Is RAG Dead? How DeepSeek R1 is Redefining Custom RAG Chatbots
With the rise of DeepSeek R1, RAG chatbots are evolving beyond their limits. This post explores how advanced retrieval techniques, dynamic embeddings, and real-time adaptation are redefining chatbot intelligence, making them more accurate, scalable, and context-aware.
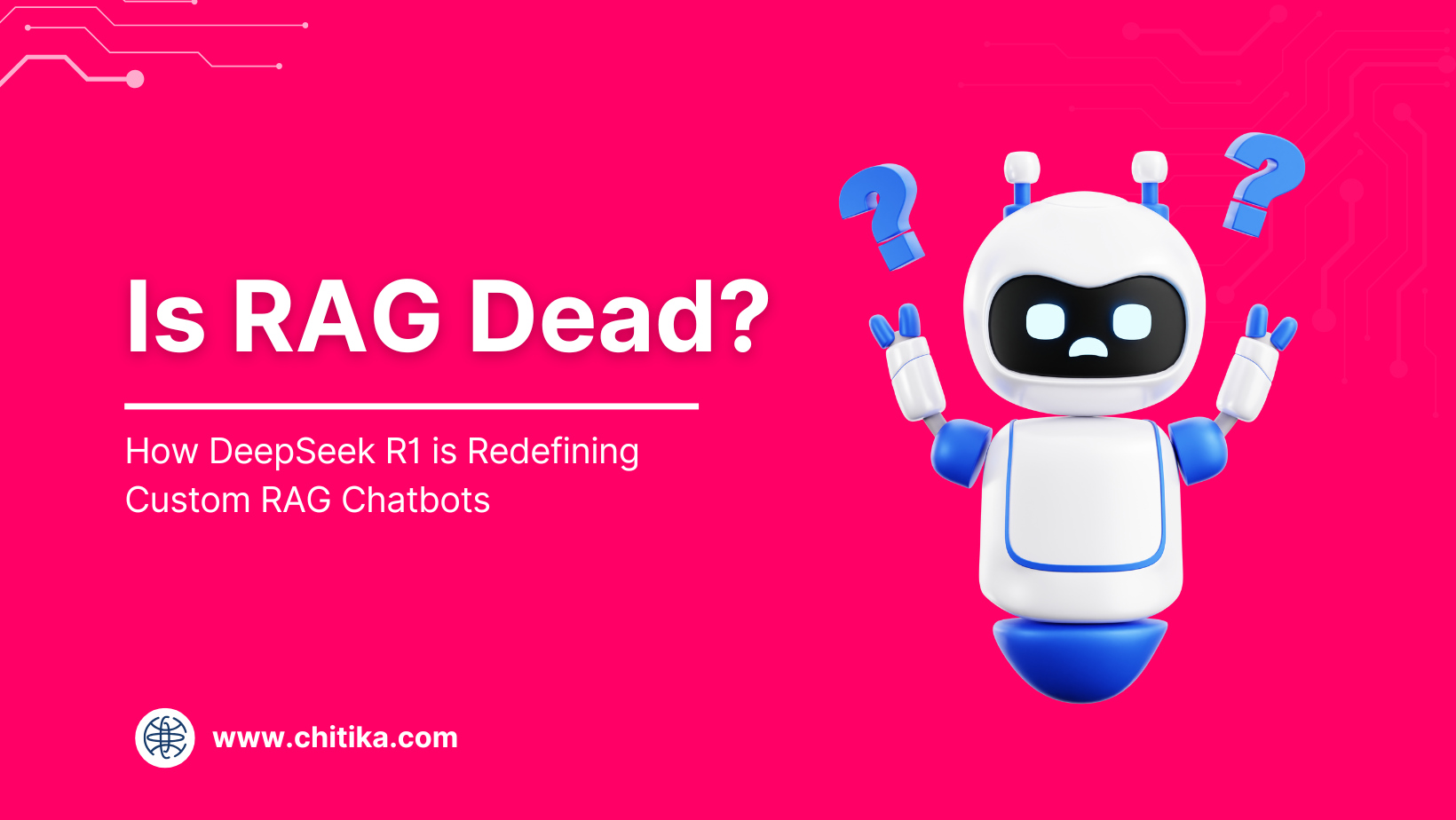
Is Retrieval-Augmented Generation (RAG) really on its last legs?
