AI-Powered Customer Support: How Next-Gen RAG Chatbots Will Replace Tier-1 Agents
Next-gen RAG chatbots are set to replace Tier-1 support agents by providing faster, smarter, and more accurate responses. This guide explores how AI-powered customer support is evolving to improve efficiency, reduce costs, and enhance user experiences.
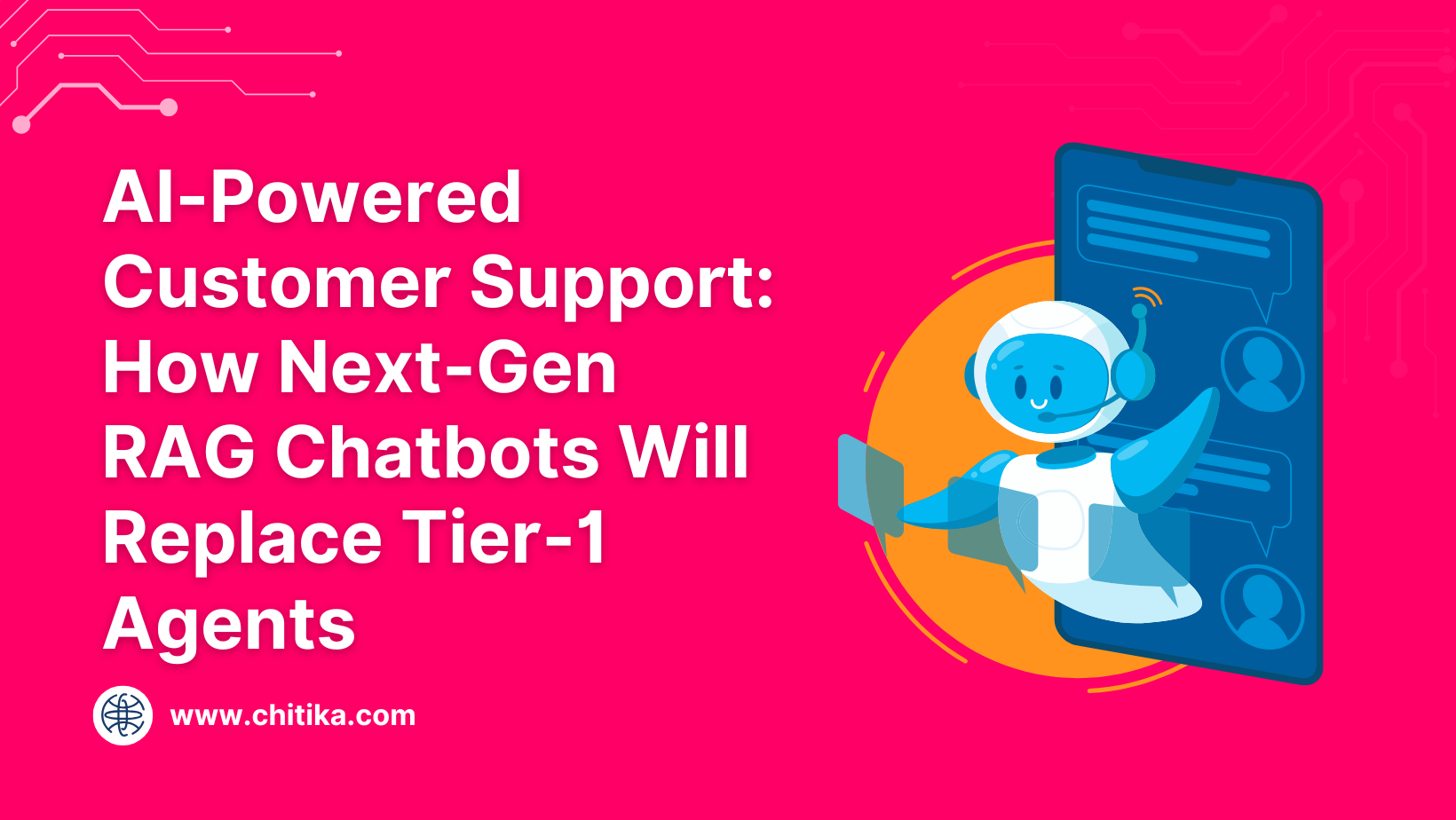
Imagine calling customer support, dreading the endless wait times and scripted responses—only to be greeted by an assistant who instantly understands your issue, pulls real-time data, and resolves your problem in seconds.
