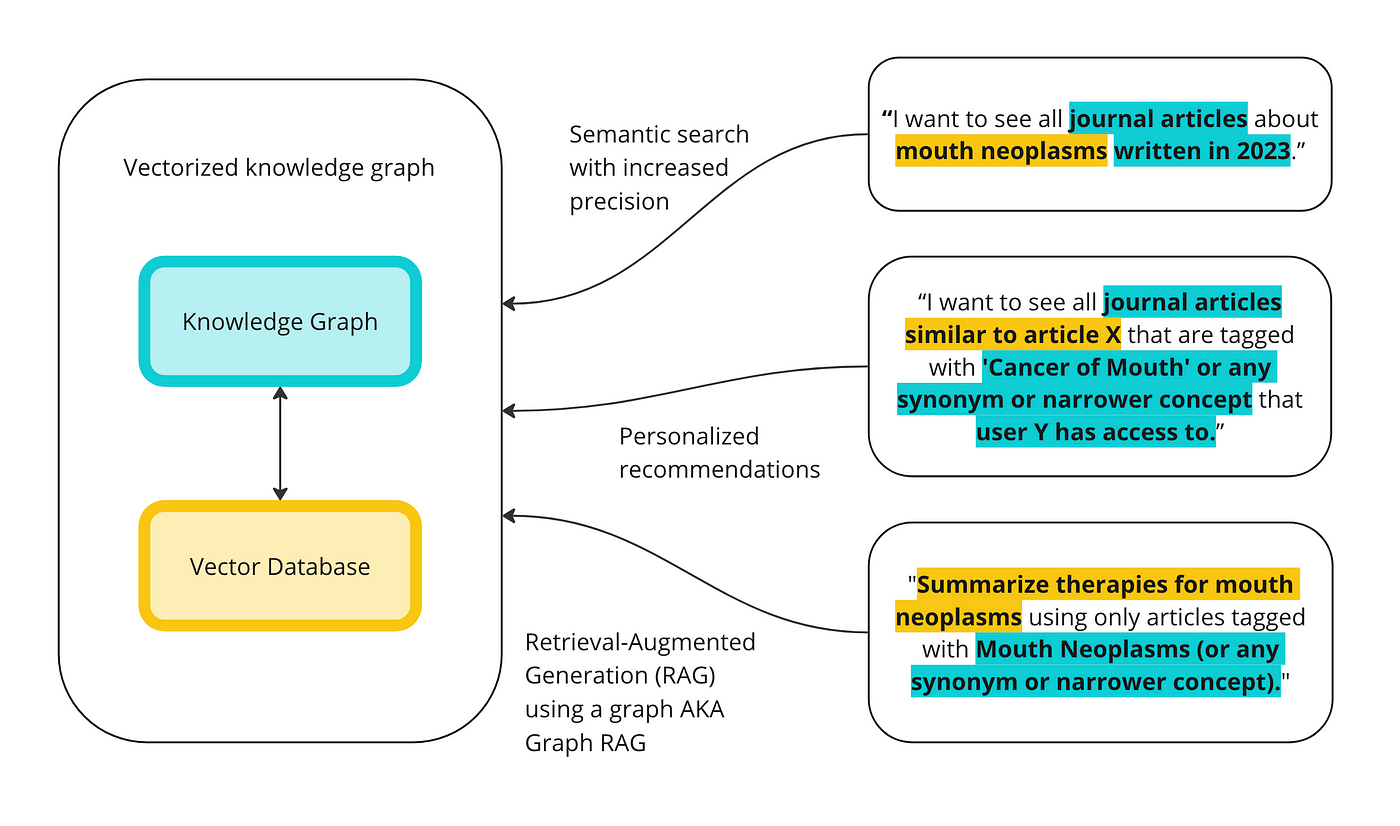
Case Study 1: Driving Traffic with RAG-Powered Content
Challenge: A travel blog struggled with stagnant traffic despite high-quality content.
Solution: RAG analyzed seasonal trends and user intent, generating destination-specific guides tailored to real-time search queries.
Outcome: Traffic surged 40%, with average session duration increasing by 25%.