Well, it’s a bold claim, especially considering that RAG has been the backbone of countless AI systems, bridging the gap between static knowledge bases and dynamic, context-aware responses.
But here’s the twist: what if the problem isn’t RAG itself but how we’ve been using it?
Enter DeepSeek R1, a system that doesn’t just tweak the RAG formula but redefines it entirely. Could this be the key to unlocking a new era of custom chatbots? Or is it simply delaying the inevitable? Let’s unpack the tension and find out.
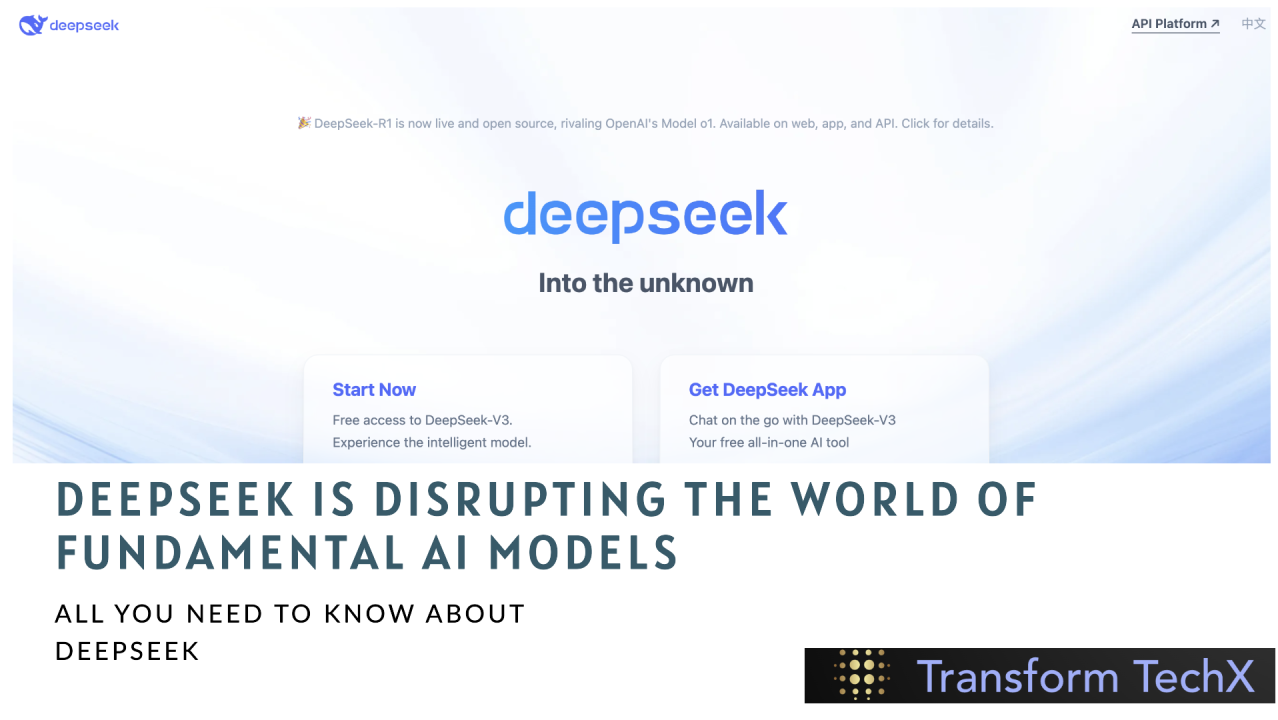
From RAG to DeepSeek R1: A New Era
DeepSeek R1 isn’t just an upgrade—it’s a paradigm shift.
Traditional RAG systems often struggled with scalability and context retention, but DeepSeek R1’s large context window and agentic reasoning address these limitations head-on.