No hold music, no transfers, no frustration. This isn’t a glimpse into the distant future; it’s happening now.
AI-powered RAG chatbots aren’t just taking over Tier-1 support—they’re redefining customer service. The days of repetitive, inefficient interactions are fading, replaced by a system that adapts, learns, and responds with precision.
However, as businesses race to implement these AI-driven assistants, one question remains: How far can they go?
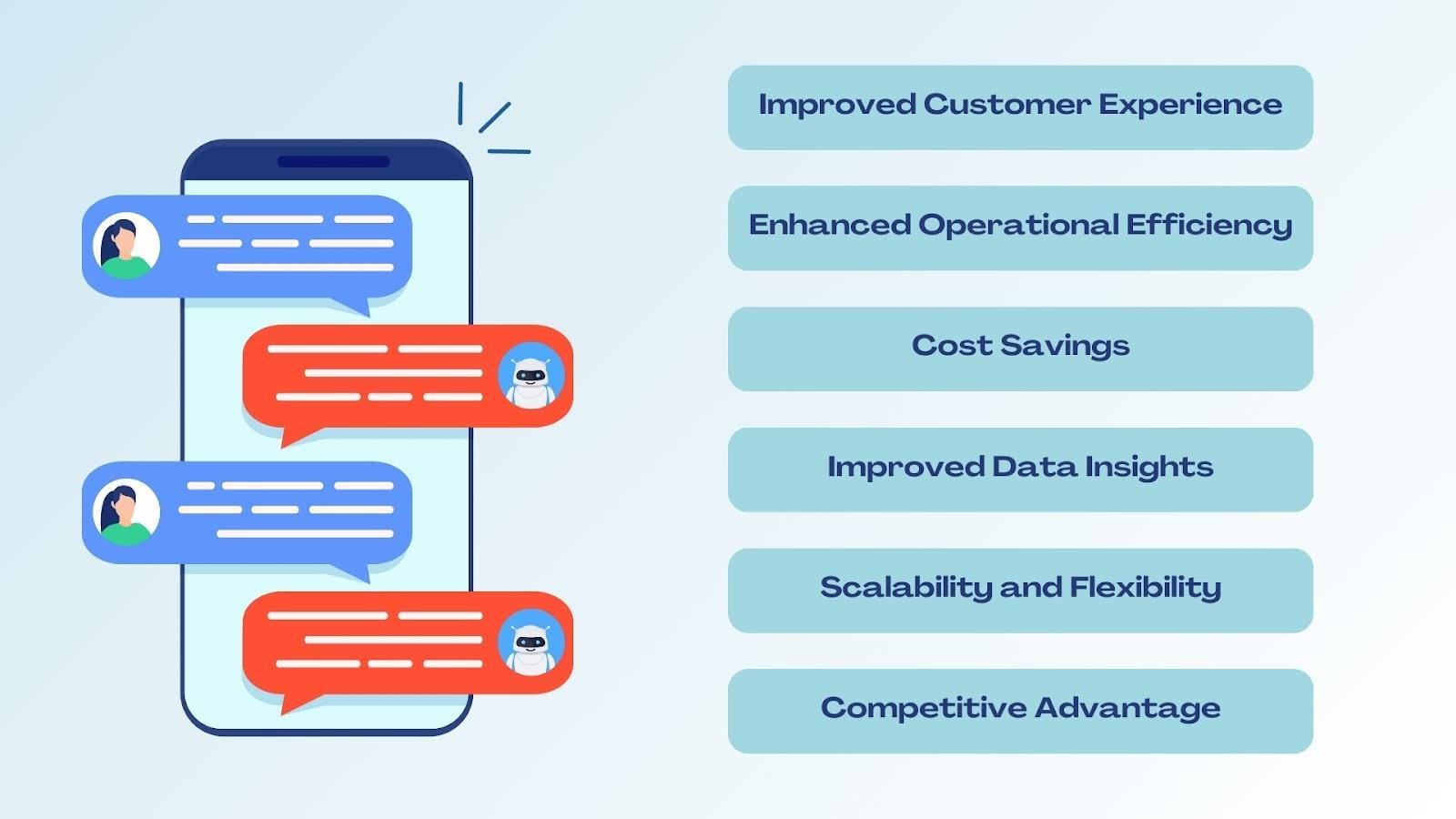
Defining AI-Powered Customer Support
One critical dimension of AI-powered customer support is its ability to leverage predictive analytics to address customer needs preemptively.
