The Role of AI Retrieval in Building Intelligent Investment Strategies
Learn how AI retrieval is transforming investment strategies with real-time data analysis, predictive insights, and enhanced decision-making. This guide explores how AI-powered retrieval helps investors optimize portfolios and stay ahead in financial markets.
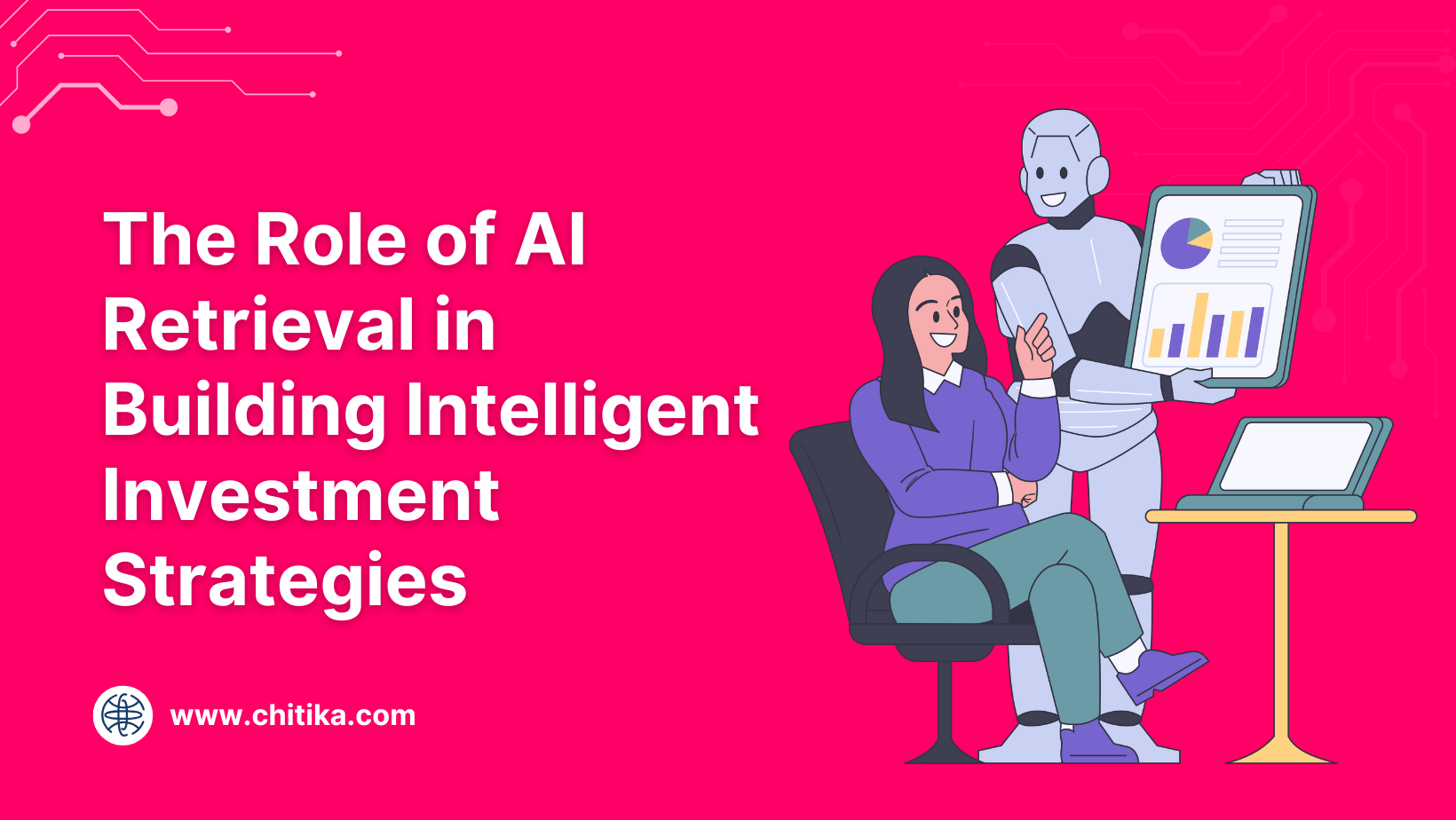
In today’s fast-paced financial markets, the sheer volume of data can often lead to more confusion than clarity, posing a significant challenge for investment strategies.
Investment strategies that once relied on intuition now face the challenge of cutting through the noise from earnings reports, social media, and global news.