It uses advanced vector embeddings to ensure that retrieval aligns seamlessly with user intent, even in multilingual or highly specialized domains.
What sets DeepSeek R1 apart is its agentic capabilities. Unlike static RAG systems, it dynamically refines its retrieval process based on real-time feedback, making it ideal for all sorts of research-intensive tasks.
Advantages and Limitations of Traditional RAG Chatbots
Traditional RAG chatbots excel at contextual accuracy, but their reliance on predefined knowledge bases can limit adaptability.
While RAG systems are faster than manual lookups, they can bottleneck when handling large, unoptimized datasets. This is especially problematic in industries like healthcare, where real-time precision is critical.
Retrieval models also often inherit biases from their source material, which skews results. Addressing this requires integrating diverse, unbiased datasets—a challenge many overlook.
However, systems like DeepSeek R1 use agentic reasoning and adaptive embeddings to refine retrieval dynamically. This approach mitigates limitations and opens the door to hyper-personalized applications, from multilingual marketing to legal tech.
The Need for Advanced Solutions
Retrieval latency is a silent killer in traditional RAG systems.
Imagine a healthcare chatbot tasked with retrieving patient-specific data during a live consultation. A delay of even a few seconds can disrupt the flow, eroding trust. DeepSeek R1 tackles this with adaptive embeddings that prioritize high-relevance data chunks, slashing retrieval times without sacrificing accuracy.
Here’s where DeepSeek’s context retention comes into play. Its large context windows allow it to quickly track user intent across interactions, making it invaluable for applications like healthcare or multilingual customer support.
Although conventional wisdom says bigger models are better, DeepSeek R1’s agentic reasoning proves otherwise. It dynamically refines retrieval based on real-time feedback.
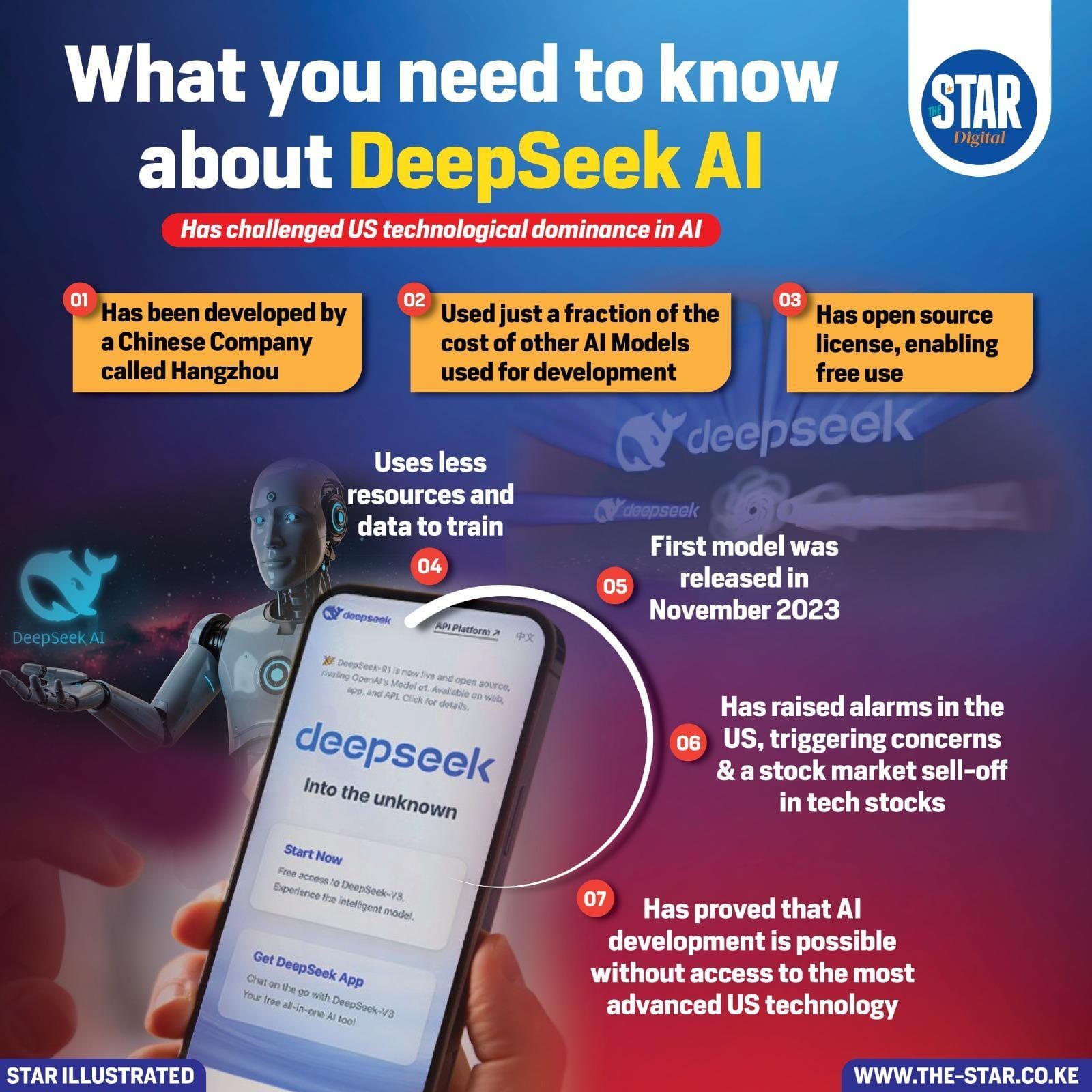
Technical Architecture of DeepSeek R1
DeepSeek R1’s architecture is like a well-oiled machine that balances power and efficiency.
At its core is the Mixture-of-Experts (MoE) framework, which activates only the parameters needed for a specific task. Think of it as a team of specialists—only the right experts are called in, reducing computational overhead while maintaining precision.