RAG chatbots can anticipate issues before they escalate by analyzing historical data, browsing patterns, and sentiment cues.
For instance, in e-commerce, these systems can detect when customers are likely to abandon their cart and proactively offer assistance or incentives, such as discounts or free shipping. This not only reduces churn but also enhances conversion rates.
What sets RAG chatbots apart is their integration with real-time data streams. Unlike traditional models, they adapt dynamically to evolving customer contexts, ensuring relevant responses. For example, in the travel industry, chatbots can provide updated flight information or alternative booking options during disruptions, minimizing customer frustration.
However, the success of these systems hinges on data quality and ethical AI practices. Poorly curated datasets or biased algorithms can lead to inaccurate predictions, eroding trust. Businesses must prioritize transparency and continuous model refinement.
Role of RAG in Tier-1 Replacement
A pivotal aspect of RAG chatbots in replacing Tier-1 agents lies in their contextual adaptability.
Unlike traditional chatbots, which often falter in multi-step or nuanced scenarios, RAG systems excel by dynamically retrieving and synthesizing information from verified sources.
This capability enables them to handle complex queries, such as troubleshooting technical issues or processing multi-layered refund requests, without human intervention
Another underexplored factor is intelligent escalation management. RAG chatbots resolve routine inquiries and identify when human expertise is required, routing customers to the appropriate agent with detailed context. This reduces resolution times and enhances customer satisfaction while optimizing agent workloads.
Moreover, integrating multimodal capabilities, such as VoiceRAG, redefines Tier-1 interactions. By combining voice-based communication with real-time data retrieval, these systems deliver near-human conversational experiences, further bridging the gap between automation and empathy.
RAG Chatbot Fundamentals
At their core, RAG chatbots combine retrieval-based and generation-based AI techniques, creating a hybrid model that outperforms traditional chatbots in both accuracy and adaptability.
Unlike rule-based systems that rely on static scripts, RAG chatbots dynamically pull verified data from vast knowledge bases, ensuring responses are contextually relevant and up-to-date.
A striking example is their application in healthcare.
By accessing real-time patient records and medical guidelines, RAG chatbots can provide tailored advice, such as medication reminders or symptom assessments, reducing the burden on medical staff. This contrasts sharply with older models, often failing in high-stakes, nuanced scenarios.
One common misconception is that RAG chatbots are limited to text-based interactions. However, advancements like VoiceRAG demonstrate their multimodal potential, enabling seamless voice-based support. This integration mirrors the human ability to process and respond across multiple communication channels.
By blending machine learning with natural language processing, RAG chatbots resolve queries and anticipate needs, setting a new standard for proactive, personalized customer support. Their evolution signals a shift from reactive assistance to predictive engagement, reshaping customer expectations.