But a game-changer is emerging: AI retrieval systems.
Unlike predictive models that chase the future, these systems uncover the most vital insights from the present. Could this overlooked technology be the secret to building intelligent investment strategies? Let’s find out.
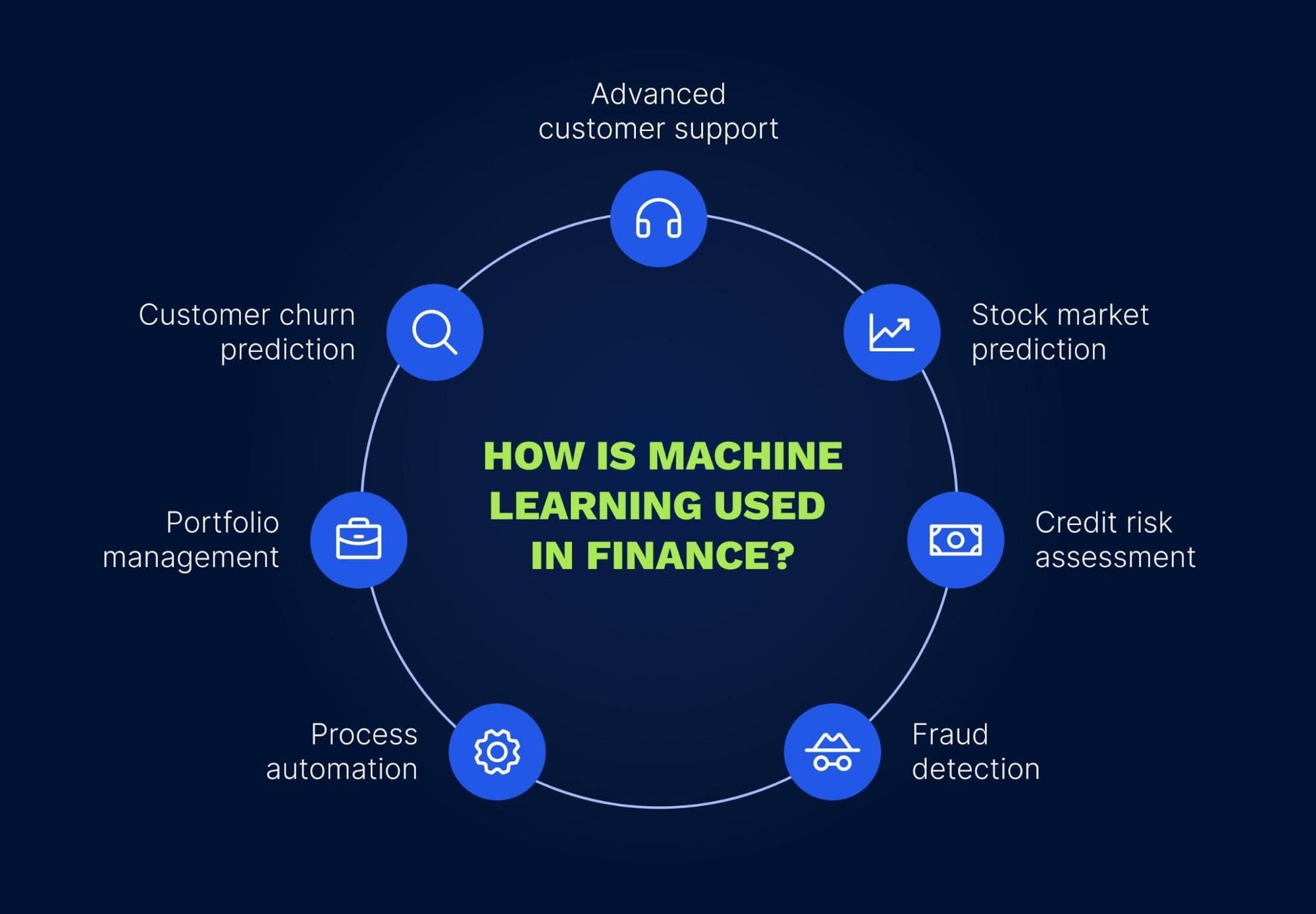
Key Concepts in AI-Driven Finance
One of AI’s greatest strengths in finance is its ability to understand and apply real-world context to data-driven decisions.
Unlike systems that rely on static datasets, AI-driven frameworks reassure investors by dynamically adapting to evolving market conditions using NLP techniques and real-time data ingestion.