For example, during a single query, just 37 billion of its 671 billion parameters are engaged. This selective activation, powered by dynamic gating mechanisms, ensures faster responses without sacrificing quality.
DeepSeek R1 understands it. Its hybrid attention mechanisms dynamically adjust focus, capturing nuanced relationships in text.
This makes it ideal for complex tasks like multilingual marketing or STEM problem-solving, where precision and adaptability are non-negotiable.
System Design and Components
DeepSeek R1’s adaptive embedding pipeline personalizes retrieval by evolving in real-time based on user interactions. It integrates context-aware vector embeddings with multi-head latent attention, capturing subtle intent shifts—crucial for applications like customer support. Continuous feedback loops refine embeddings, proving adaptability trumps sheer model size.
Advanced Algorithms & Machine Learning
DeepSeek R1’s Mixture-of-Experts (MoE) activates only relevant parameters per query, reducing computational load while boosting accuracy. This hybrid attention mechanism enables nuanced responses in complex, multi-turn conversations, making it ideal for domains like healthcare. Modular AI designs enhance efficiency and scalability.
Data Integration & API Orchestration
Unlike static RAG systems, DeepSeek R1 dynamically adjusts retrieval based on API response patterns. In e-commerce, it harmonizes inventory data across sources in real-time. Predictive throttling and fallback mechanisms ensure seamless operation, proving flexible API frameworks are key to scalability.
Implementation & Performance
DeepSeek R1 adapts, learns, and evolves with interactions, outperforming traditional RAG systems in speed and accuracy. A legal chatbot using it reduced research time by 40% through a large context window, while its agentic reasoning anticipates user needs, transforming retrieval into intelligent recommendations.
Customization for Industries
DeepSeek R1’s open-source framework allows fine-tuning for niche applications. In retail, it integrates IoT data for dynamic inventory updates, boosting sales by 25%. In healthcare and finance, it enhances diagnosis and fraud detection by adapting embeddings to domain-specific data patterns.
Performance Metrics & Benchmarking
Achieving 50ms inference speed with MoE, DeepSeek R1 reduces computational overhead without sacrificing accuracy. Its 30% reduction in false positives is crucial in high-stakes applications like healthcare, proving that intelligent design outperforms brute-force scaling.