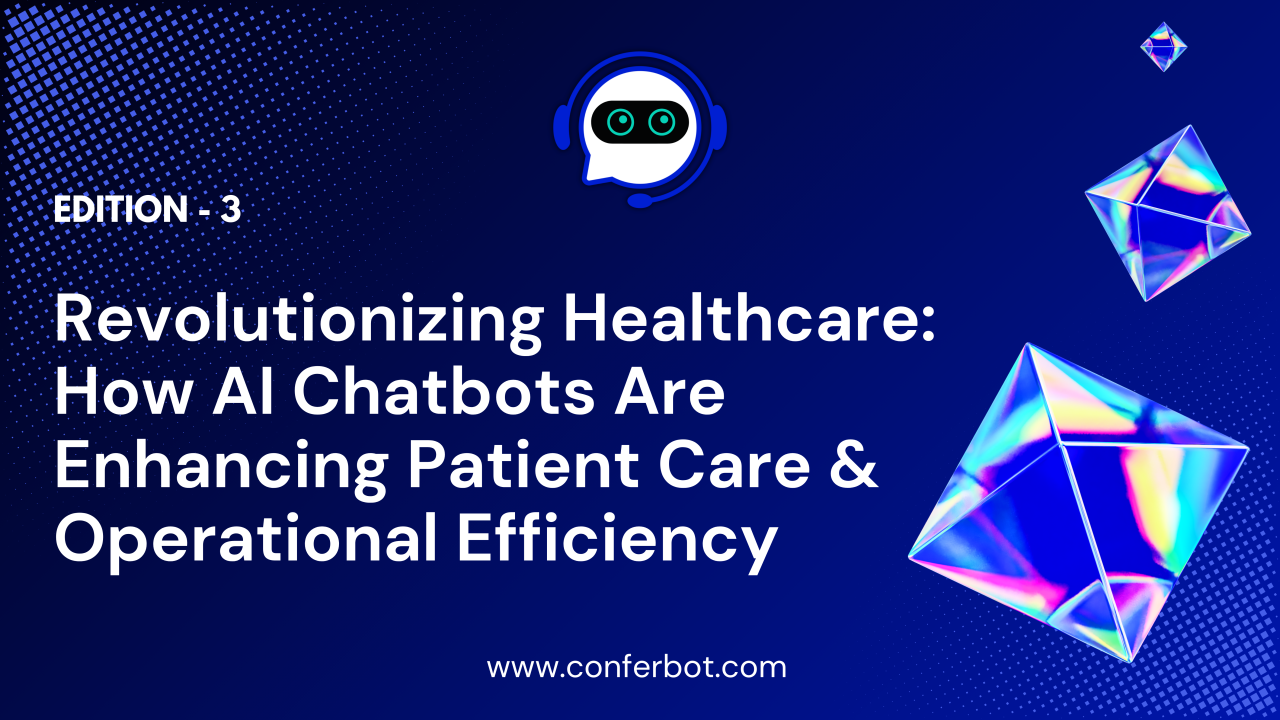
Core Architecture of Next-Gen RAG Chatbots
The architecture of next-gen RAG chatbots hinges on the seamless integration of retrieval modules and generation models, but the real innovation lies in their adaptive feedback loops.
These loops enable continuous learning by analyzing user interactions in real time, refining retrieval accuracy and response generation. Unlike static systems, this dynamic adaptability ensures that chatbots evolve alongside shifting user needs and data landscapes.
A critical advancement is using vector-based retrieval systems like FAISS or Pinecone, which store semantic representations of data rather than raw text. This allows RAG chatbots to retrieve contextually relevant information with unparalleled precision, even in ambiguous or multi-layered queries. For instance, in legal tech, these systems can parse complex case histories to provide actionable insights, saving hours of manual research.
Looking ahead, integrating neuro-symbolic AI—combining symbolic reasoning with neural networks—could further enhance decision-making, enabling chatbots to tackle abstract, logic-driven tasks.
This evolution positions RAG systems as tools and strategic partners in enterprise ecosystems.
Key Differentiators and Advantages
One standout differentiator of RAG chatbots is their contextual depth, achieved through real-time integration with external knowledge bases.
Unlike traditional systems, RAG chatbots dynamically retrieve and synthesize verified data, enabling them to precisely address nuanced queries.
This capability is particularly transformative in industries like healthcare, where chatbots can access up-to-the-minute medical guidelines to provide accurate symptom assessments or treatment recommendations.
A lesser-known factor driving their success is scalability without degradation in quality. By leveraging distributed computing frameworks, RAG systems maintain response accuracy even under high query volumes.
Challenging conventional wisdom, RAG chatbots demonstrate that automation can enhance, rather than diminish, empathy in customer interactions. Through advancements in emotional AI, these systems interpret sentiment and urgency, tailoring responses to reflect human-like understanding.
Implementation Strategies
Effective implementation of RAG chatbots requires a phased approach that balances technical precision with user-centric design.
A critical first step is data ecosystem readiness.
Businesses must curate diverse, high-quality datasets to ensure the chatbot delivers accurate, unbiased responses.
Another key strategy is iterative deployment. Instead of a full-scale rollout, companies can pilot RAG chatbots in specific use cases, such as handling FAQs or processing returns. This approach minimizes risk while refining performance through adaptive feedback loops.
A common misconception is that RAG systems are plug-and-play. In reality, cross-functional collaboration between AI developers, domain experts, and customer experience teams is essential.
Finally, businesses should prioritize emotional AI integration. RAG chatbots can humanize interactions by interpreting sentiment, fostering deeper customer loyalty.