For instance, NLP models can parse earnings call transcripts to detect subtle shifts in sentiment, which provides investors with early indicators of potential market movements.
A particularly compelling application lies in sentiment-aware trading algorithms.
These systems integrate unstructured data—such as social media chatter or geopolitical news—with structured financial metrics to refine decision-making.
These systems go beyond numbers by converting qualitative factors into measurable insights to offer a comprehensive and well-rounded view of market trends, keeping investors informed and aware.
Fundamentals of AI Retrieval for Investments
AI retrieval systems act like a guide, filtering vast amounts of data to highlight actionable insights without overwhelming investors.
Unlike predictive models that attempt to forecast future trends, retrieval systems excel at extracting contextually significant data from the present.
This insight allowed investors to adjust portfolios quickly, showcasing contextual intelligence's power.
A common misconception is that retrieval systems merely filter data. In reality, they dynamically adapt to evolving market conditions to uncover hidden relationships.
For instance, linking geopolitical events to commodity price fluctuations can expose opportunities invisible to traditional analysis.
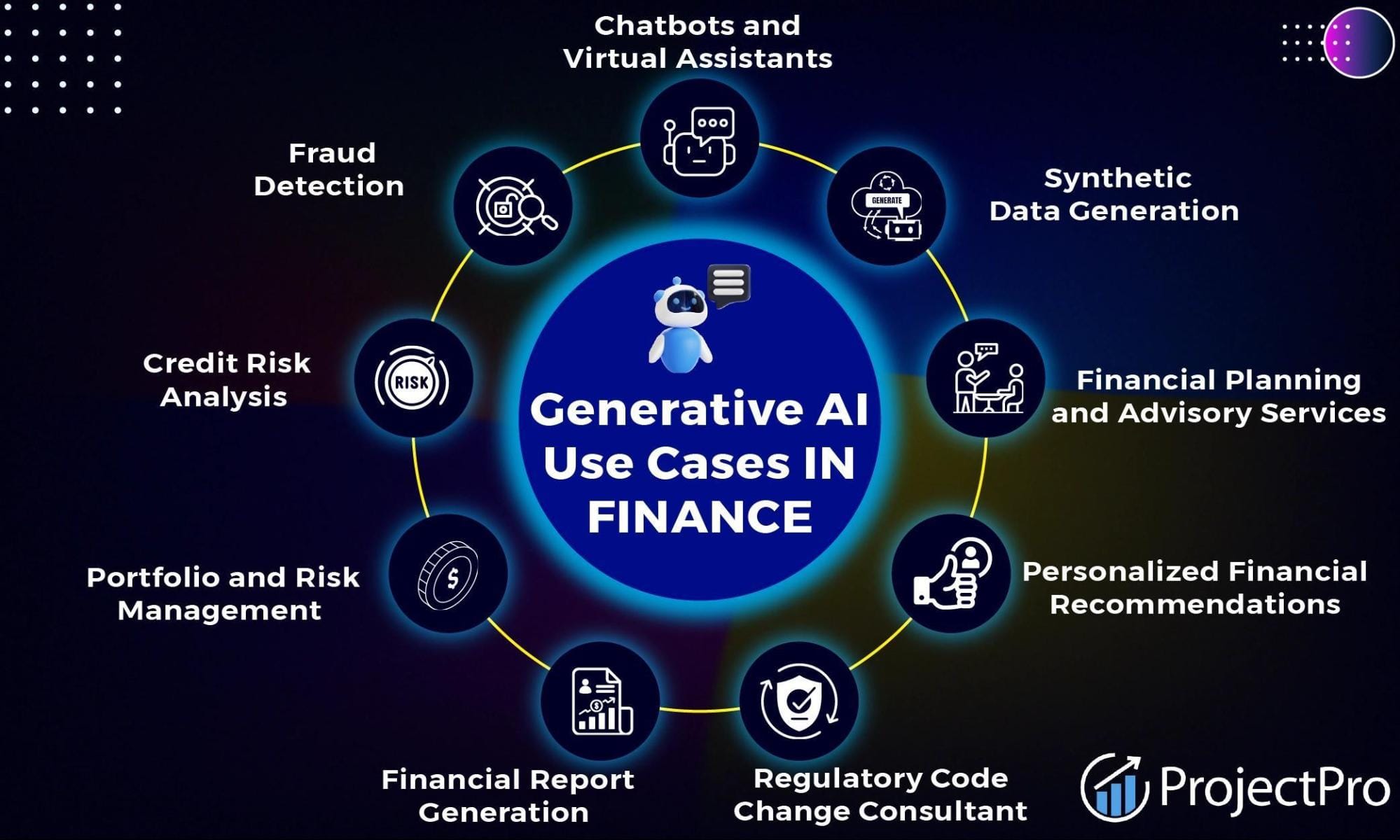
Defining AI Retrieval
AI retrieval is not merely about locating data; it’s about contextual alignment.