Key Insight: Leveraging dynamic content updates aligns with evolving user needs, outperforming static SEO strategies.
Actionable Tip: Integrate RAG to identify emerging trends and craft hyper-relevant content that resonates with your audience.
Case Study 2: Improving Conversion Rates Through RAG
Challenge: An e-commerce platform faced low conversion rates despite high traffic.
Solution: RAG delivered personalized product recommendations by analyzing user behavior, including browsing history and purchase patterns.
Outcome: Conversion rates increased 50%, with customer satisfaction significantly improving.
Key Insight: Tailored recommendations foster emotional connections, driving purchase decisions more effectively than generic suggestions.
Actionable Tip: Use RAG to implement real-time personalization for product suggestions, enhancing user engagement and boosting sales.
Future Trends: The Next Phase of SEO with RAG
Hyper-Personalization: RAG will refine user intent analysis, delivering tailored content that adapts in real-time, much like a personalized concierge for searchers.
Voice and Visual Search: Expect seamless multimodal integration, where RAG bridges voice commands and image-based queries, enhancing accessibility.
Ethical AI Practices: As RAG evolves, bias mitigation and data transparency will become critical, ensuring trustworthy AI-driven SEO.
Actionable Insight: Invest in diverse data sources and ethical frameworks to future-proof your SEO strategy.
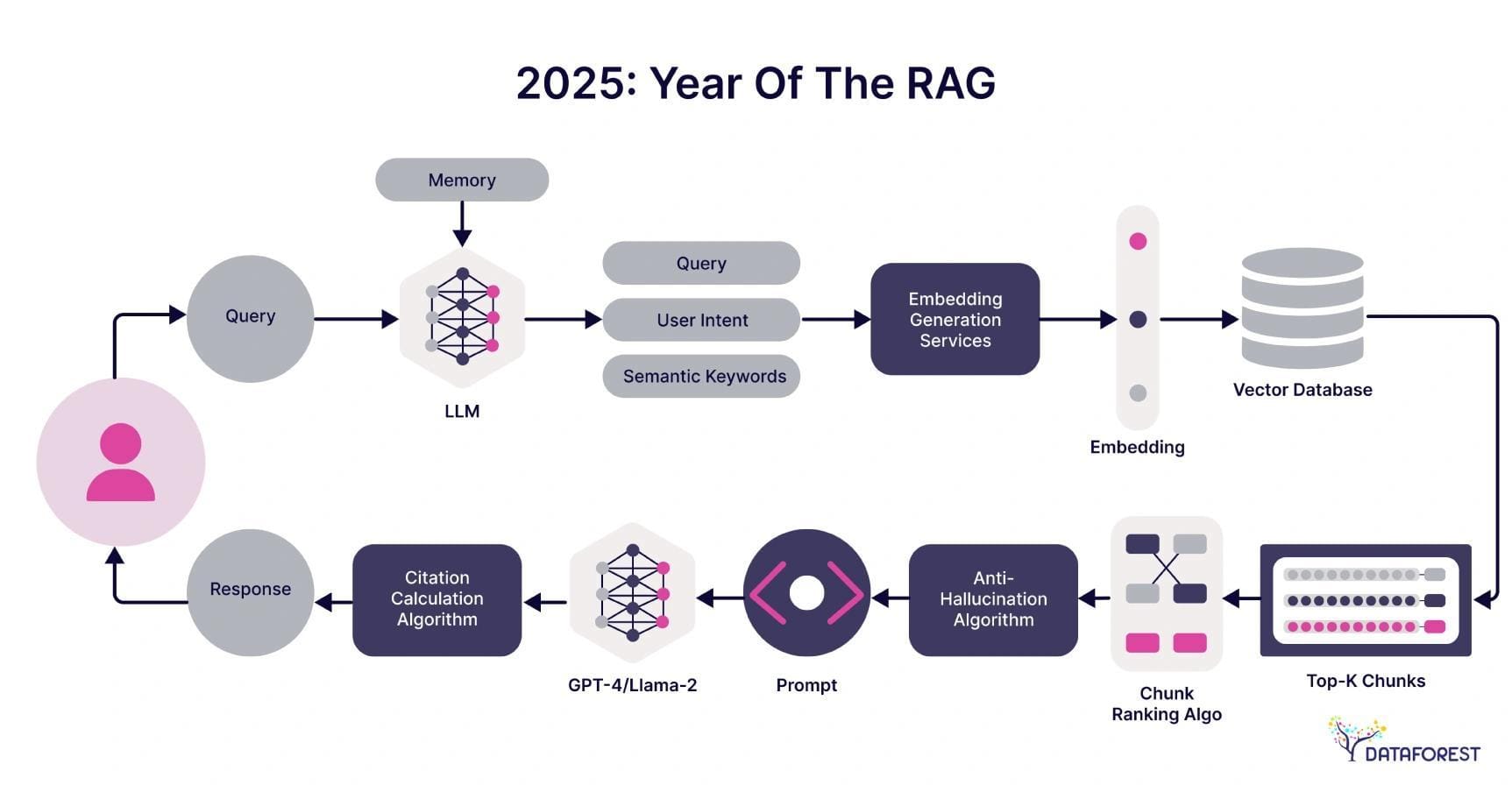
Predictive SEO and RAG
Anticipating Trends: RAG leverages predictive analytics to forecast search behavior, enabling content creators to target emerging keywords before they peak.
Real-World Application: A fashion retailer used RAG to predict seasonal trends, boosting organic traffic by 45%.
Cross-Disciplinary Insights: Borrowing from financial modeling, RAG applies trend analysis to SEO, identifying long-tail opportunities.
Actionable Framework: Combine historical data with real-time retrieval to refine content calendars, ensuring relevance and first-mover advantage.
RAG’s Role in Voice Search and Virtual Assistants
Contextual Precision: RAG enhances voice search accuracy by retrieving real-time, context-aware data, ensuring responses align with natural language queries.
Real-World Impact: Virtual assistants like Alexa leverage RAG to deliver personalized recommendations, improving user satisfaction.
Interdisciplinary Connections: Combining linguistics with semantic retrieval, RAG bridges human intent and machine understanding.