This strategy enhances satisfaction and positions companies as leaders in empathetic automation.
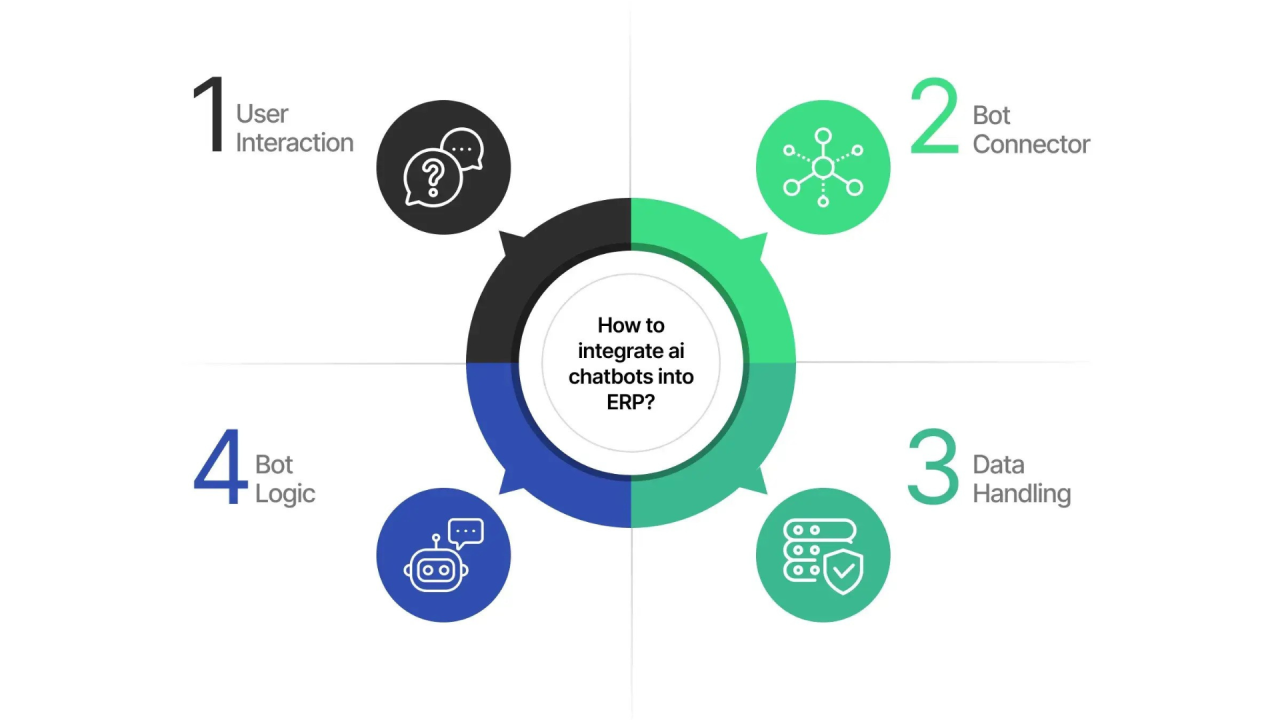
Integration with Existing Systems
Seamless integration of RAG chatbots with existing systems hinges on system interoperability and data synchronization.
A critical yet often overlooked factor is ensuring compatibility with legacy infrastructure. For example, many businesses still rely on older CRM platforms that lack native support for AI-driven tools.
Companies can bridge these gaps by employing middleware solutions or robust APIs, enabling RAG chatbots to access and update customer data in real-time without overhauling existing systems.
Another pivotal aspect is real-time data flow. Unlike traditional batch processing, RAG chatbots thrive on continuous data streams.
In e-commerce, for instance, integrating chatbots with inventory management systems allows them to instantly provide accurate stock updates or suggest alternatives, enhancing customer trust and satisfaction.
Data Management and Continuous Learning
A critical yet underexplored aspect of RAG chatbot success lies in adaptive knowledge base optimization.
Unlike static systems, RAG chatbots require dynamic updates to their knowledge repositories to remain relevant.
Automating this process through real-time ingestion pipelines ensures that the chatbot consistently reflects the latest policies, product updates, or regulatory changes. For instance, chatbots integrated with live feeds of compliance updates in the financial sector can instantly adapt to new legal requirements, reducing the risk of misinformation.