At its core, it involves dynamically extracting the most relevant information from vast, unstructured datasets to inform decisions in real-time.
This capability hinges on advanced techniques like knowledge graphs and semantic search, which go beyond keyword matching to understand relationships and intent.
Consider the use of vector embeddings in financial analysis. AI retrieval systems can measure semantic similarity by representing data points—such as earnings reports or geopolitical news—as high-dimensional vectors.
A lesser-known factor influencing outcomes is query optimization.
Retrieval systems must balance precision with recall, ensuring no critical insights are overlooked. This requires fine-tuning algorithms to adapt to evolving market conditions, a process akin to calibrating a telescope for clarity.
Data Integration & Machine Learning
One critical yet underexplored aspect of data integration in investment strategies is the role of intelligent data mapping.
Traditional integration methods often struggle with fragmented datasets, leading to incomplete analyses.
Machine learning (ML) transforms this process by automating the alignment of disparate data sources, ensuring consistency and accuracy. For example, ML algorithms can reconcile conflicting financial records by identifying patterns across structured and unstructured data, such as earnings reports and social media sentiment.
A particularly impactful application is in real-time data ingestion. ML-driven systems can process streaming data from financial markets using tools like Apache Kafka, enabling near-instantaneous updates to investment models. This capability is crucial for high-frequency trading, where milliseconds can determine profitability.
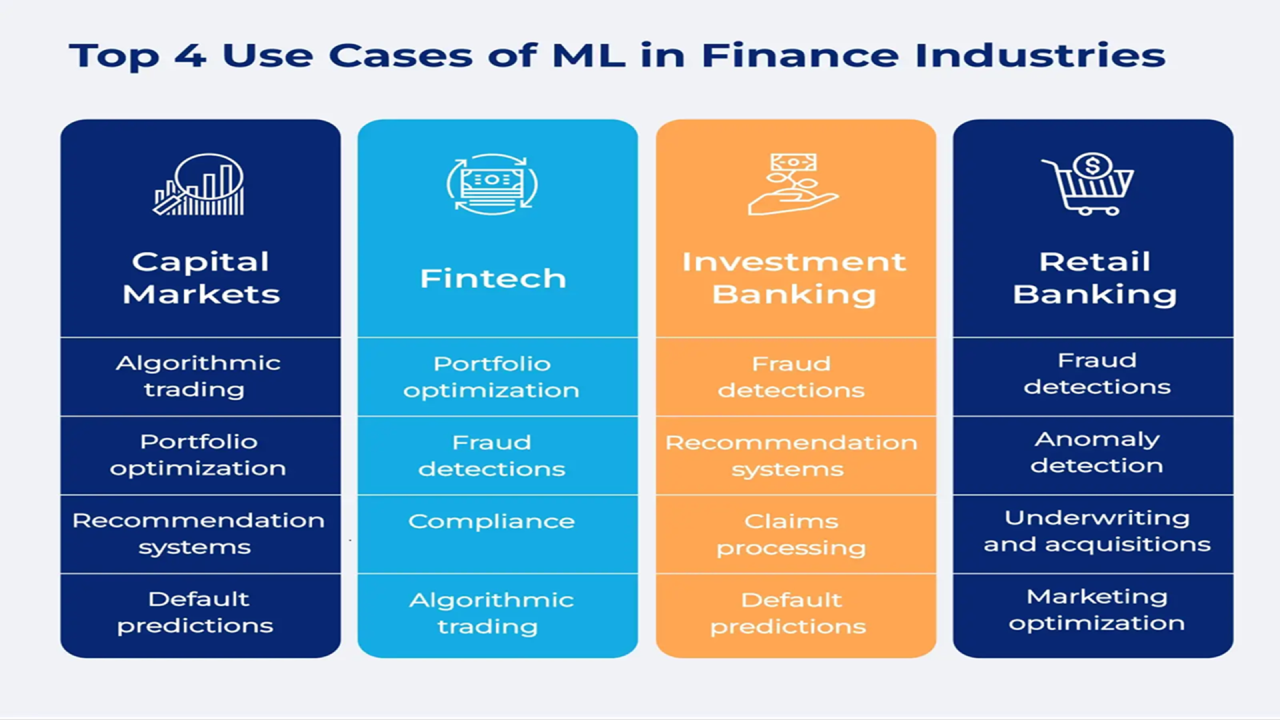
Core Data Analysis & Portfolio Optimization
AI-driven portfolio optimization combines deep data analysis with advanced computing techniques to build smarter strategies.