Scalability & Reliability
Scaling isn’t just about capacity—it’s about efficiency. DeepSeek R1’s multi-head latent attention maintains precision as data volume grows, while real-time feedback loops minimize errors, making it indispensable for industries like legal research, finance, and e-commerce.
The takeaway? RAG isn’t dead—it’s evolving with smarter, leaner AI solutions.
Comparative Analysis: RAG vs. DeepSeek R1
Traditional RAGs are like a GPS from the early 2000s—functional, but clunky. They retrieve relevant data, sure, but struggle with real-time adaptability and nuanced user intent.
DeepSeek R1, on the other hand, doesn’t just retrieve—it anticipates.
For example, in fraud detection, it identifies anomalies faster by learning from evolving patterns, something static RAG systems can’t match.
Note that RAG isn’t obsolete—it’s just limited. DeepSeek R1 builds on RAG’s foundation, adding scalability and cultural nuance. Think of it as upgrading from a flip phone to a smartphone.
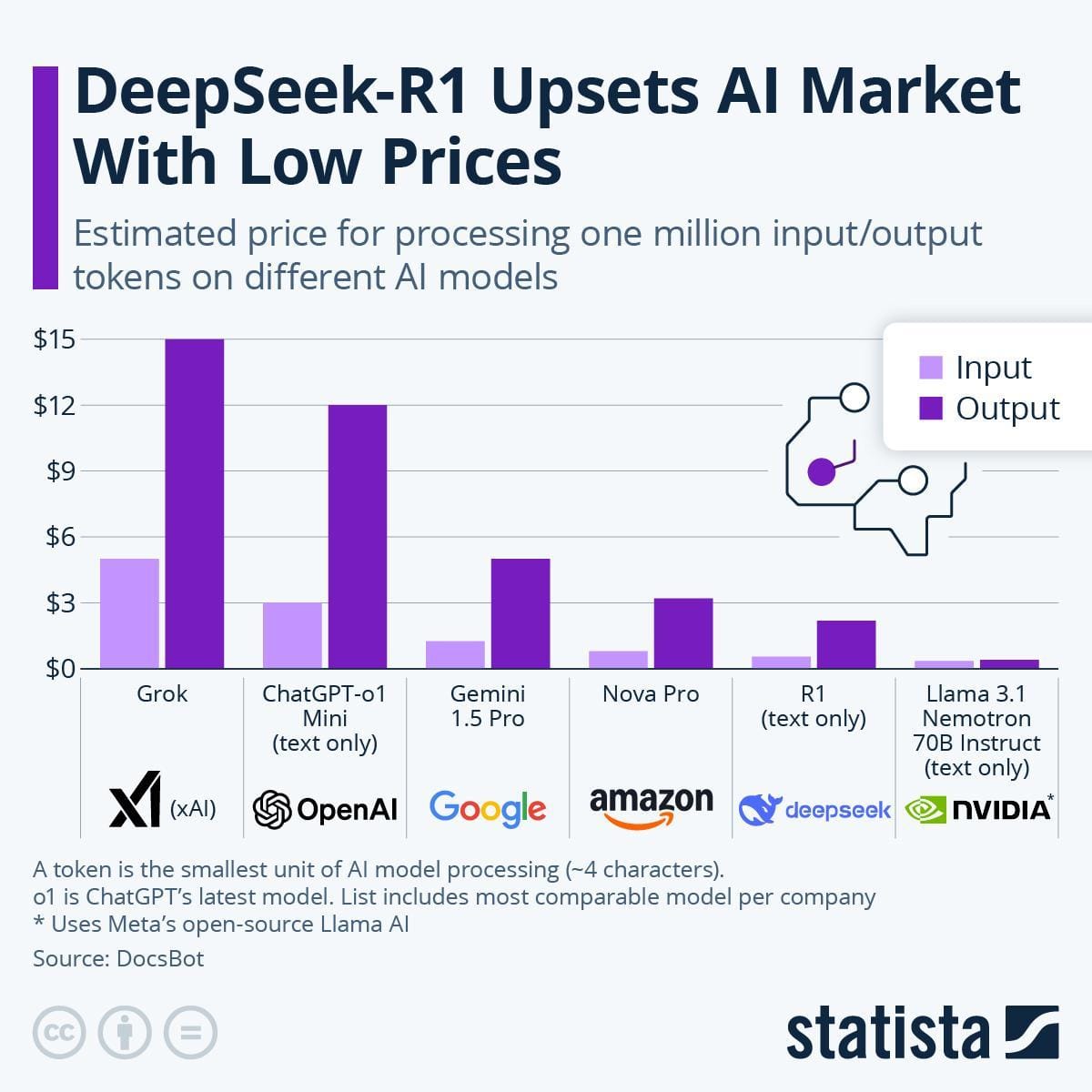
Efficiency and Response Accuracy
DeepSeek R1 redefines efficiency by leveraging its Mixture-of-Experts (MoE) architecture, which activates only task-relevant parameters.