Another pivotal factor is the implementation of context-aware feedback loops. These systems can identify gaps in their knowledge base or recurring inaccuracies by analyzing user interactions.
For example, e-commerce platforms can leverage this feedback to refine product descriptions or FAQs, directly improving both chatbot performance and customer satisfaction.
Collaborative learning frameworks are a lesser-known but impactful approach, where RAG systems share anonymized insights across industries. This cross-domain knowledge transfer accelerates learning and enhances chatbot versatility, particularly in emerging fields like telemedicine.
Looking forward, integrating federated learning could revolutionize data management by enabling decentralized model training. This approach enhances privacy and ensures that RAG chatbots evolve in tandem with diverse, localized datasets, setting a new standard for continuous learning.
Advanced Applications and Use Cases
RAG chatbots are redefining customer support by extending their capabilities into high-stakes and specialized domains.
In healthcare, for example, these systems are not limited to symptom navigation; they now assist in post-operative care by providing real-time medication reminders and recovery tips tailored to individual patient records.
In the legal sector, RAG chatbots are transforming case preparation. By leveraging vector-based retrieval systems, they parse through complex legal precedents to deliver actionable insights, saving attorneys hours of manual research.
This application highlights their ability to handle abstract, logic-driven tasks, a domain previously thought exclusive to human expertise.
A common misconception is that RAG chatbots are confined to customer-facing roles. However, their integration into internal operations, such as employee training, showcases their versatility.
By delivering personalized learning modules based on real-time performance data, they enhance workforce efficiency.
These advanced applications underscore the untapped potential of RAG systems, positioning them as indispensable tools across industries.
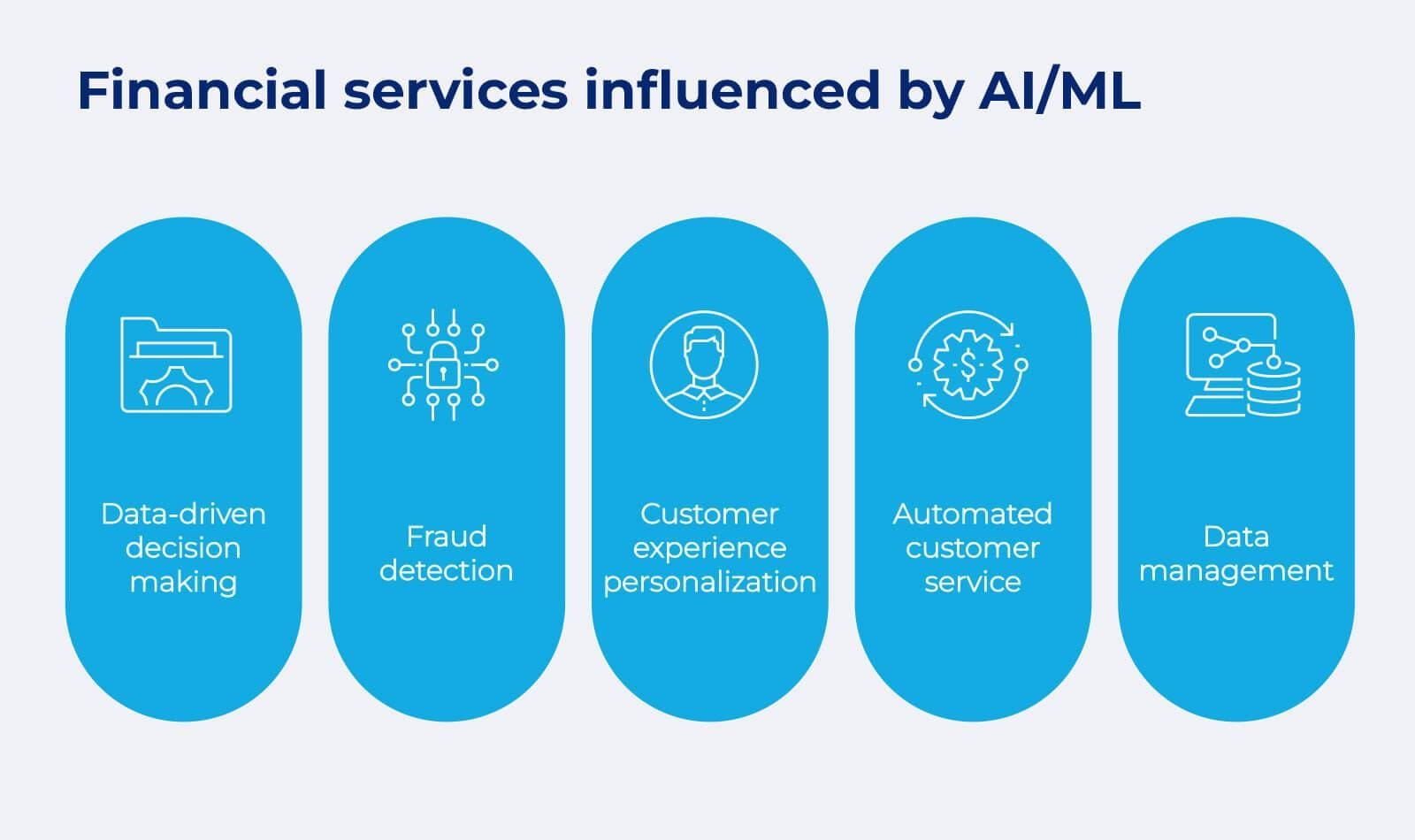
VoiceRAG and Multimodal Extensions
VoiceRAG represents a paradigm shift in customer support by enabling real-time, voice-to-voice interactions that feel inherently human.