At its heart, this process transforms raw data into actionable strategies by using machine learning (ML) and natural language processing (NLP).
For instance, neural networks can predict asset returns by analyzing historical performance alongside real-time market signals, while NLP deciphers sentiment from earnings calls or geopolitical news.
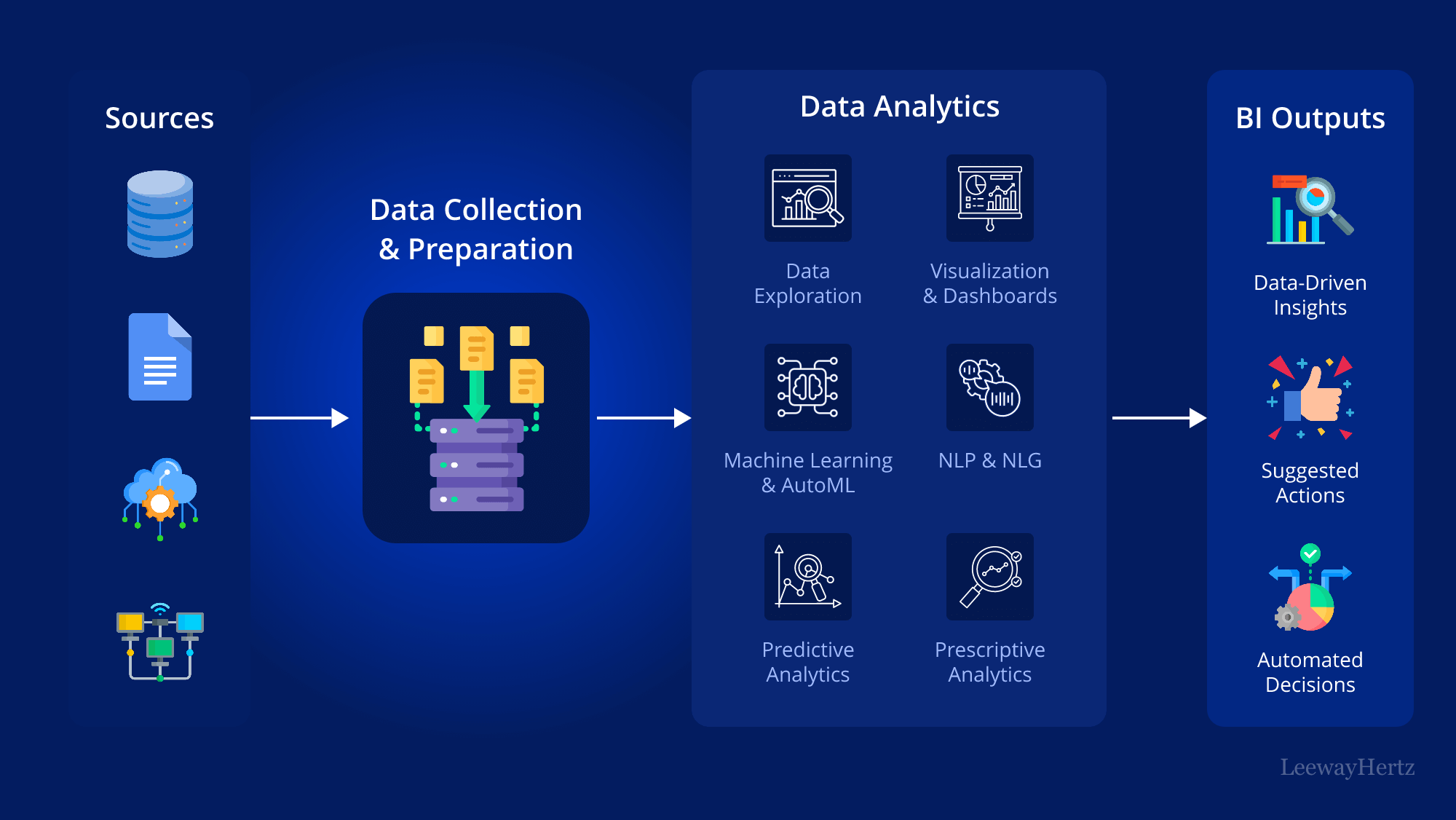
Quantitative Techniques
One of the most transformative quantitative techniques in portfolio optimization is Bayesian inference, which dynamically updates investment models as new data becomes available.