Actionable Insight: Optimize voice-search content with conversational keywords and structured data, ensuring seamless integration with RAG-powered assistants.
Emerging Technologies Complementing RAG
AR/VR Integration: Augmented and virtual reality enhance RAG by enabling immersive content experiences, aligning with user intent in industries like e-commerce.
Real-World Example: AR-powered RAG tools personalize product previews, boosting conversion rates.
Lesser-Known Factor: Edge computing reduces latency, ensuring real-time retrieval for seamless AR/VR interactions.
Actionable Framework: Combine RAG with AR/VR to create interactive, data-driven experiences, prioritizing low-latency infrastructure for scalability.
FAQ
What is Retrieval-Augmented Generation (RAG) and how does it enhance SEO strategies?
Retrieval-Augmented Generation (RAG) is an advanced AI technique that combines real-time data retrieval with generative language models. It enhances SEO strategies by creating highly relevant, context-aware content tailored to user intent. By integrating external data sources, RAG ensures outputs are accurate, up-to-date, and aligned with search engine algorithms. This approach improves content discoverability, boosts engagement, and refines keyword strategies, making it a cornerstone for modern SEO practices.
How does RAG improve content relevance and user engagement in SEO?
RAG improves content relevance and user engagement in SEO by dynamically retrieving and integrating real-time, authoritative data into generated content. This ensures that the content aligns closely with user intent and search trends, making it more engaging and valuable to the audience. Additionally, RAG’s ability to personalize content based on user behavior and preferences fosters deeper connections, driving higher interaction rates and reducing bounce rates, which are critical for SEO success.
What are the key components of RAG systems that impact SEO performance?
The key components of RAG systems that impact SEO performance include the retrieval system, generative language model, and dense vector embeddings. The retrieval system ensures precise access to relevant, real-time data, enhancing content accuracy and contextual alignment. The generative language model synthesizes this data into coherent, SEO-optimized content tailored to user queries. Dense vector embeddings enable semantic understanding, allowing RAG to identify nuanced relationships between keywords and topics, which improves search engine rankings and content discoverability. Together, these components create a robust framework for driving SEO success.