Unlike traditional voice systems, VoiceRAG integrates Azure OpenAI’s GPT-4o Real-Time API with Azure AI Search, creating a seamless blend of conversational fluency and data precision.
This multimodal capability allows chatbots not only to respond verbally, but also adapt dynamically to the emotional tone and urgency of a customer’s voice.
One standout application is in technical support for complex products.
Imagine a customer assembling a smart home device: VoiceRAG can provide step-by-step voice instructions supplemented by visual aids sent via text or email.
A lesser-known factor driving VoiceRAG’s success is its decoupled architecture, which separates the front-end client from the real-time model.
This ensures compatibility with diverse platforms while enhancing security by isolating sensitive configurations. Additionally, session management capabilities allow for seamless conversation continuity across multiple channels.
Real-World Impact on Operational Efficiency
One transformative aspect of RAG chatbots in terms of operational efficiency is their ability to streamline resource allocation through intelligent automation.
By handling high volumes of repetitive queries, such as order tracking or account updates, these systems free human agents to focus on complex, high-value tasks.
A lesser-known yet critical factor is the role of adaptive feedback loops in optimizing workflows.
By analyzing interaction data in real-time, RAG chatbots identify bottlenecks and recurring issues, enabling businesses to refine processes proactively. For example, in e-commerce, insights from chatbot interactions can inform inventory management, reducing delays and improving fulfillment accuracy.
Challenging conventional wisdom, RAG systems demonstrate that automation can enhance collaborative efficiency. Integrating with CRM platforms provides agents with detailed customer histories, reducing resolution times and improving satisfaction.
FAQ
How do RAG chatbots differ from traditional Tier-1 agents?
RAG chatbots combine retrieval and generation AI, offering instant, data-backed responses. Unlike human agents, they scale effortlessly, minimize errors, and integrate emotional AI for personalized interactions.
How do RAG chatbots use real-time data to improve customer support?
By accessing live updates from CRMs, inventory systems, and schedules, RAG chatbots provide accurate, context-aware responses, ensuring proactive, real-time support.
Which industries benefit most from RAG-powered customer support?
Retail, healthcare, and finance lead adoption, with chatbots managing returns, offering medical guidance, and handling policy updates—boosting efficiency and customer satisfaction.
How does emotional AI enhance RAG chatbots?
Emotional AI enables chatbots to detect sentiment, adapt responses, and prioritize urgent cases, creating empathetic, human-like interactions that improve engagement and trust.
What’s the best way to implement RAG chatbots at scale?
Start small with FAQs, refine via feedback loops, and ensure high-quality data. Integrate emotional AI, real-time data, and continuous monitoring for seamless scalability.
Conclusion
RAG chatbots are more than just an upgrade to traditional customer service—they are redefining how businesses interact with customers.
As businesses continue integrating RAG chatbots, the focus will shift from simple automation to hyper-personalized engagement.
With advancements in multimodal AI, predictive analytics, and continuous learning, the question is no longer whether these systems will replace Tier-1 agents but how soon they will become the new standard across industries.