This targeted approach slashes computational overhead, enabling response times as low as 50 milliseconds per query—ideal for real-time applications like supply chain optimization.
Compare this to traditional RAG systems, which often struggle with retrieval latency due to their one-size-fits-all parameter activation.
User Experience Enhancements
DeepSeek R1 transforms user experience by integrating Chain of Thought (CoT) reasoning into its retrieval process. Unlike traditional RAG systems that often deliver static, one-dimensional responses, CoT enables R1 to simulate human-like problem-solving.
R1’s adaptive embeddings don’t just retrieve data—they evolve with user interactions. Imagine a multilingual e-commerce platform where R1 adjusts its tone and recommendations based on regional preferences.
This dynamic personalization boosts engagement and conversion rates, a game-changer for global businesses.
As AI systems become integral to customer-facing roles, prioritizing nuanced, adaptive interactions will set the benchmark for future innovations.
Implications for AI and Chatbot Development
DeepSeek R1 is a wake-up call for how we think about AI chatbots. Here’s why: traditional RAG systems rely on static retrieval pipelines, but R1 flips the script with adaptive embeddings and real-time learning.
The misconception? That bigger LLMs makes RAG obsolete. In reality, R1 shows that smarter retrieval beats brute force. By integrating agentic reasoning, it bridges the gap between generative power and contextual precision.
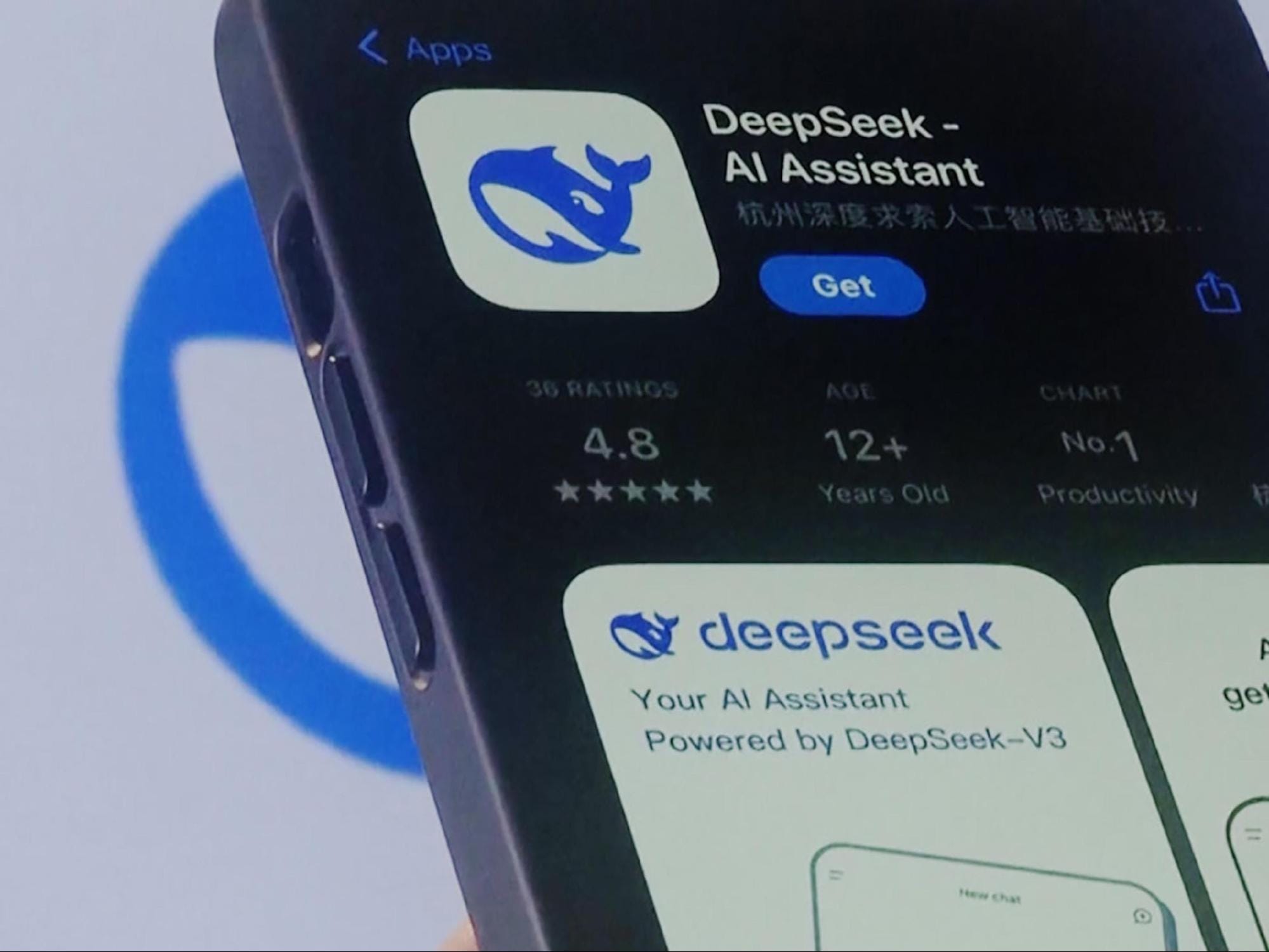
Impact on Natural Language Processing Advancements
DeepSeek R1 is reshaping Natural Language Processing (NLP) by prioritizing contextual depth over sheer model size.