Unlike static approaches, Bayesian methods incorporate uncertainty, allowing portfolios to adapt to volatile market conditions. For example, during the COVID-19 pandemic, Bayesian models helped investors recalibrate risk exposure by integrating real-time data on supply chain disruptions and shifting consumer behavior.
Another particularly compelling application is the use of Monte Carlo simulations to stress-test portfolios.
By generating thousands of potential market scenarios, these simulations evaluate the resilience of investment strategies under extreme conditions, such as sudden interest rate hikes or geopolitical crises. This approach enhances risk management and uncovers hidden vulnerabilities in traditional optimization models.
Risk Management & Predictive Modeling
In risk management, AI retrieval systems redefine how predictive modeling is applied to safeguard investments.
Unlike traditional models that rely heavily on static datasets, AI retrieval dynamically integrates real-time data streams, such as geopolitical events or market sentiment, to predict risks more precisely.
Risk Mitigation Practices
One of the most transformative risk mitigation practices enabled by AI retrieval is dynamic scenario analysis.
Unlike static stress tests, which rely on predefined conditions, AI retrieval systems continuously ingest real-time data—such as regulatory changes or supply chain disruptions—and simulate their cascading effects across portfolios.
A crucial but often overlooked tool is adaptive risk thresholds, which adjust automatically to market changes.
Traditional models often apply fixed risk parameters, but AI retrieval systems dynamically adjust these thresholds based on evolving market conditions. This adaptability ensures that portfolios remain resilient despite extreme volatility, such as sudden interest rate hikes or geopolitical crises.
Moreover, integrating knowledge graphs into risk frameworks uncovers hidden interdependencies, such as how energy price fluctuations ripple through manufacturing sectors. This challenges the conventional wisdom of siloed risk assessments, offering a more interconnected perspective.
Forecasting & Predictive Analytics
Causal inference models help predictive analytics go beyond correlations to understand the real drivers behind market changes. Unlike traditional time series models that rely on correlations, causal inference identifies the underlying drivers of market behavior, enabling more robust predictions.
A particularly compelling application is the use of ensemble learning in predictive analytics. Ensemble models, which blend different AI techniques (such as gradient boosting), reduce biases and produce more reliable predictions.
Real-World Applications & Future Trends
AI retrieval systems are reshaping investment strategies by bridging the gap between raw data and actionable insights.
A striking example is JPMorgan Chase’s proprietary AI models, which analyze internal datasets to optimize decision-making. These systems have demonstrated the ability to identify market anomalies, such as early indicators of liquidity risks, enabling preemptive action.
Looking forward, the fusion of retrieval with quantum-inspired algorithms could revolutionize high-frequency trading, solving complex optimization problems at unprecedented speeds.
This evolution promises a future where investment strategies are smarter and more adaptive to uncertainty.