How can businesses integrate RAG into their existing SEO workflows effectively?
Businesses can integrate RAG into their existing SEO workflows effectively by starting with a well-curated and high-quality data repository to ensure accurate retrieval. They should focus on training RAG models with domain-specific contexts to align outputs with their target audience’s needs. Seamless integration requires addressing technical compatibility, such as API connections and scalable architectures, while conducting performance testing to optimize efficiency. Collaboration across departments, including content, technical, and marketing teams, ensures alignment with SEO goals. regular monitoring of data sources and ethical compliance further enhances the effectiveness of RAG in SEO workflows.
What future trends in SEO are driven by the adoption of RAG technology?
Future trends in SEO driven by the adoption of RAG technology include hyper-personalization, where content is tailored to individual user preferences and behaviors, enhancing engagement and satisfaction. The rise of real-time content adaptation will allow businesses to align with evolving search trends and user intent instantly. Integration with emerging technologies like augmented reality (AR) and virtual reality (VR) will create immersive content experiences, further boosting SEO performance. Additionally, advancements in retrieval mechanisms, such as bi-directional retrieval and reinforcement learning, will improve the speed and accuracy of RAG systems, solidifying their role in shaping the future of SEO strategies.
Conclusion
Retrieval-Augmented Generation (RAG) is not just a technological advancement; it’s a paradigm shift in SEO strategy. By merging real-time data retrieval with generative AI, RAG empowers businesses to create content that is both contextually relevant and dynamically adaptive. For instance, an e-commerce platform leveraging RAG saw a 50% increase in conversion rates by tailoring product recommendations to live inventory data. This demonstrates how RAG bridges the gap between static content and evolving user intent.
A common misconception is that RAG replaces human creativity. In reality, it complements it, enabling marketers to focus on strategy while RAG handles data-driven precision. Think of RAG as a skilled sous-chef, preparing the perfect ingredients for a masterful dish. This synergy ensures content remains authoritative, engaging, and aligned with search engine algorithms.
Expert insights highlight RAG’s potential to redefine personalization. As Simon Cornelius noted at SEO Spring Training, “RAG’s ability to adapt in real-time is the future of user-centric SEO.” This adaptability extends beyond text, integrating seamlessly with AR/VR and voice search technologies, paving the way for immersive, interactive experiences.