FAQ
What is Retrieval-Augmented Generation (RAG) and why is it important for AI chatbots?
RAG combines real-time data retrieval with AI-generated responses, ensuring accuracy and contextual relevance. This reduces hallucinations and enhances adaptability, making chatbots more reliable.
How does DeepSeek R1 address the limitations of traditional RAG systems?
DeepSeek R1 improves scalability, retrieval accuracy, and context retention using Mixture-of-Experts (MoE) and agentic reasoning. It dynamically refines retrieval, reducing latency and enhancing multi-turn conversations.
What are the key innovations in DeepSeek R1 that redefine custom RAG chatbots?
DeepSeek R1 introduces agentic reasoning, large context windows, and adaptive embeddings for better accuracy. Its MoE framework optimizes efficiency while reducing computational costs.
Can DeepSeek R1 be integrated into existing chatbot frameworks, and how?
Yes, DeepSeek R1 offers API-based integration with chatbot frameworks, enabling real-time retrieval and seamless deployment. It supports open-source tools like Hugging Face for customization.
What industries benefit the most from DeepSeek R1’s advancements in RAG technology?
Healthcare, finance, retail, and manufacturing gain from its real-time insights, fraud detection, personalized recommendations, and predictive maintenance, improving efficiency and decision-making.
Conclusion
The misconception that RAG is obsolete ignores how models like DeepSeek R1 enhance LLMs rather than replace them.
By bridging retrieval and generation seamlessly, it proves that RAG isn’t dead—it’s evolving. And with DeepSeek R1, it’s thriving in ways we never imagined.