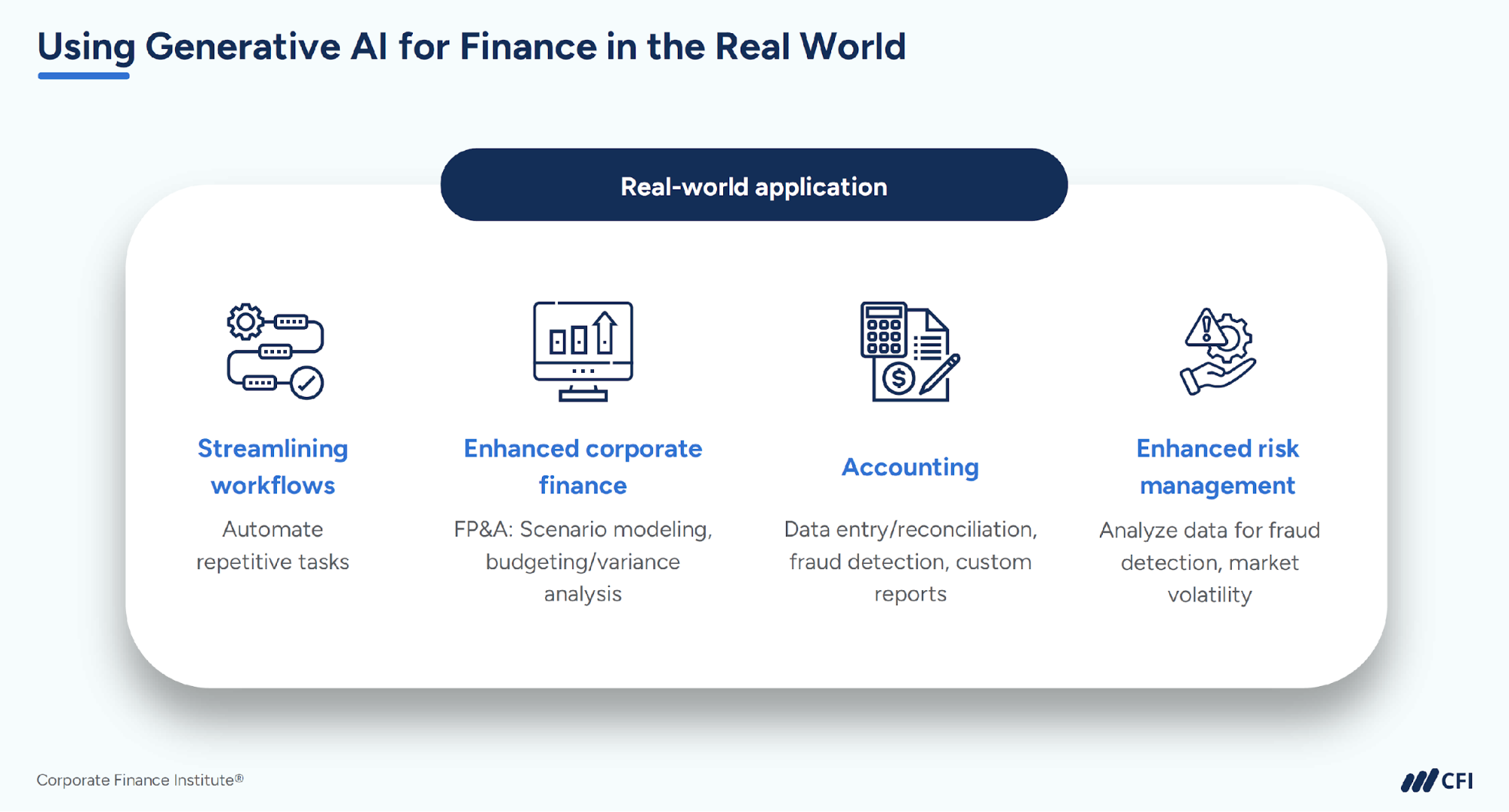
FAQ
What is AI retrieval, and how does it differ from predictive analytics in investment strategies?
AI retrieval extracts relevant real-time data for immediate insights, while predictive analytics forecasts trends using historical patterns. Together, they enhance intelligent investment strategies.
How do AI retrieval systems enhance real-time decision-making for portfolio management?
AI retrieval systems provide timely insights by extracting real-time data, enabling adaptive strategies like automatic rebalancing and risk mitigation during market shifts.
What role do knowledge graphs play in uncovering hidden relationships within financial data?
Knowledge graphs map connections between entities like policies and market trends, revealing insights that help investors identify risks and opportunities missed by traditional analysis.
How can AI retrieval systems mitigate risks associated with unstructured and fragmented datasets?
AI retrieval integrates unstructured data using anomaly detection and semantic search, ensuring data quality and enabling reliable decisions even in volatile markets.
What are the ethical and regulatory considerations when implementing AI retrieval in investment strategies?
Key considerations include data privacy, algorithmic bias prevention, and regulatory compliance. Explainable AI and governance practices ensure ethical and transparent use of AI in finance.
Conclusion
AI retrieval systems are redefining how investment strategies are built. Unlike traditional models, these systems excel at understanding real-time market shifts, identifying hidden patterns, and adapting to uncertainty with unmatched speed.
As the financial industry evolves, those who can utilize the power of AI retrieval will gain a significant edge—building portfolios that are not only data-driven but also context-aware, resilient, and future-proof.