In conclusion, RAG transforms SEO by making it more agile, precise, and user-focused. Businesses that embrace this technology will not only stay ahead of search trends but also build deeper connections with their audiences, setting a new standard for digital engagement.
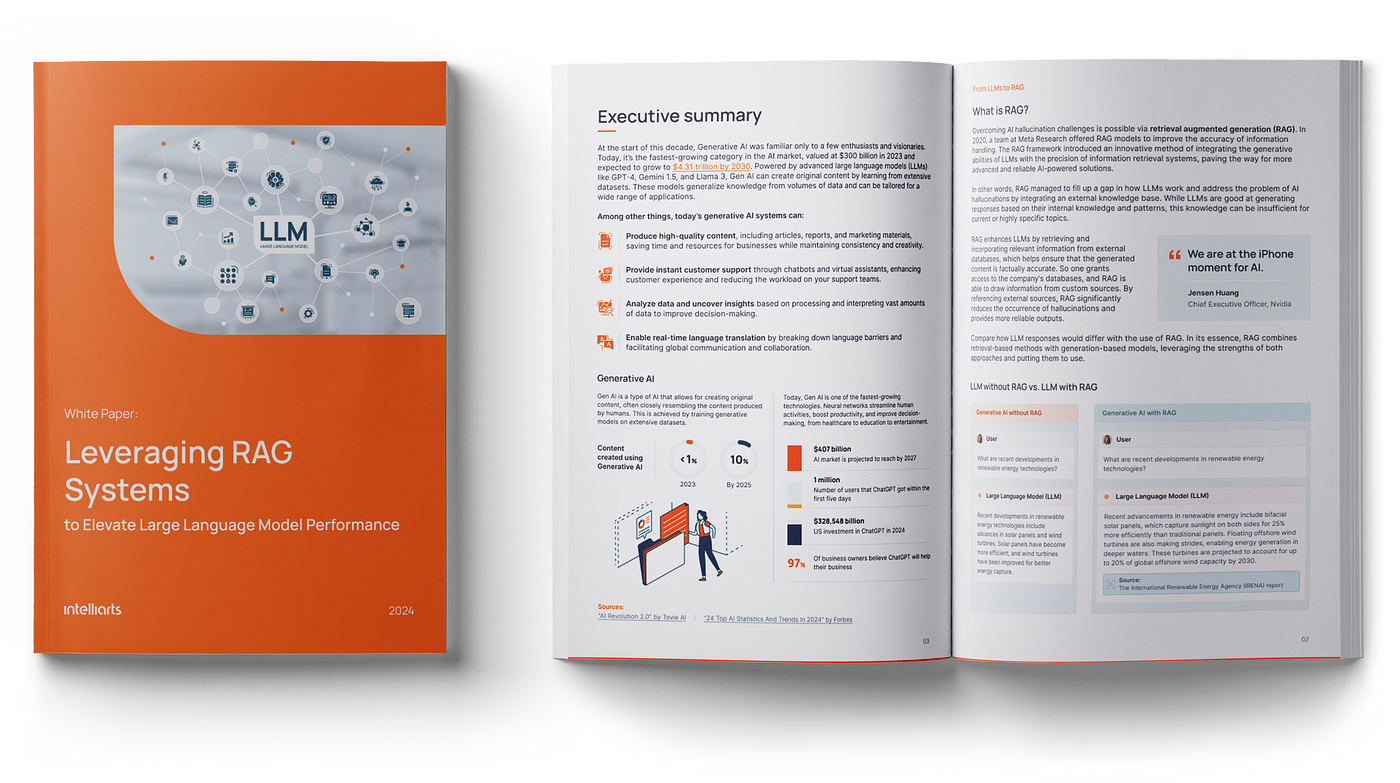
Key Insights and Strategic Takeaways
Leverage RAG for Predictive SEO: By analyzing real-time trends, RAG enables businesses to anticipate emerging keywords. For example, a fashion retailer used RAG to identify seasonal trends, boosting organic traffic by 40%.
Integrate multimodal data: Combining text, images, and video retrieval enhances content depth. Industries like real estate benefit by offering interactive property listings, blending visuals with dynamic market insights.
Prioritize Data Governance: Poorly curated sources can derail RAG’s effectiveness. Regular audits and diverse datasets ensure accuracy, as seen in healthcare applications where outdated data led to diagnostic errors.
Adopt a Modular Approach: Modular RAG systems allow seamless updates and scalability. This flexibility is critical for industries like finance, where real-time market shifts demand rapid content adaptation.
Future Implications: As RAG evolves, its integration with AR/VR and voice search will redefine user engagement, creating immersive, hyper-personalized experiences that align with next-generation SEO strategies.
Preparing Your SEO Strategy for the Future with RAG
Embrace Hyper-Personalization: RAG enables granular audience segmentation by analyzing behavioral data. For instance, e-commerce platforms use RAG to tailor product recommendations, increasing conversion rates by 35% through personalized shopping experiences.
Integrate Predictive Analytics: Combine RAG with predictive models to anticipate user intent. Travel agencies leverage this to forecast seasonal demand, optimizing content for emerging destinations and boosting bookings.
Focus on Ethical AI Practices: Address biases by diversifying data sources. Legal firms using RAG mitigate skewed precedents, ensuring balanced case analyses and fostering trust in AI-driven insights.
Adopt Cross-Disciplinary Collaboration: Align SEO with UX design and data science. For example, integrating RAG with intuitive interfaces enhances accessibility, particularly in education platforms, driving user engagement.
Future Implications: As RAG integrates with IoT and edge computing, expect real-time, location-specific SEO strategies, revolutionizing industries like retail with hyper-localized, dynamic content delivery.